Not so long ago, Sberbank, and then Yandex, announced the creation of super-large Russian language models similar to GPT-3. They not only generate believable text (articles, songs, blogs, etc.), but also solve many different problems, and these problems can often be set in Russian without programming and additional training - something very close to "universal" artificial intelligence ... But, as the authors of Sberbank write in their blog, “ such experiments are available only to companies with significant computing resources.". Training models with billions of parameters costs several tens, or even hundreds of millions of rubles. It turns out that individual developers and small companies are now excluded from the process and can now only use models trained by someone. In this article, I'll try to challenge this thesis by talking about the results of trying to train a model with 30 billion parameters on two RTX 2080Ti cards.
How this article is structured, which parts you can skip
In the first part, I will briefly describe what the language model is and what it gives. If you know this, you can skip the first part without prejudice.
The second part explains the problem with training large models and discusses the possibilities for solving it.
Part 3 talks about the results of trying to train a model with 30 billion parameters on two RTX 2080Ti cards
In conclusion, we discuss why you need to bother learning language models on your own. This part is somewhat subjective and expresses my personal opinion, if you are not interested in the subjective opinion, then you can skip it.
In general, I tried to make the article understandable for a wide range of developers and self-sufficient, so I included a small background to the question and an explanation of important terms for understanding, as a result of which the article became a little bloated. Experts can replace that some things are explained not quite accurately and not completely, but here I tried to prefer an intuitive presentation to a mathematically rigorous one.
With this preface, let's get down to the main presentation.
What are language models and why it matters
, ( , ). , , . .
. - , . :
, . , :
-
, .
, — , «» . , , :
– , , . , , , , .
, . , , , « . ? :» . « :» , . , .
, Google Brain [1] OpenAI [2,3]
, , , , , , .
? , . — . — .
, :
1. *
|
|
GPU/ |
GPU |
, |
RuGPT3-small |
117 |
5376 |
32 |
940 |
RuGPT3-medium |
345 |
32256 |
64 |
5.6 |
RuGPT3-large |
762 |
43008 |
128 |
7.5 |
RuGPT3-XL |
1.3 |
61440 |
256 |
10.7 |
RuGPT3-12B |
12 |
609484.8* |
? |
106 |
YaLM-10B |
13 |
626688.0 |
? |
109 |
* . ,
** , OpenAI
GPT-
«» GPT-3 175 .
, , . , - , . , .
, . 100 — , . , , , "" .
:
, «» - , - , . , , , , .
. , , Portia , . Portia — , . . , , . Portia , , , [4]
, , — , , .
, , Mixture of Experts (MoE). [5]. , , «» . . , ( 1).
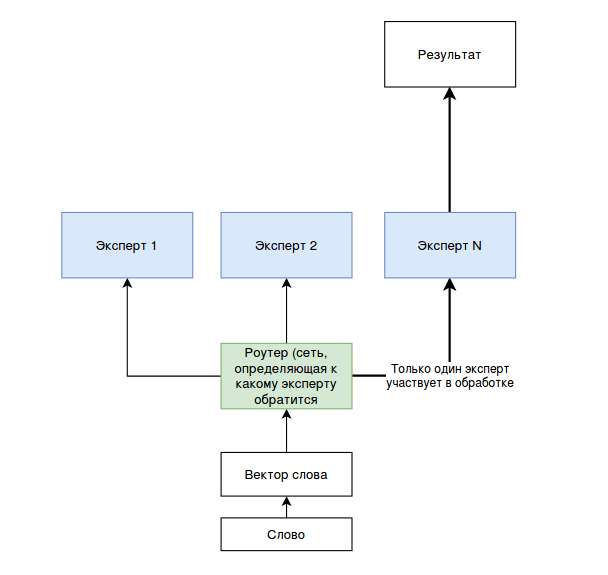
2020 Google Brain , , . Google Switch Transformer [6].
Switch Transformer GPT-2/3 , . ( Masked Language Objective). , .
, Switch Transformer 2.5-6 , ( — . dense) , .
-, - . Switch Transformer c 390 SuperGlue , 13 (SuperGlue 10 ).
( 2, ), , , Switch . , Switch , «». , ( « ?»), Switch .
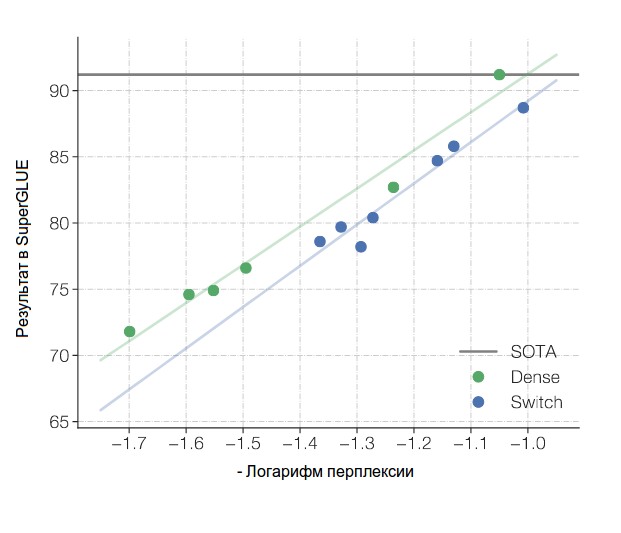
, - ( — ).
, Switch ( ), GPU, , (. . ). .
, , . ?
, (. . , ). [7, 8], . — ( ), .
20GB Common Crawl, - (7GB) (5GB). RTX 2080Ti ( 70000 ).
