
Before the advent of YOLO, most methods of object detection tried to adapt classifiers for detection. In YOLO, object detection was formulated as a regression problem on spatially separated bounding boxes and associated class probabilities.
In this article, we will learn about the YOLO Object Detection system and how to implement such a system in Tensorflow 2.0.
About YOLO:
Our unified architecture is extremely fast. The base model YOLO processes images in real time at 45 frames per second. A smaller version of the network, Fast YOLO, processes as much as 155 frames per second ...
- You Only Look Once: Unified, Real-Time Object Detection, 2015
What is YOLO?

YOLO – ( ) () . (Joseph Redmon). YOLO . YOLO R-CNN (Region-Based Convolutional Neural Network) . .
( 2016 ) , R-CNN DPM (Deformable Part Model), YOLO mAP (mean Average Precision). , YOLO . .
( ): , (DPM). , R-CNN (region proposal method). bounding box’. , , bounding box’, , .
YOLO . bounding box’ . , bounding box’ .
YOLO , . , S x S
. .
-, bounding box’. , (confidence value) , bounding box’. , . , - , , .
, , .

-, . , - - , . , , , . , , .
.
YOLO anchor boxes ( / ) bounding box’. anchor box’ . , anchor box’ ( , anchor box’). COCO (Common Objects in Context) k- (K-means clustering).

, :
bounding box':
4 (tx , ty , tw , th)
1 objectness error ( ),
cx , cy :
pw () ph () bounding box'. , YOLOv2, .
. S x S x [B * (4+1+C)]
, B
– bounding box', , C
– , 4
– bounding box', 1
– objectness prediction ( ). , . , YOLOv3 bounding box' .
, , bounding box', .
. IoU (Intersection over Union / ). .

, “ -” (non-maximum suppression). - : bounding box , , bounding box' , IoU .
, , , . , , . , , .

YOLO:
You Only Look Once: Unified, Real-Time Object Detection
YOLO9000: Better, Faster, Stronger
YOLOv3: An Incremental Improvement
Tensorflow
, (fully convolutional network / FCN) . , , . YOLOv3 COCO (Common Objects in Context). .
# checkpoint' .
# !mkdir checkpoints
# YOLOv3 .
# !wget https://pjreddie.com/media/files/yolov3.weights
# .
import cv2
import numpy as np
import tensorflow as tf
from absl import logging
from itertools import repeat
from PIL import Image
from tensorflow.keras import Model
from tensorflow.keras.layers import Add, Concatenate, Lambda
from tensorflow.keras.layers import Conv2D, Input, LeakyReLU
from tensorflow.keras.layers import MaxPool2D, UpSampling2D, ZeroPadding2D
from tensorflow.keras.regularizers import l2
from tensorflow.keras.losses import binary_crossentropy
from tensorflow.keras.losses import sparse_categorical_crossentropy
yolo_iou_threshold = 0.6 # Intersection Over Union (iou) threshold.
yolo_score_threshold = 0.6 # Score threshold.
weightyolov3 = 'yolov3.weights' # .
size = 416 # .
checkpoints = 'checkpoints/yolov3.tf' # checkpoint'.
num_classes = 80 # .
# YOLOv3 Fully Convolutional Network (FCN).
YOLO_V3_LAYERS = [
'yolo_darknet',
'yolo_conv_0',
'yolo_output_0',
'yolo_conv_1',
'yolo_output_1',
'yolo_conv_2',
'yolo_output_2'
]
, Darknet (open source NN framework) tf.keras , API . , keras. TF Checkpoints Tensorflow.
