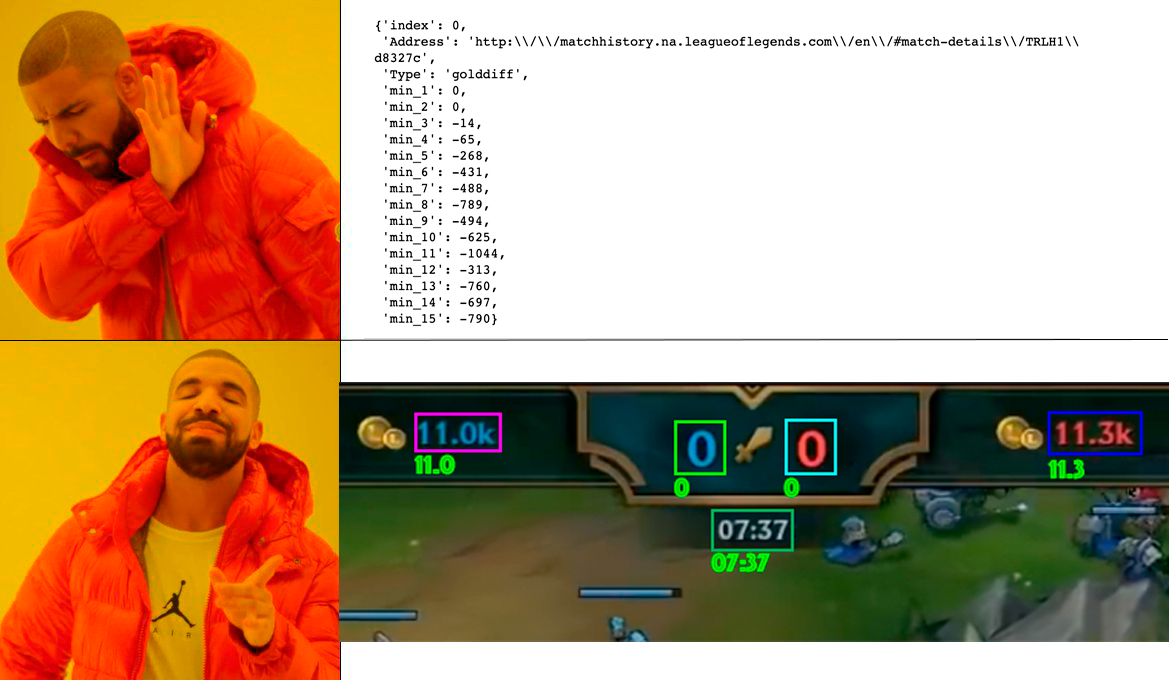
Hello, Habr!
Today I dare to tell you how I happened to extract data directly from video recordings of League of Legends tournament games using deep neural networks: why is it needed, what architectures and techniques were used, and what difficulties I encountered.
Step 0: figuring out what's what
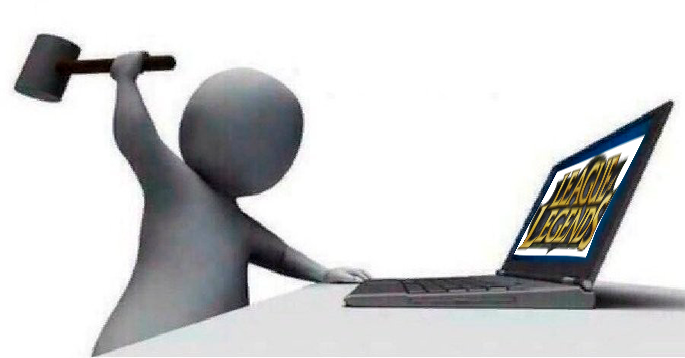
League of Legends ( LoL ) is a popular MOBA game with a monthly audience of over 100 million players worldwide. LoL was developed by Riot Games and released back in 2009.
Riot Games . , , - . - . ...
