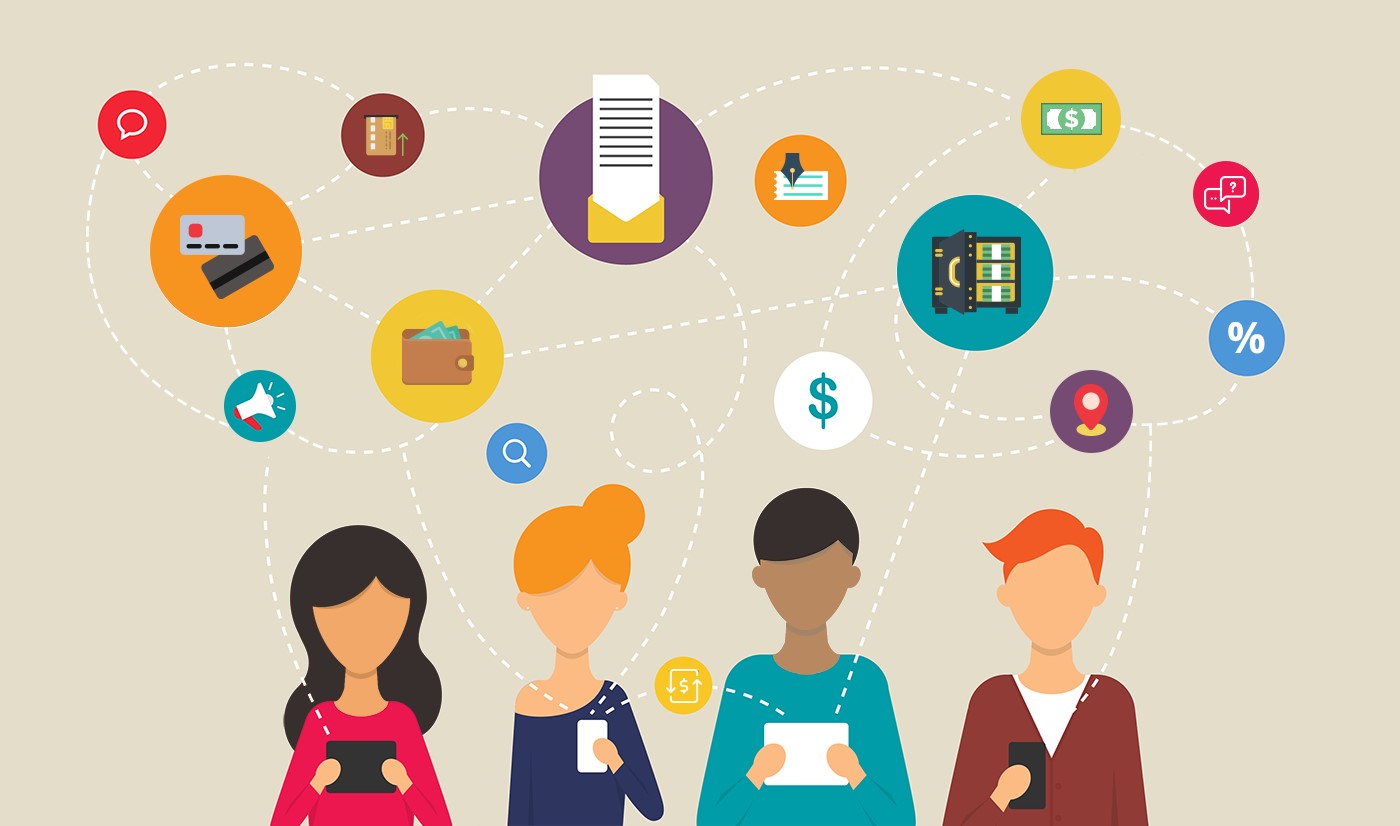
Hello, Habr! My name is Andrey Tyschenko from Dynamic Yield. Our area of work is website personalization in order to increase sales and improve user experience for partner companies. And that is why today I would like to talk about personalization of sites in eCommerce, personalization tools and the results that these technologies can give.