# .
def load_darknet_weights(model, weights_file):
wf = open(weights_file, 'rb')
major, minor, revision, seen, _ = np.fromfile(wf, dtype=np.int32, count=5)
layers = YOLO_V3_LAYERS
for layer_name in layers:
sub_model = model.get_layer(layer_name)
for i, layer in enumerate(sub_model.layers):
if not layer.name.startswith('conv2d'):
continue
batch_norm = None
if i + 1 < len(sub_model.layers) and \
sub_model.layers[i + 1].name.startswith('batch_norm'):
batch_norm = sub_model.layers[i + 1]
logging.info("{}/{} {}".format(
sub_model.name, layer.name, 'bn' if batch_norm else 'bias'))
filters = layer.filters
size = layer.kernel_size[0]
in_dim = layer.input_shape[-1]
if batch_norm is None:
conv_bias = np.fromfile(wf, dtype=np.float32, count=filters)
else:
bn_weights = np.fromfile(wf, dtype=np.float32, count=4*filters)
bn_weights = bn_weights.reshape((4, filters))[[1, 0, 2, 3]]
conv_shape = (filters, in_dim, size, size)
conv_weights = np.fromfile(wf, dtype=np.float32, count=np.product(conv_shape))
conv_weights = conv_weights.reshape(conv_shape).transpose([2, 3, 1, 0])
if batch_norm is None:
layer.set_weights([conv_weights, conv_bias])
else:
layer.set_weights([conv_weights])
batch_norm.set_weights(bn_weights)
assert len(wf.read()) == 0, 'failed to read weights'
wf.close()
, IoU. batch normalization ( ) , . tf.keras.layers.BatchNormalization (transfer learning), .
# IoU.
def interval_overlap(interval_1, interval_2):
x1, x2 = interval_1
x3, x4 = interval_2
if x3 < x1:
return 0 if x4 < x1 else (min(x2,x4) - x1)
else:
return 0 if x2 < x3 else (min(x2,x4) - x3)
def intersectionOverUnion(box1, box2):
intersect_w = interval_overlap([box1.xmin, box1.xmax], [box2.xmin, box2.xmax])
intersect_h = interval_overlap([box1.ymin, box1.ymax], [box2.ymin, box2.ymax])
intersect_area = intersect_w * intersect_h
w1, h1 = box1.xmax-box1.xmin, box1.ymax-box1.ymin
w2, h2 = box2.xmax-box2.xmin, box2.ymax-box2.ymin
union_area = w1*h1 + w2*h2 - intersect_area
return float(intersect_area) / union_area
class BatchNormalization(tf.keras.layers.BatchNormalization):
def call(self, x, training=False):
if training is None: training = tf.constant(False)
training = tf.logical_and(training, self.trainable)
return super().call(x, training)
# 3 anchor box' .
yolo_anchors = np.array([(10, 13), (16, 30), (33, 23), (30, 61), (62, 45),
(59, 119), (116, 90), (156, 198), (373, 326)], np.float32) / 416
yolo_anchor_masks = np.array([[6, 7, 8], [3, 4, 5], [0, 1, 2]])
3 anchor box' . :
0, 1, 2 – ,
3, 4 ,5 – , ,
6, 7, 8 – , , ,
# bounding box'.
def draw_outputs(img, outputs, class_names, white_list=None):
boxes, score, classes, nums = outputs
boxes, score, classes, nums = boxes[0], score[0], classes[0], nums[0]
wh = np.flip(img.shape[0:2])
for i in range(nums):
if class_names[int(classes[i])] not in white_list:
continue
x1y1 = tuple((np.array(boxes[i][0:2]) * wh).astype(np.int32))
x2y2 = tuple((np.array(boxes[i][2:4]) * wh).astype(np.int32))
img = cv2.rectangle(img, x1y1, x2y2, (255, 0, 0), 2)
img = cv2.putText(img, '{} {:.4f}'.format(
class_names[int(classes[i])], score[i]),
x1y1, cv2.FONT_HERSHEY_COMPLEX_SMALL, 1, (0, 0, 255), 2)
return img
YOLOv3. , . 53, , , .