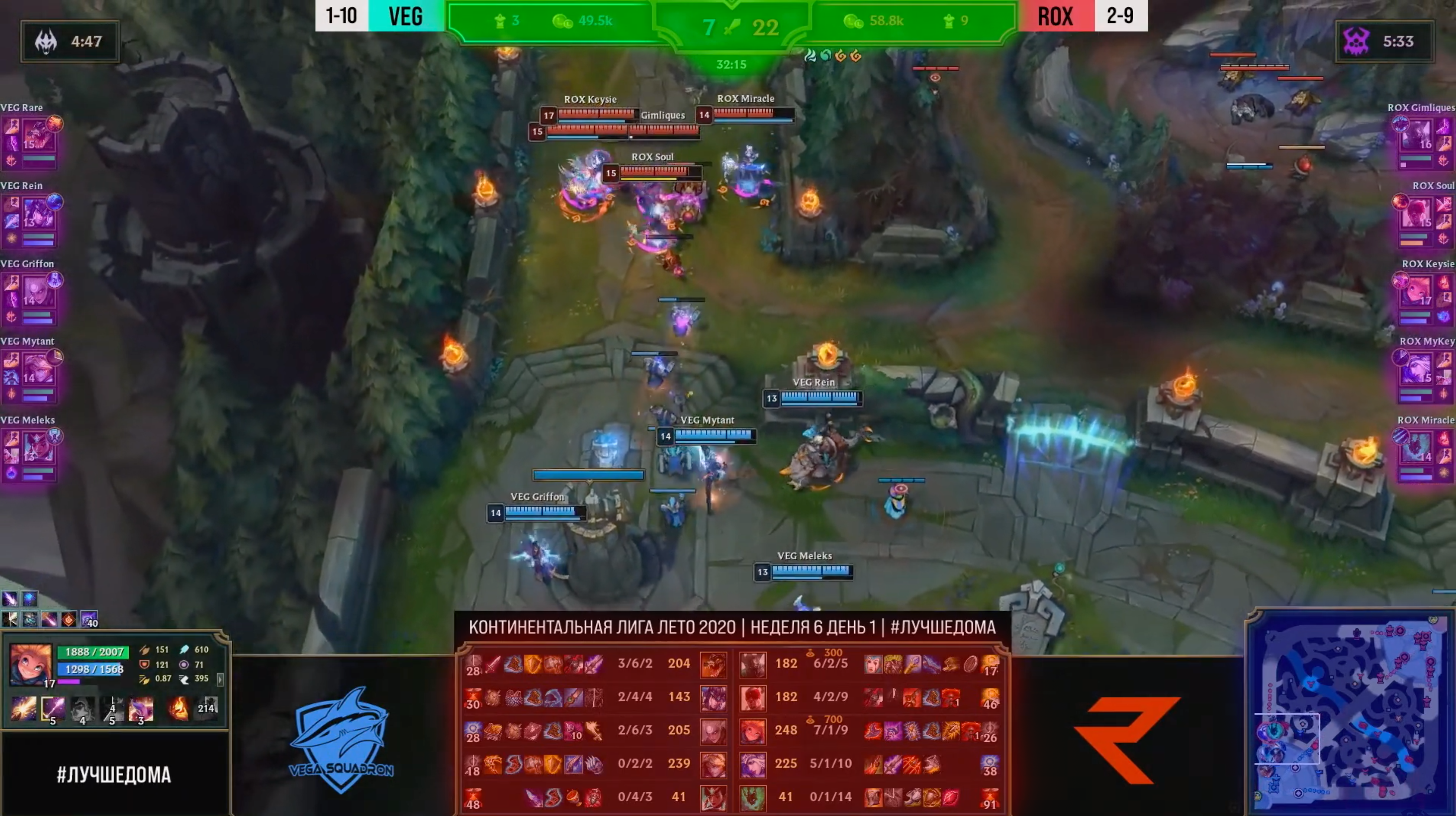
HUD- (Heads-Up Display - ). :
( ) - , , : , - - , - , , .
( ) - , - . , .
- ( ) - -, , . - , , .
( ) - , , : K/D/A (Kills/Deaths/Assists), - , .
1:

. CVAT , . , , .. (Google OCR, Yandex OCR) , (Tesseract OCR, EasyOCR).
