Introduction
My main job is in mobile advertising, and from time to time I have to work with data about mobile applications. I decided to make some of the data publicly available for those who want to practice building models or get an idea of the data that can be collected from open sources. I believe that open datasets are always useful to the community. Collecting data is often difficult and dull work, and not everyone has the opportunity to do it. In this article, I will introduce a dataset and use it to build one model.
Data
The dataset is published on the Kaggle website .
DOI: 10.34740/KAGGLE/DSV/2107675.
For 293,392 applications (the most popular), description tokens and application data themselves, including the original description, are collected. There are no application names in the dataset; they are identified by unique identifiers. Before tokenization, most of the descriptions were translated into English.
There are 4 files in the dataset:
bundles_desc.csv - contains only descriptions;
bundles_desc_tokens.csv - contains tokens and genres;
bundles_prop.csv, bundles_summary.csv - contain various application characteristics and release / update dates.
EDA
First of all, let's take a look at how data is distributed across operating systems.

Android apps dominate data. This is most likely due to the fact that more Android apps are being created.
, , , .
histnorm ='probability' # type of normalization

, .

2021 .

- .
df['bundle_update_period'] = \
(pd.to_datetime(
df['bundle_updated_at'], utc=True).dt.tz_convert(None).dt.to_period('M').astype('int') -
df['bundle_released_at'].dt.to_period('M').astype('int'))

, . , .

, . . . ? - Android , iOS Games. , , . . , .
, .
def get_lengths(df, columns=['tokens', 'description']):
lengths_df = pd.DataFrame()
for i, c in enumerate(columns):
lengths_df[f"{c}_len"] = df[c].apply(len)
if i > 0:
lengths_df[f"{c}_div"] = \
lengths_df.iloc[:, i-1] / lengths_df.iloc[:, i]
lengths_df[f"{c}_diff"] = \
lengths_df.iloc[:, i-1] - lengths_df.iloc[:, i]
return lengths_df
df = pd.concat([df, get_lengths(df)], axis=1, sort=False, copy=False)

, . , - .

Android-.
android_df = df[df['store_os']=='android']
ios_df = df[df['store_os']=='ios']
:
columns = [
'genre', 'tokens', 'bundle_update_period', 'tokens_len',
'description_len', 'description_div', 'description_diff',
'description', 'rating', 'reviews', 'score',
'released_at_month'
]
- train validation. , .
train_df, test_df = train_test_split(
android_df[columns], train_size=0.7, random_state=0, stratify=android_df['genre'])
y_train, X_train = train_df['genre'], train_df.drop(['genre'], axis=1)
y_test, X_test = test_df['genre'], test_df.drop(['genre'], axis=1)
CatBoost. CatBoost - . , CatBoost . 0.19.1
: BERT vs CatBoost , CatBoost BERT.
!pip install -U catboost
CatBoost Pool. , , . , .
train_pool = Pool(
data=X_train,
label=y_train,
text_features=['tokens', 'description']
)
test_pool = Pool(
data=X_test,
label=y_test,
text_features=['tokens', 'description']
)
. ; .
def fit_model(train_pool, test_pool, **kwargs):
model = CatBoostClassifier(
random_seed=0,
task_type='GPU',
iterations=10000,
learning_rate=0.1,
eval_metric='Accuracy',
od_type='Iter',
od_wait=500,
**kwargs
)
return model.fit(
train_pool,
eval_set=test_pool,
verbose=1000,
plot=True,
use_best_model=True
)
. CatBoost, .
CatBoostClassifier :
tokenizers — ;
dictionaries — ;
feature_calcers — ;
text_processing — JSON- , , , .
, , , , .
tpo = {
'tokenizers': [
{
'tokenizer_id': 'Sense',
'separator_type': 'BySense',
}
],
'dictionaries': [
{
'dictionary_id': 'Word',
'token_level_type': 'Word',
'occurrence_lower_bound': '10'
},
{
'dictionary_id': 'Bigram',
'token_level_type': 'Word',
'gram_order': '2',
'occurrence_lower_bound': '10'
},
{
'dictionary_id': 'Trigram',
'token_level_type': 'Word',
'gram_order': '3',
'occurrence_lower_bound': '10'
},
],
'feature_processing': {
'0': [
{
'tokenizers_names': ['Sense'],
'dictionaries_names': ['Word'],
'feature_calcers': ['BoW']
},
{
'tokenizers_names': ['Sense'],
'dictionaries_names': ['Bigram', 'Trigram'],
'feature_calcers': ['BoW']
},
],
'1': [
{
'tokenizers_names': ['Sense'],
'dictionaries_names': ['Word'],
'feature_calcers': ['BoW', 'BM25']
},
{
'tokenizers_names': ['Sense'],
'dictionaries_names': ['Bigram', 'Trigram'],
'feature_calcers': ['BoW']
},
]
}
}
:
model_catboost = fit_model( train_pool, test_pool, text_processing = tpo )
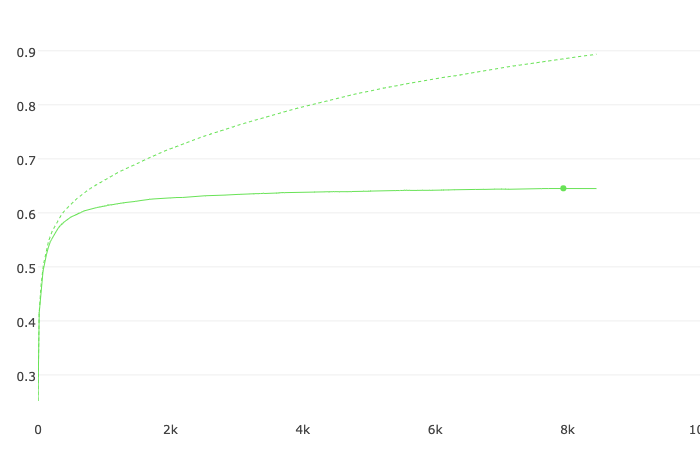
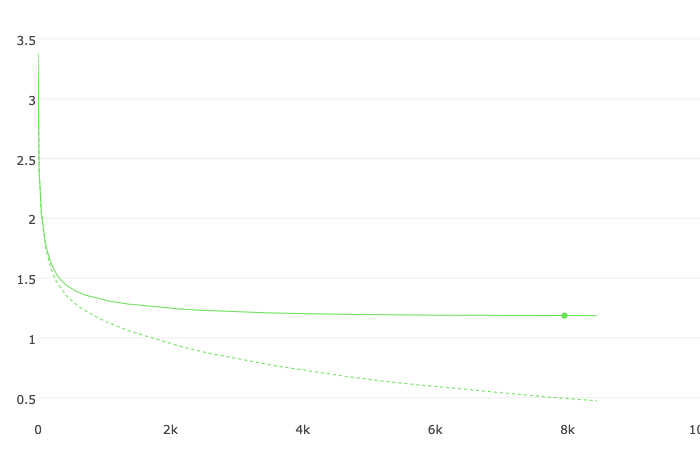
bestTest = 0.6454657601