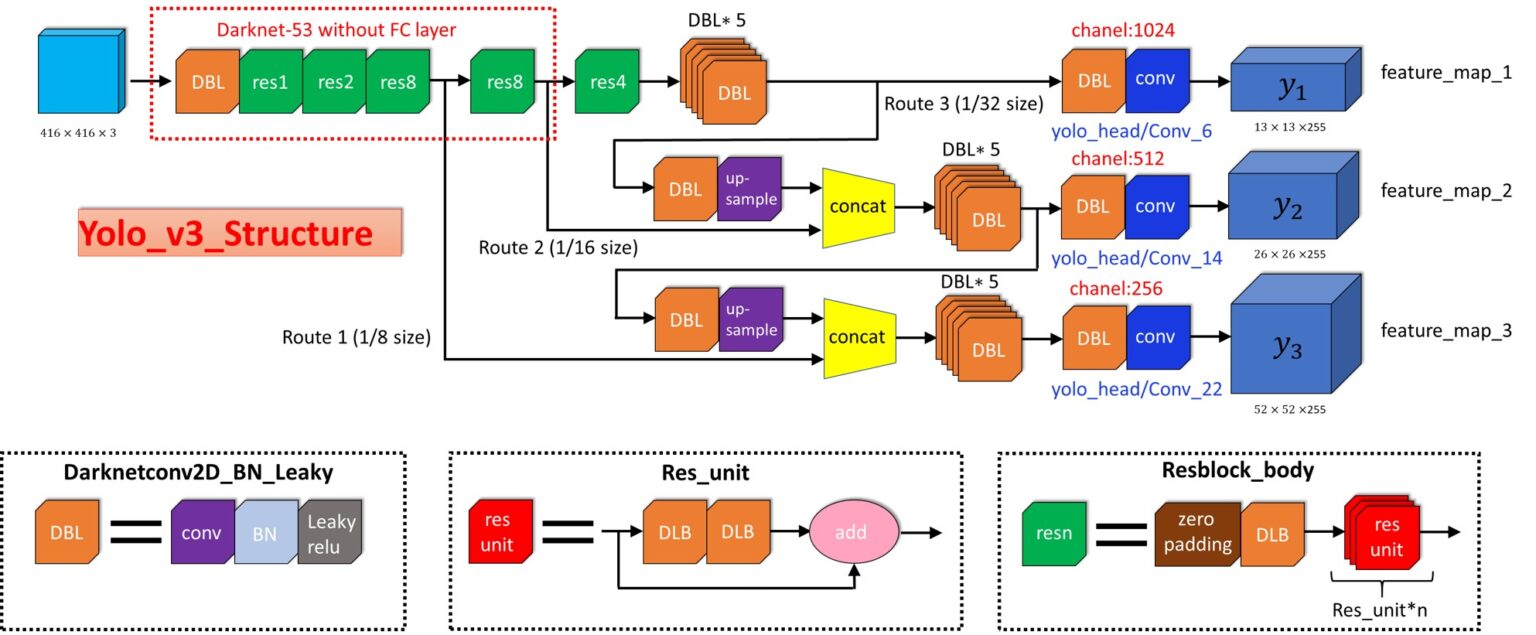

, API, . (ResNet Block) .
def DarknetConv(x, filters, size, strides=1, batch_norm=True):
if strides == 1:
padding = 'same'
else:
x = ZeroPadding2D(((1, 0), (1, 0)))(x)
padding = 'valid'
x = Conv2D(filters=filters, kernel_size=size,
strides=strides, padding=padding,
use_bias=not batch_norm, kernel_regularizer=l2(0.0005))(x)
if batch_norm:
x = BatchNormalization()(x)
x = LeakyReLU(alpha=0.1)(x)
return x
def DarknetResidual(x, filters):
previous = x
x = DarknetConv(x, filters // 2, 1)
x = DarknetConv(x, filters, 3)
x = Add()([previous , x])
return x
def DarknetBlock(x, filters, blocks):
x = DarknetConv(x, filters, 3, strides=2)
for _ in repeat(None, blocks):
x = DarknetResidual(x, filters)
return x
def Darknet(name=None):
x = inputs = Input([None, None, 3])
x = DarknetConv(x, 32, 3)
x = DarknetBlock(x, 64, 1)
x = DarknetBlock(x, 128, 2)
x = x_36 = DarknetBlock(x, 256, 8)
x = x_61 = DarknetBlock(x, 512, 8)
x = DarknetBlock(x, 1024, 4)
return tf.keras.Model(inputs, (x_36, x_61, x), name=name)
def YoloConv(filters, name=None):
def yolo_conv(x_in):
if isinstance(x_in, tuple):
inputs = Input(x_in[0].shape[1:]), Input(x_in[1].shape[1:])
x, x_skip = inputs
x = DarknetConv(x, filters, 1)
x = UpSampling2D(2)(x)
x = Concatenate()([x, x_skip])
else:
x = inputs = Input(x_in.shape[1:])
x = DarknetConv(x, filters, 1)
x = DarknetConv(x, filters * 2, 3)
x = DarknetConv(x, filters, 1)
x = DarknetConv(x, filters * 2, 3)
x = DarknetConv(x, filters, 1)
return Model(inputs, x, name=name)(x_in)
return yolo_conv
def YoloOutput(filters, anchors, classes, name=None):
def yolo_output(x_in):
x = inputs = Input(x_in.shape[1:])
x = DarknetConv(x, filters * 2, 3)
x = DarknetConv(x, anchors * (classes + 5), 1, batch_norm=False)
x = Lambda(lambda x: tf.reshape(x, (-1, tf.shape(x)[1], tf.shape(x)[2],
anchors, classes + 5)))(x)
return tf.keras.Model(inputs, x, name=name)(x_in)
return yolo_output
def yolo_boxes(pred, anchors, classes):
grid_size = tf.shape(pred)[1]
box_xy, box_wh, score, class_probs = tf.split(pred, (2, 2, 1, classes), axis=-1)
box_xy = tf.sigmoid(box_xy)
score = tf.sigmoid(score)
class_probs = tf.sigmoid(class_probs)