() . segmentation-based . Unet
c efficientnet instance : ( ), ( ) - ( ). segmentation_models.pytorch. Pytorch Lightning .
, watershed . . SVHN
, multihead . , ( ), RNN . , 11- (11- , ) . , . Pytorch .
, . .. . : 2D . , , , . 3D , , N , N+1.
: , , , , .

2:
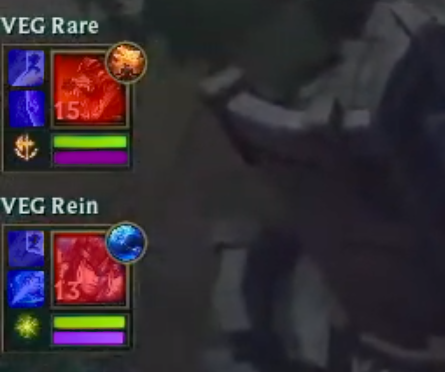
, : ( ), ( ), ( ). Unet .
: - .
. HSV ( ) : . : ( ) . x- , - / . , , , .
( ).
, . , multihead . , , (/ ).
, , , , . , (, - LoL) . , , 20-
20- . , 20-, 100 , argmax ( , ).
. . , , , . - , OOD (Out-of-Domain), , , metric learning. . - - hinge-loss hard-negative triplet , .
?
hinge-loss- ?
. , .. pattern recogntion. pattern recogntion , .
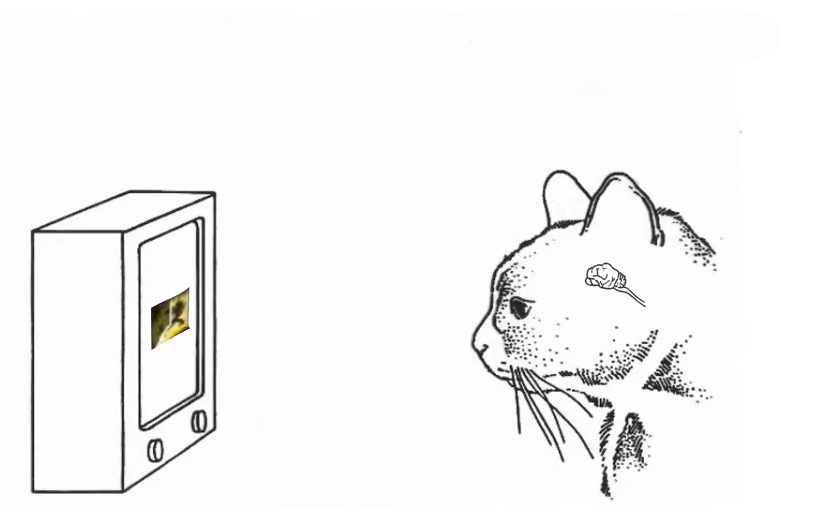
3: -
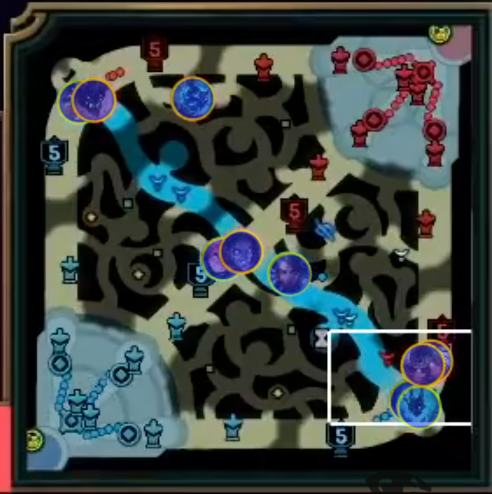
, - . Farza ( ) Yolo, , . , .
Yolo - anchor-based , . segmentation-based . . : ( ), ( ). instance , , .
Unet.
watershed . , . , , . , .
, . -.
N:
, , , Riot Games. , , , .
I would also like to apologize for not providing the source code of the resulting framework and omitting some points of training networks.
Thanks for attention!