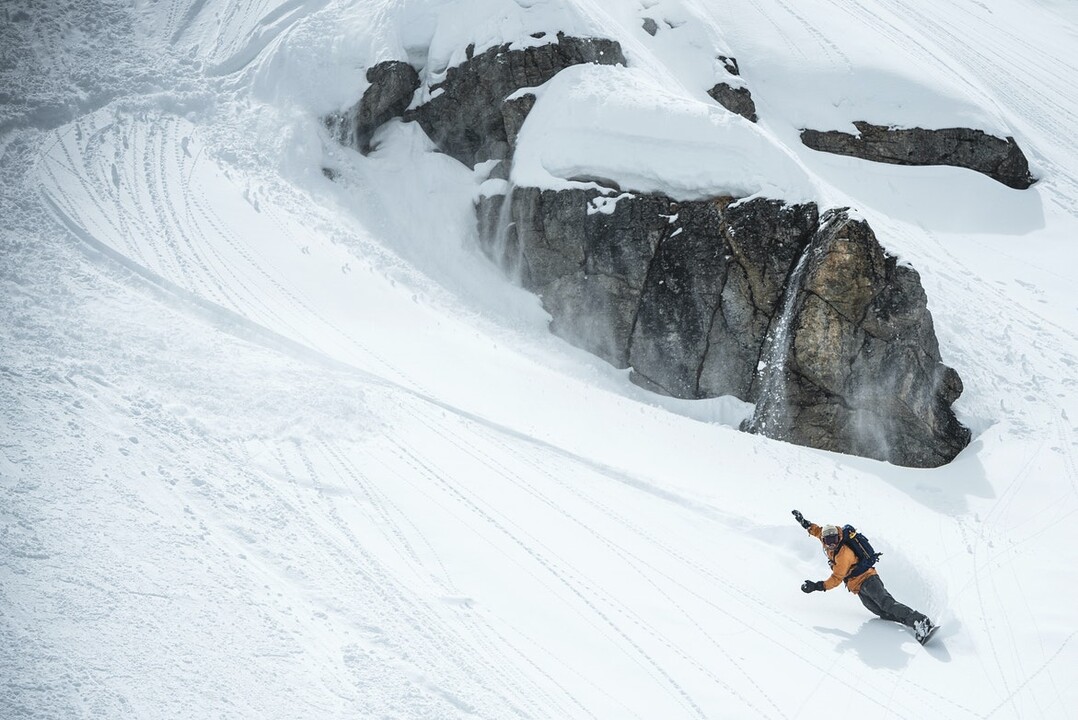
Challenge and requirements
The main goal is to create an algorithm that will find the maximum value modulo the minimum at a given radius.
The algorithm must be efficient and work fast enough
The result should be displayed on a graph
Introduction, description of the algorithm
The working area of ββthe function (specified interval) is divided into several points. Points of local minima are selected. After that, all coordinates are passed to the function as arguments, and the argument that gives the smallest value is selected. Then the gradient descent method is applied.
Implementation
, numpy sinus, cosinus exp. matplotlib .
import numpy as np
import matplotlib.pyplot as plot
radius = 8 # working plane radius
centre = (global_epsilon, global_epsilon) # centre of the working circle
arr_shape = 100 # number of points processed / 360
step = radius / arr_shape # step between two points
arr_shape 100, , , . , .
, :
def differentiable_function(x, y):
return np.sin(x) * np.exp((1 - np.cos(y)) ** 2) + \
np.cos(y) * np.exp((1 - np.sin(x)) ** 2) + (x - y) ** 2
:

, :
global_epsilon = 0.000000001 # argument increment for derivative
:

(x, 0), :
def rotate_vector(length, a):
return length * np.cos(a), length * np.sin(a)
Y, - y:
def derivative_y(epsilon, arg):
return (differentiable_function(arg, epsilon + global_epsilon) -
differentiable_function(arg, epsilon)) / global_epsilon
X, - x:
def derivative_x(epsilon, arg):
return (differentiable_function(global_epsilon + epsilon, arg) -
differentiable_function(epsilon, arg)) / global_epsilon
:

2D-, k
gradient = derivative_x(x, y) + derivative_y(y, x)