pred_box = tf.concat((box_xy, box_wh), axis=-1)
grid = tf.meshgrid(tf.range(grid_size), tf.range(grid_size))
grid = tf.expand_dims(tf.stack(grid, axis=-1), axis=2)
box_xy = (box_xy + tf.cast(grid, tf.float32)) / tf.cast(grid_size, tf.float32)
box_wh = tf.exp(box_wh) * anchors
box_x1y1 = box_xy - box_wh / 2
box_x2y2 = box_xy + box_wh / 2
bbox = tf.concat([box_x1y1, box_x2y2], axis=-1)
return bbox, score, class_probs, pred_box
-.
def nonMaximumSuppression(outputs, anchors, masks, classes):
boxes, conf, out_type = [], [], []
for output in outputs:
boxes.append(tf.reshape(output[0], (tf.shape(output[0])[0], -1, tf.shape(output[0])[-1])))
conf.append(tf.reshape(output[1], (tf.shape(output[1])[0], -1, tf.shape(output[1])[-1])))
out_type.append(tf.reshape(output[2], (tf.shape(output[2])[0], -1, tf.shape(output[2])[-1])))
bbox = tf.concat(boxes, axis=1)
confidence = tf.concat(conf, axis=1)
class_probs = tf.concat(out_type, axis=1)
scores = confidence * class_probs
boxes, scores, classes, valid_detections = tf.image.combined_non_max_suppression(
boxes=tf.reshape(bbox, (tf.shape(bbox)[0], -1, 1, 4)),
scores=tf.reshape(
scores, (tf.shape(scores)[0], -1, tf.shape(scores)[-1])),
max_output_size_per_class=100,
max_total_size=100,
iou_threshold=yolo_iou_threshold,
score_threshold=yolo_score_threshold)
return boxes, scores, classes, valid_detections
:
def YoloV3(size=None, channels=3, anchors=yolo_anchors,
masks=yolo_anchor_masks, classes=80, training=False):
x = inputs = Input([size, size, channels])
x_36, x_61, x = Darknet(name='yolo_darknet')(x)
x = YoloConv(512, name='yolo_conv_0')(x)
output_0 = YoloOutput(512, len(masks[0]), classes, name='yolo_output_0')(x)
x = YoloConv(256, name='yolo_conv_1')((x, x_61))
output_1 = YoloOutput(256, len(masks[1]), classes, name='yolo_output_1')(x)
x = YoloConv(128, name='yolo_conv_2')((x, x_36))
output_2 = YoloOutput(128, len(masks[2]), classes, name='yolo_output_2')(x)
if training:
return Model(inputs, (output_0, output_1, output_2), name='yolov3')
boxes_0 = Lambda(lambda x: yolo_boxes(x, anchors[masks[0]], classes),
name='yolo_boxes_0')(output_0)
boxes_1 = Lambda(lambda x: yolo_boxes(x, anchors[masks[1]], classes),