Almost all companies use personalization tools, including eCommerce, Retail, Financial sector, Travel, IT companies and many others. Great results are provided by individual suggestions and recommendations, which have long been implemented by the same Amazon, eBay, Netflix and many other companies. Under the cut - interesting details about personalization tools and a couple of cases.
High technology and personalization
It is worth noting that although major eCommerce players say they are using machine learning, AI, neural networks and similar technologies for personalization purposes, in reality this is not always the case. Relatively simple algorithms for working with data are often used that are neither a neural network nor machine learning - perhaps quite a stretch. At the same time, if these technologies are really used, they allow you to get a good increase in sales / traffic. However, you need to understand well where and for what what tools can be used.
Another interesting point - in the United States and Russia, companies are on a par in terms of using high technologies for personalization. Some examples of the most popular technologies are the Deep Learning and Predictive Targeting engines.
Why are they popular? Because they make it possible to quickly test ideas that arise at the very first stage of personalization, when marketers and product managers come up with hypotheses regarding the UI / UX of sites, marketing campaigns or promotions.
The essence of the approach to building personalization is to identify a trend, build a hypothesis, quickly test it, and then “just” quickly test dozens more options for implementing this hypothesis and, as a result, create an optimal communication strategy with one segment or another (or even with “segment of one ”- that is, a communication strategy in 1: 1 mode - in its own way with each individual user). The personalization strategies of the best players in this field, such as Amazon, Netflix, Spotify, and others, take into account not only the explicit actions of users, such as clicks on products, add to cart, purchases (or watching movies / listening to music tracks), etc. .p., but also take into account the characteristics of goods (films, tracks, etc.), patterns of user behavior on the site,comparison of all users on the site and the interaction of all users with all products and prediction of the most likely next user action. After the user has interacted with the site for a long enough time (say, a few minutes), the content automatically begins to adapt to his preferences. On the next visit of the client, all pages of the site, including the home page, are personalized.
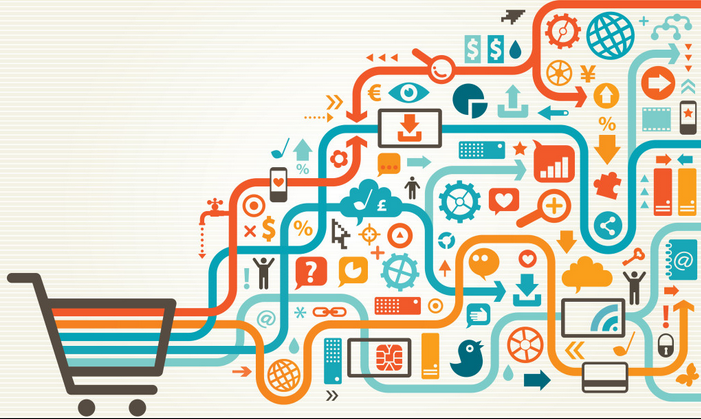
You can personalize not only websites, but also mobile applications. An example is SimpleWine. She did it:
- Achieve comprehensive personalization of the mobile application. Not only recommendations, but entire screenshots of the application are rebuilt for the user and look different.
- Make it easier for the user to choose. The app helps you to choose a wine with tips, navigation and recommendations - just like a competent wine consultant.
- Ensure the launch of any changes in the application in real time - that is, there is no need to wait for the build of a new release and upload it to the application catalog.
Further plans include the introduction of Deep Learning an engine that predicts the preferences and interests of users not on formal grounds, but on the basis of comparing this user with all other users and predicting what other wine will interest this user with the greatest probability based on recognition of other users with similar tastes and their patterns of behavior, much like movies or music on Netflix or Spotify are matched to you.
Okay, can you be more specific about personalization technologies?
There are quite a few of them, but not all of them are effective. Personalization technologies can be conditionally divided into two groups, according to the time of work with the user.
Interaction with the user on the site. It is important to take into account the interests and preferences of the buyer, helping him to make a good purchase. Personalization makes it possible to customize the appearance and functions of the site in real time to the needs of the user in accordance with his behavior. For example, the product issue automatically changes, and its formation is influenced by the user's interest in different products, categories, brands, price range, etc. Analysis systems are now able to take into account a huge number of factors related to a particular buyer.