. , summary, , iOS, . , . .
, . , , . , . , .
, , OOF (Out-of-Fold). ; .
def get_oof(n_folds, x_train, y, x_test, text_features, seeds):
ntrain = x_train.shape[0]
ntest = x_test.shape[0]
oof_train = np.zeros((len(seeds), ntrain, 48))
oof_test = np.zeros((ntest, 48))
oof_test_skf = np.empty((len(seeds), n_folds, ntest, 48))
test_pool = Pool(data=x_test, text_features=text_features)
models = {}
for iseed, seed in enumerate(seeds):
kf = StratifiedKFold(
n_splits=n_folds,
shuffle=True,
random_state=seed)
for i, (tr_i, t_i) in enumerate(kf.split(x_train, y)):
print(f'\nSeed {seed}, Fold {i}')
x_tr = x_train.iloc[tr_i, :]
y_tr = y[tr_i]
x_te = x_train.iloc[t_i, :]
y_te = y[t_i]
train_pool = Pool(
data=x_tr, label=y_tr, text_features=text_features)
valid_pool = Pool(
data=x_te, label=y_te, text_features=text_features)
model = fit_model(
train_pool, valid_pool,
random_seed=seed,
text_processing = tpo
)
x_te_pool = Pool(
data=x_te, text_features=text_features)
oof_train[iseed, t_i, :] = \
model.predict_proba(x_te_pool)
oof_test_skf[iseed, i, :, :] = \
model.predict_proba(test_pool)
models[(seed, i)] = model
oof_test[:, :] = oof_test_skf.mean(axis=1).mean(axis=0)
oof_train = oof_train.mean(axis=0)
return oof_train, oof_test, models
, :
oof_train — OOF- Android
oof_test — OOF- iOS
models — all OOF-
from sklearn.metrics import accuracy_score
accuracy_score(
android_df['genre'].values,
np.take(models[(0,0)].classes_, oof_train.argmax(axis=1)))
.
OOF accuracy: 0.6560790777135628
android_genre_vec, oof_train Android oof_test iOS.
idx = df[df['store_os']=='ios'].index
df.loc[df['store_os']=='ios', 'android_genre_vec'] = \
pd.Series(list(oof_test), index=idx)
idx = df[df['store_os']=='android'].index
df.loc[df['store_os']=='android', 'android_genre_vec'] = \
pd.Series(list(oof_train), index=idx)
android_genre, .
df.loc[df['store_os']=='ios', 'android_genre'] = \
np.take(models[(0,0)].classes_, oof_test.argmax(axis=1))
df.loc[df['store_os']=='android', 'android_genre'] = \
np.take(models[(0,0)].classes_, oof_train.argmax(axis=1))
After all the manipulations, you can finally see and compare the distribution of applications by genre.

Outcomes
In the article:
new free dataset introduced;
made a small EDA;
several new features have been created;
a model has been created to predict the genres of applications from descriptions.
I hope this dataset will be useful to the community and will be used both in models and for further study. As far as possible, I will try to update it.
The code from the article can be viewed here .