
Leading scientific conferences ask for reproducibility of experiments. And this is necessary to increase the credibility of the work, to extract value (reusability and citation), well, and the "trend" ( according to a survey of the journal Nature ).
Expectations are growing, in 2021 already 9 out of 10 conferences offer authors to be checked for reproducibility. Pass the test, fill out a questionnaire, bring a witness, etc.
What we are talking about, why reproducibility is needed, what problems need to be solved, we will discuss in this article.
Experiments in machine learning
, AAAI 2014, AAAI 2016, IJCAI 2013 IJCAI 2016 , 80% โ !
,
2021 . GuideToResearch (Top 100), Machine Learning, Data Mining & Artificial Intelligence. .
# |
|
|
|
1 |
CVPR 2020 |
http://cvpr2020.thecvf.com/submission/main-conference/author-guidelines |
Encouraged |
2 |
NeurIPS 2021 |
https://neurips.cc/Conferences/2021/PaperInformation/PaperChecklist |
Required |
3 |
ICCV 2021 |
http://iccv2021.thecvf.com/node/4 |
Encouraged |
4 |
ECCV 2020 |
https://eccv2020.eu/reviewer-instructions/ |
Encouraged |
5 |
AAAI 2021 |
https://aaai.org/Conferences/AAAI-21/aaai21call/ |
Required |
6 |
ICML 2021 |
https://icml.cc/Conferences/2021/CallForPapers |
Encouraged |
7 |
SIGKDD 2021 |
https://www.kdd.org/kdd2020/files/KDD_2020_Call_for_Research_Papers.pdf |
Encouraged |
8 |
IJCAI 2021 |
|
Required |
9 |
ICLR 2021 |
https://iclr.cc/Conferences/2021/CallForPapers |
Not found |
10 |
ACL 2021 |
https://2021.aclweb.org/calls/papers/ |
Reminder |
:
Not found โ CFP .
Reminder โ .
Encouraged โ , .
Required โ .
?
, . , , , . , , . ( NeurIPS, Gundersen et al.).
, , .
, . , 2020-2021 , , . ACM , :
(repeatable experiment / )
, .
(artifact) , , , , , .
(reproducible / )
, .
(replicable / )
.
, ยซ ยป, .
.
, - https://en.wikipedia.org/wiki/Reproducibility
โnon-reproducible single occurrences are of no significance to scienceโ
โ Popper, K. R. 1959. The logic of scientific discovery. Hutchinson, London, United Kingdom.
. .
โ
.
: , ? -, , (, ). , ?
: , , . , ? ? , , !
, ?
. , arxiv.org paperswithcode.org. ? ? ? ? . ยซ ยป, โ .
โ provenance
. ! Prov-ML . UML 2 ( , << ยซ).
. ? ? ? ? ?
โ ?!
. , , , .
โ , , code style, , , .
โ //, / ( ), . . , (. ).
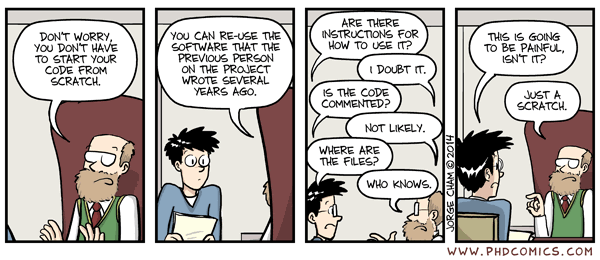
, ยซ ยป StackOverflow StackExchange. ยซ , . โ , . , , , , , , .ยป
, , -, .
,
Jupyter Notebooks, GitHub, , 4% .
, , :

A Large-scale Study about Quality and Reproducibility of Jupyter Notebooks.
โ . requirements.txt, setup.py. - .
โ . , .
โ , , .
(40%)
(13%)
( matplotlib ) (52%)
(3%)
(3%)
. python (4%)
(27%)
? , R 44% ( , ). , .
?
, ยซ ยป (. ยซ DLยป , google it).
. .
โ . (a.k.a. MLOps), (Exploratory Data Analysis, EDA) , , - .
P.S. FAIR
, (Findable), (Accessible), (Interoperable) (Reusable) (FAIR) 2016 , .
[7] , Best Practices for Scientific Computing.
[8] Top Ten Reasons (not) to Share your Research Code .
[9] An article with survey results that has a greater impact on reproducibility, Understanding experiments and research practices for reproducibility: an exploratory study