.
. : https://en.wikipedia.org/wiki/Maxima_and_minima
def calculate_flip_points():
flip_points = np.array([0, 0])
points = np.zeros((360, arr_shape), dtype=bool)
cx, cy = centre
for i in range(arr_shape):
for alpha in range(360):
x, y = rotate_vector(step, alpha)
x = x * i + cx
y = y * i + cy
points[alpha][i] = derivative_x(x, y) + derivative_y(y, x) > 0
if not points[alpha][i - 1] and points[alpha][i]:
flip_points = np.vstack((flip_points, np.array([alpha, i - 1])))
return flip_points
flip_points, :
def pick_estimates(positions):
vx, vy = rotate_vector(step, positions[1][0])
cx, cy = centre
best_x, best_y = cx + vx * positions[1][1], cy + vy * positions[1][1]
for index in range(2, len(positions)):
vx, vy = rotate_vector(step, positions[index][0])
x, y = cx + vx * positions[index][1], cy + vy * positions[index][1]
if differentiable_function(best_x, best_y) > differentiable_function(x, y):
best_x = x
best_y = y
for index in range(360):
vx, vy = rotate_vector(step, index)
x, y = cx + vx * (arr_shape - 1), cy + vy * (arr_shape - 1)
if differentiable_function(best_x, best_y) > differentiable_function(x, y):
best_x = x
best_y = y
return best_x, best_y
:
def gradient_descent(best_estimates, is_x):
derivative = derivative_x if is_x else derivative_y
best_x, best_y = best_estimates
descent_step = step
value = derivative(best_y, best_x)
while abs(value) > global_epsilon:
descent_step *= 0.95
best_y = best_y - descent_step \
if derivative(best_y, best_x) > 0 else best_y + descent_step
value = derivative(best_y, best_x)
return best_y, best_x
:
def find_minimum():
return gradient_descent(gradient_descent(pick_estimates(calculate_flip_points()), False), True)
:
def get_grid(grid_step):
samples = np.arange(-radius, radius, grid_step)
x, y = np.meshgrid(samples, samples)
return x, y, differentiable_function(x, y)
:
def draw_chart(point, grid):
point_x, point_y, point_z = point
grid_x, grid_y, grid_z = grid
plot.rcParams.update({
'figure.figsize': (4, 4),
'figure.dpi': 200,
'xtick.labelsize': 4,
'ytick.labelsize': 4
})
ax = plot.figure().add_subplot(111, projection='3d')
ax.scatter(point_x, point_y, point_z, color='red')
ax.plot_surface(grid_x, grid_y, grid_z, rstride=5, cstride=5, alpha=0.7)
plot.show()
main:
if __name__ == '__main__':
min_x, min_y = find_minimum()
minimum = (min_x, min_y, differentiable_function(min_x, min_y))
draw_chart(minimum, get_grid(0.05))
:

The process of calculating the minimum value using the algorithm may not be very accurate when calculating at a larger scale, for example, if the radius of the work plane is 1000, but it is very fast compared to the exact one. Plus, in any case, if the radius is large, the result is approximately in the position in which it should be, so the difference will not be noticeable on the graph.
Source:
import numpy as np
import matplotlib.pyplot as plot
radius = 8 # working plane radius
global_epsilon = 0.000000001 # argument increment for derivative
centre = (global_epsilon, global_epsilon) # centre of the working circle
arr_shape = 100 # number of points processed / 360
step = radius / arr_shape # step between two points
def differentiable_function(x, y):
return np.sin(x) * np.exp((1 - np.cos(y)) ** 2) + \
np.cos(y) * np.exp((1 - np.sin(x)) ** 2) + (x - y) ** 2
def rotate_vector(length, a):
return length * np.cos(a), length * np.sin(a)
def derivative_x(epsilon, arg):
return (differentiable_function(global_epsilon + epsilon, arg) -
differentiable_function(epsilon, arg)) / global_epsilon
def derivative_y(epsilon, arg):
return (differentiable_function(arg, epsilon + global_epsilon) -
differentiable_function(arg, epsilon)) / global_epsilon
def calculate_flip_points():
flip_points = np.array([0, 0])
points = np.zeros((360, arr_shape), dtype=bool)
cx, cy = centre
for i in range(arr_shape):
for alpha in range(360):
x, y = rotate_vector(step, alpha)
x = x * i + cx
y = y * i + cy
points[alpha][i] = derivative_x(x, y) + derivative_y(y, x) > 0
if not points[alpha][i - 1] and points[alpha][i]:
flip_points = np.vstack((flip_points, np.array([alpha, i - 1])))
return flip_points
def pick_estimates(positions):
vx, vy = rotate_vector(step, positions[1][0])
cx, cy = centre
best_x, best_y = cx + vx * positions[1][1], cy + vy * positions[1][1]
for index in range(2, len(positions)):
vx, vy = rotate_vector(step, positions[index][0])
x, y = cx + vx * positions[index][1], cy + vy * positions[index][1]
if differentiable_function(best_x, best_y) > differentiable_function(x, y):
best_x = x
best_y = y
for index in range(360):
vx, vy = rotate_vector(step, index)
x, y = cx + vx * (arr_shape - 1), cy + vy * (arr_shape - 1)
if differentiable_function(best_x, best_y) > differentiable_function(x, y):
best_x = x
best_y = y
return best_x, best_y
def gradient_descent(best_estimates, is_x):
derivative = derivative_x if is_x else derivative_y
best_x, best_y = best_estimates
descent_step = step
value = derivative(best_y, best_x)
while abs(value) > global_epsilon:
descent_step *= 0.95
best_y = best_y - descent_step \
if derivative(best_y, best_x) > 0 else best_y + descent_step
value = derivative(best_y, best_x)
return best_y, best_x
def find_minimum():
return gradient_descent(gradient_descent(pick_estimates(calculate_flip_points()), False), True)
def get_grid(grid_step):
samples = np.arange(-radius, radius, grid_step)
x, y = np.meshgrid(samples, samples)
return x, y, differentiable_function(x, y)
def draw_chart(point, grid):
point_x, point_y, point_z = point
grid_x, grid_y, grid_z = grid
plot.rcParams.update({
'figure.figsize': (4, 4),
'figure.dpi': 200,
'xtick.labelsize': 4,
'ytick.labelsize': 4
})
ax = plot.figure().add_subplot(111, projection='3d')
ax.scatter(point_x, point_y, point_z, color='red')
ax.plot_surface(grid_x, grid_y, grid_z, rstride=5, cstride=5, alpha=0.7)
plot.show()
if __name__ == '__main__':
min_x, min_y = find_minimum()
minimum = (min_x, min_y, differentiable_function(min_x, min_y))
draw_chart(minimum, get_grid(0.05))