name='yolo_boxes_1')(output_1)
boxes_2 = Lambda(lambda x: yolo_boxes(x, anchors[masks[2]], classes),
name='yolo_boxes_2')(output_2)
outputs = Lambda(lambda x: nonMaximumSuppression(x, anchors, masks, classes),
name='nonMaximumSuppression')((boxes_0[:3], boxes_1[:3], boxes_2[:3]))
return Model(inputs, outputs, name='yolov3')
:
def YoloLoss(anchors, classes=80, ignore_thresh=0.5):
def yolo_loss(y_true, y_pred):
pred_box, pred_obj, pred_class, pred_xywh = yolo_boxes(
y_pred, anchors, classes)
pred_xy = pred_xywh[..., 0:2]
pred_wh = pred_xywh[..., 2:4]
true_box, true_obj, true_class_idx = tf.split(
y_true, (4, 1, 1), axis=-1)
true_xy = (true_box[..., 0:2] + true_box[..., 2:4]) / 2
true_wh = true_box[..., 2:4] - true_box[..., 0:2]
box_loss_scale = 2 - true_wh[..., 0] * true_wh[..., 1]
grid_size = tf.shape(y_true)[1]
grid = tf.meshgrid(tf.range(grid_size), tf.range(grid_size))
grid = tf.expand_dims(tf.stack(grid, axis=-1), axis=2)
true_xy = true_xy * tf.cast(grid_size, tf.float32) - \
tf.cast(grid, tf.float32)
true_wh = tf.math.log(true_wh / anchors)
true_wh = tf.where(tf.math.is_inf(true_wh),
tf.zeros_like(true_wh), true_wh)
obj_mask = tf.squeeze(true_obj, -1)
true_box_flat = tf.boolean_mask(true_box, tf.cast(obj_mask, tf.bool))
best_iou = tf.reduce_max(intersectionOverUnion(
pred_box, true_box_flat), axis=-1)
ignore_mask = tf.cast(best_iou < ignore_thresh, tf.float32)
xy_loss = obj_mask * box_loss_scale * \
tf.reduce_sum(tf.square(true_xy - pred_xy), axis=-1)
wh_loss = obj_mask * box_loss_scale * \
tf.reduce_sum(tf.square(true_wh - pred_wh), axis=-1)
obj_loss = binary_crossentropy(true_obj, pred_obj)
obj_loss = obj_mask * obj_loss + \
(1 - obj_mask) * ignore_mask * obj_loss
class_loss = obj_mask * sparse_categorical_crossentropy(
true_class_idx, pred_class)
xy_loss = tf.reduce_sum(xy_loss, axis=(1, 2, 3))
wh_loss = tf.reduce_sum(wh_loss, axis=(1, 2, 3))
obj_loss = tf.reduce_sum(obj_loss, axis=(1, 2, 3))
class_loss = tf.reduce_sum(class_loss, axis=(1, 2, 3))
return xy_loss + wh_loss + obj_loss + class_loss
return yolo_loss
" " :
(
[N, 13, 13, 3, 6],
[N, 26, 26, 3, 6],
[N, 52, 52, 3, 6]
)
N
– , 6
[x, y, w, h, obj, class]
bounding box'.
@tf.function
def transform_targets_for_output(y_true, grid_size, anchor_idxs, classes):
N = tf.shape(y_true)[0]
y_true_out = tf.zeros(
(N, grid_size, grid_size, tf.shape(anchor_idxs)[0], 6))
anchor_idxs = tf.cast(anchor_idxs, tf.int32)
indexes = tf.TensorArray(tf.int32, 1, dynamic_size=True)
updates = tf.TensorArray(tf.float32, 1, dynamic_size=True)
idx = 0
for i in tf.range(N):
for j in tf.range(tf.shape(y_true)[1]):
if tf.equal(y_true[i][j][2], 0):
continue
anchor_eq = tf.equal(
anchor_idxs, tf.cast(y_true[i][j][5], tf.int32))
if tf.reduce_any(anchor_eq):
box = y_true[i][j][0:4]
box_xy = (y_true[i][j][0:2] + y_true[i][j][2:4]) / 2
anchor_idx = tf.cast(tf.where(anchor_eq), tf.int32)
grid_xy = tf.cast(box_xy // (1/grid_size), tf.int32)
indexes = indexes.write(
idx, [i, grid_xy[1], grid_xy[0], anchor_idx[0][0]])
updates = updates.write(
idx, [box[0], box[1], box[2], box[3], 1, y_true[i][j][4]])
idx += 1
return tf.tensor_scatter_nd_update(
y_true_out, indexes.stack(), updates.stack())
def transform_targets(y_train, anchors, anchor_masks, classes):
outputs = []
grid_size = 13
anchors = tf.cast(anchors, tf.float32)
anchor_area = anchors[..., 0] * anchors[..., 1]
box_wh = y_train[..., 2:4] - y_train[..., 0:2]
box_wh = tf.tile(tf.expand_dims(box_wh, -2),
(1, 1, tf.shape(anchors)[0], 1))
box_area = box_wh[..., 0] * box_wh[..., 1]
intersection = tf.minimum(box_wh[..., 0], anchors[..., 0]) * \
tf.minimum(box_wh[..., 1], anchors[..., 1])
iou = intersection / (box_area + anchor_area - intersection)
anchor_idx = tf.cast(tf.argmax(iou, axis=-1), tf.float32)
anchor_idx = tf.expand_dims(anchor_idx, axis=-1)
y_train = tf.concat([y_train, anchor_idx], axis=-1)
for anchor_idxs in anchor_masks:
outputs.append(transform_targets_for_output(
y_train, grid_size, anchor_idxs, classes))
grid_size *= 2
return tuple(outputs) # [x, y, w, h, obj, class]
def preprocess_image(x_train, size):
return (tf.image.resize(x_train, (size, size))) / 255
, . COCO 80.
yolo = YoloV3(classes=num_classes)
load_darknet_weights(yolo, weightyolov3)
yolo.save_weights(checkpoints)
class_names = ["person", "bicycle", "car", "motorbike", "aeroplane", "bus", "train", "truck",
"boat", "traffic light", "fire hydrant", "stop sign", "parking meter", "bench",
"bird", "cat", "dog", "horse", "sheep", "cow", "elephant", "bear", "zebra", "giraffe",
"backpack", "umbrella", "handbag", "tie", "suitcase", "frisbee", "skis", "snowboard",
"sports ball", "kite", "baseball bat", "baseball glove", "skateboard", "surfboard",
"tennis racket", "bottle", "wine glass", "cup", "fork", "knife", "spoon", "bowl",
"banana","apple", "sandwich", "orange", "broccoli", "carrot", "hot dog", "pizza", "donut",
"cake","chair", "sofa", "pottedplant", "bed", "diningtable", "toilet", "tvmonitor", "laptop",
"mouse","remote", "keyboard", "cell phone", "microwave", "oven", "toaster", "sink",
"refrigerator","book", "clock", "vase", "scissors", "teddy bear", "hair drier", "toothbrush"]
def detect_objects(img_path, white_list=None):
image = img_path # .
img = tf.image.decode_image(open(image, 'rb').read(), channels=3)
img = tf.expand_dims(img, 0)
img = preprocess_image(img, size)
boxes, scores, classes, nums = yolo(img)
img = cv2.imread(image)
img = draw_outputs(img, (boxes, scores, classes, nums), class_names, white_list)
cv2.imwrite('detected_{:}'.format(img_path), img)
detected = Image.open('detected_{:}'.format(img_path))
detected.show()
detect_objects('test.jpg', ['bear'])

YOLOv3 . TensorFlow 2.0 (TF 2.0).