Communication with the user after he closed the website of the online store. This is where newsletters, trigger letters, sms, push notifications, instant messengers, etc. come into play. Of course, important conditions are unobtrusiveness, relevance and timeliness of communication.
As for the technologies themselves, most often we work with tools such as:
Predictive Targetingbased on machine learning, predicts the best combination of a communication option or content and a segment for which such communication will work best, and suggests using the optimal targeting for this or that communication option. Thus, the engine informs marketers or product managers in real time which communication option is best to show and for which segment. In this case, manual analysis is not needed, everything works in a fully automatic mode. After the “pre-production” targeting is completed, the engine notifies the marketer or “product”, making it possible to quickly deploy the optimal setting.
It turns out such a combination of a person who has initial (as a rule, rather simple) hypotheses, ideas, tasks, and a machine that “falls through” into the data, in the context of all possible segments, “spins” a person’s logic and builds a smarter one, complex and granular personalization.

Sorting Engine (Ranking Engine).Helps to automatically optimize product sorting on product listing pages using a sophisticated Deep Learning model. At the same time, the listing of goods is formed in real time, individually for each user. In the process of formation, data about the interests of a potential client is used, and a prediction of actions is performed based on comparison with the behavior of other users. Moreover, this process also uses data on the balance of goods, margin, promotions, etc.
Server API.A set of APIs allows product managers, developers to experiment on the server side with any device, develop product recommendations, and introduce new features based on customer interests. And all this is adjusted for each individual buyer.
Omnichannel... Here we are talking about the fact that there are many data collection channels for subsequent analysis. This can be a call center, cash register, terminals and other devices in offline stores, mobile devices of sales assistants (the so-called Clientelling), electronic menus in restaurants, applications for Smart TV or Apple TV, smart watches, smart speakers, smart glasses. and many others. By the way, when personalizing, it is very important to take into account from which device the user will perform certain actions with the storefront or goods.
Do you need cases? And here they are
In order to demonstrate the effectiveness of personalization and give examples of how technologies work, we will point out several cases.
The largest marketplace in Kazakhstan Kaspi.kz
This is one of the largest innovative companies in the country, the application of which is very popular. It allows users to make payments and transfers, manage personal finances, and shop online with delivery. Today Kaspi.kz is the largest e-commerce platform in Kazakhstan with a turnover of about $ 600 million in 9 months of 2020 and an increase of + 129% compared to last year.
With the help of personalization technologies, the company achieved 19.4% of sales from referrals in just three months. And this is a huge amount when you consider these facts:
- 450 000 .
- 8 500 000 .
Perekrestok store
The online supermarket was launched in 2017. Since then, the company has grown tremendously. Its revenue increased to 2.09 billion rubles, the number of orders exceeded 590 thousand, while the average check amounted to 4026 rubles.
The company decided to implement personalization technology on its website and it was right. Only the product recommendation strategy with similar products on the product card showed an increase in conversion of + 10.9% relative to the control group, and + 12.0% in revenue for the desktop.

In addition, Perekrestok has added a widget with promotional items on the item card. This managed to increase revenue on the desktop by 2.5%, and on the mobile version - by 1%. The segmentation of the audience additionally led to an increase in revenue for new customers by 10%, and for current ones - by 5%.
Well, the "Empty Search" widget, which offers personalized product recommendations in case of a failed search, increased revenue per user by 3.1%. The maximum revenue growth from the empty search widget was 6.2%.
Behind all these cases is a system based on machine learning, including Deep Learning algorithms, which, based on data on the behavior and interests of users, as well as on the properties of goods, selects for each user the goods that are most likely relevant to him in every moment of working with the site. Machine learning helps to analyze which segment of customers which users belong to, and offer them the most interesting offer and choose an individual strategy for using personalized recommendations.
As a conclusion, it should be said that personalization in the next few years will penetrate all business sectors, including large players in the retail sector, banks, and to small companies. Machine learning, data processing makes it possible to make personalization as effective as possible. But there is another important nuance that should not be forgotten - this is the protection of user data. The data collected must be secure - without this, personalization cannot be used.