By issuing prompts and predicting events, artificial intelligence forms recommendation systems for the rotation of goods and services that are adequate to user requests, focusing on their preferences. According to the idea, this process should occur almost instantly: the distribution of products by category, the automatic creation of a pool of related products based on the associative row and manipulation of the big date. But in reality, artificial intelligence does not keep up with changing user preferences, and often simply makes mistakes when ranking products based on previous requests. As a result, marketplaces offer adult men sets of clothes for dolls, which, once using his card, were ordered by a schoolgirl daughter, and children are given “as a gift” new brands of machine oil and subscriptions to business seminars.
Hitting the client
Often, systems generate either erroneous or irrelevant recommendations, and in large numbers, using an algorithm that does not resist cheating and hacking. Thus, online stores are faced with the problem of poor efficiency of the generated recommendations.
To prevent this from happening, the creators of neural networks need to solve the problem of accurately identifying trends for changes and user preferences. That is, recommendation engines must learn to predict not only the reaction to the offer of a product or service, but also offer similar or alternative options (depending on the user's reaction).
In e-commerce, separately functioning collaborative, content and expert recommender systems can fail, and a hybrid must be created. A flexible hybrid recommendation algorithm will combine data from multiple channels over time. Weighted, augmented, mixed and random techniques can be used in them at the same time.
The collaborative filtering algorithm looks like this. Given a preference matrix and the ability to determine similarity using a cosine measure, you will need to select the number of users with similar tastes. Calculate the cosine measure for each user, multiply their ratings by the resulting measure, and calculate the sum of the calibrated ratings for each product. The algorithm formula looks like this.

The sim function is a measure of the similarity of two users.
U — .
r — .
k — , :
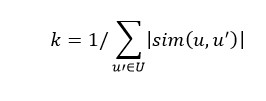
rec = makeRecommendation (‘ , (), , ).
, , , , . – . , «» , . , – . , , .
, . 5%, 12%. , , . , , . , , , .
, . , . , , , .
, , . , . , . json.
, , , «» . .
, . «» , - , .
, , , - , , , . , , – - . , , .