, GM30B 30 ( 160 GB ). (32 GB ), , .
«» , . , 100, 100
2.
|
( - ) |
RuGPT3-small |
30.91 |
RuGPT3-medium |
22.96 |
RuGPT3-large |
21.34 |
GM30B |
18.02 |
, , 1. , , , . , , .
, — - . , . , - ( , , ).
, , . , . ( YaLM, «», ).
: . ,
:
ruGPT3-medium: , .
ruGPT3-large: , , , . , , , "" !
ruGPT3-XL: , . ,
YALM ( «»): , . , , , .
GT30B: , , . , "", , (2), .
« » ruGPT3-large — - .
: , . , . , *
:
:
ruGPT3-medium: , . , , .
ruGPT3-large: , — , , , , . , , .
ruGPT3-XL: : " ". . .
YAML (c «»):— ! — , . — ! —
GM30B: , . , , , , : - - , , .
* . « ». GM30B , .
, , . . 400Kb (400Kb , « » ).
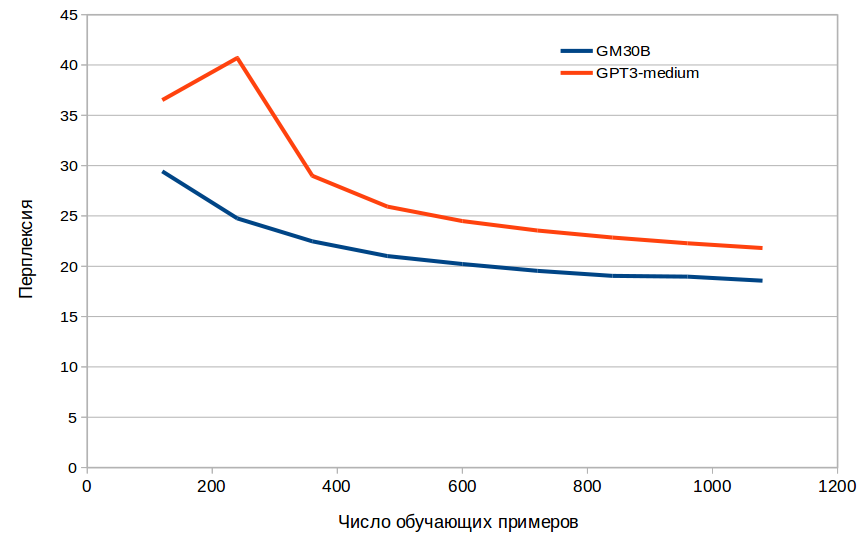
3 ruGPT3-medium. , .
. , (, ).
, . , , .
.
PARus «Russian SuperGlue». :
« PARus , , , . , 50%»
, « », : « -» « ». , .
3. PARus
|
|
RuGPT3-small |
0.562 |
RuGPT3-medium |
0.598 |
RuGPT3-large |
0.584 |
RuGPT3-XL |
0.676 |
GM30B |
0.704 |
YaLM 1B |
0.766 |
Leaderboard «Russian SuperGlue». . GM30B zero-shot learning, , , . .
, , GM30B , RuGPT3-XL.
Russian SuperGlue c PARus

? , , , ruGPT3/YaLM , . .
?
, . ( GitHub Copilot), , , , , - . , , . , , , -.
. GPT3 OpenAI , , «» . «» .
, OpenAI
GPT-3 , , , .. , . , , . " - ". , - . , , .
, «» , , . , Microsoft OpenAI 1 , GPT3 ( OpenAI). Microsoft , , GitHub Copilot, GPT3. , , , source.dev, API , , API , .
( ). . OpenAI GPT-2, , . , talktotransformer InferKit. InferKit , . , API GPT-3 OpenAI InferKit , .
, OpenAI ? talktotransformer, InferKit . .. .
, , , , — GPU , ruGPT3-XL .
, , - . — , ( ) — .
, IT, . - «» - . , , .
— . , , GPT-3 . , , , . , - ( ruGPT3 YaLM - Yet Anoter Language Model - , ).
, . , .
1. Trinh, Trieu H., and Quoc V. Le. "Do language models have common sense?." (2018). https://openreview.net/forum?id=rkgfWh0qKX
2. Radford, Alec, et al. "Language models are unsupervised multitask learners." OpenAI blog 1.8 (2019): 9.
3. Brown, T. B., Mann, B., Ryder, N., Subbiah, M., Kaplan, J., Dhariwal, P., ... & Amodei, D. (2020). Language models are few-shot learners. arXiv preprint arXiv:2005.14165.
4. Prete, Frederick R., ed. Complex worlds from simpler nervous systems. MIT press, 2004.
5. Noam Shazeer, Azalia Mirhoseini, Krzysztof Maziarz, Andy Davis, Quoc Le, Geoffrey Hinton,and Jeff Dean. Outrageously large neural networks: The sparsely-gated mixture-of-experts layer.arXiv preprint arXiv:1701.06538, 2017
6. Fedus, William, Barret Zoph, and Noam Shazeer. "Switch Transformers: Scaling to Trillion Parameter Models with Simple and Efficient Sparsity." arXiv preprint arXiv:2101.03961 (2021).
7. Liang, Yuchen, et al. "Can a Fruit Fly Learn Word Embeddings?." arXiv preprint arXiv:2101.06887 (2021).
8. Ryali, Chaitanya, et al. "Bio-inspired hashing for unsupervised similarity search." International Conference on Machine Learning . PMLR, 2020 and