Hello everyone! My name is Vladislav Mosin, and I am a 4th year student of the Bachelor's program in Applied Mathematics and Informatics at the St. Petersburg HSE. Last summer, together with Alina Pleshkova, a graduate student of our faculty, I did an internship at JetBrains Research. We worked on the Music2Dance project, the goal of which is to learn how to generate dance moves that fit the given music. This can be used, for example, in self-study of dancing: I heard the music, launched the application, and it showed movements that are harmoniously combined with this music.
Looking ahead, I will say that our results, unfortunately, turned out to be far from the best models of motion generation that exist now. But if you are also interested in sorting out this problem, I invite you under cat.
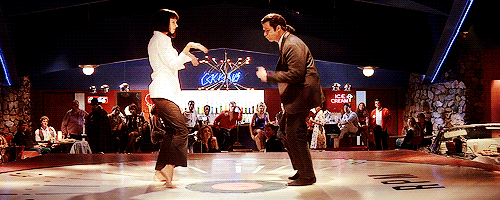
Existing approaches
The idea of ββgenerating dance from music is pretty old. Probably the most striking example is dance simulators such as Dance Dance Revolution, where the player must step on the panels on the floor that are glowing in time with the music, and thus a kind of dance is created. Also a beautiful result in this area is the creation of dancing geometric shapes or 2D men.
There is also more serious work - the generation of 3D movements for people. Most of these approaches are based solely on deep learning. The best results for the summer of 2020 were shown by the DanceNet architecture , and we decided to take it as a baseline. We will discuss their approach in more detail below.
Data preprocessing
: , , . .
: onset, beats, chroma
, β β . , , , . , onset, beats chroma ( , ).
:
. β β . , , , -. , (, 240x240) .
, . , . , , , , .
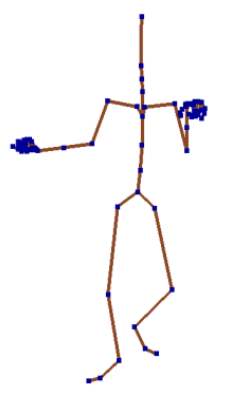
( ), .
. , 3D-, : , . , , . : , .
DanceNet

DanceNet coc :
;
;
;
;
.
:
. Bi-LSTM .
. , Bi-LSTM .
- . .
. , . dilated () -.
DanceNet , , β , , .
, , . , . , , , . . , .
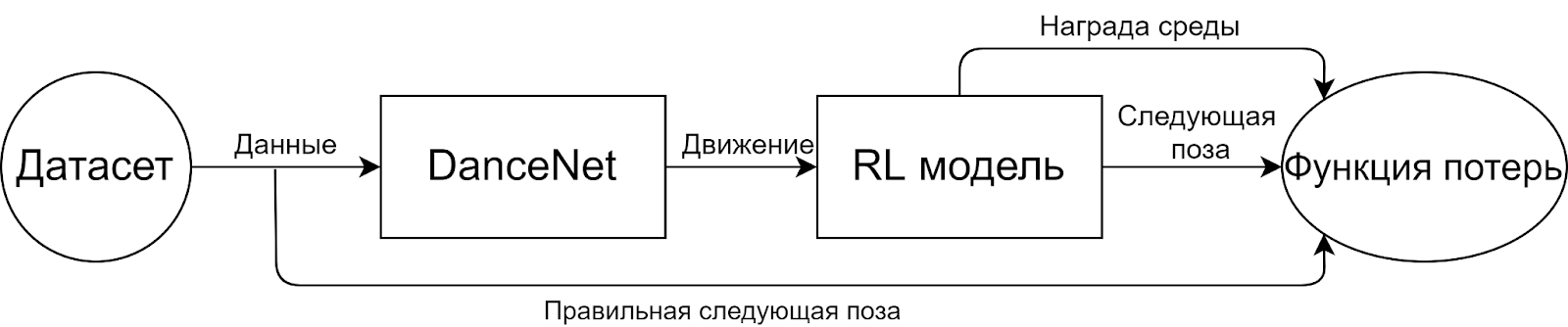
:
-
(DanceNet)
(RL )
. 2020 , . , , . , . , - . , : YouTube VIBE.
DanceNet
β , -, , -, .
RL
RL β , . ( ) , .
. : .

, Q-Learning ( TD3, ) ( PPO).
, . -, , . , , , . Humanoid, , .
, , .
S β , Sreal β , R β .

, , , . , . .
, , DanceNet. , , , , . . , β YouTube , RL .
. !
: