What's wrong with the TargetEncoder from the category_encoders library?
This article is a continuation of the previous article , which explained how objective probabilistic coding actually works. In this article we will see in what cases the standard solution of the category_encoders library gives an incorrect result, and in addition, we will study the theory and code example for correct multi-class objective-probabilistic coding. Go!
1. When is TargetEncoder wrong?
Take a look at this data. Color is a feature, and a goal is ... a goal. Our goal is to encode the color based on the Target.

Let's do the usual objective-probabilistic encoding for this.
import category_encoders as ce
ce.TargetEncoder(smoothing=0).fit_transform(df.Color,df.Target)
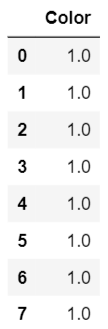
Hmm ... doesn't look good, does it? All colors have been changed to 1. Why? This is because the TargetEncoder takes the average of all target values ββfor each color, not the probability.
While TargetEncoder works correctly when you have a binary target with 0 and 1, it will fail in two cases:
When the target is binary, but not 0/1 (at least, for example, 1 and 2).
When the target is a multiclass like in the above example.
So what to do ?!
Theory
, n . , . n , . n-1 , , . - , , .
.
.
1: - .
enc=ce.OneHotEncoder().fit(df.Target.astype(str)) y_onehot=enc.transform(df.Target.astype(str)) y_onehot
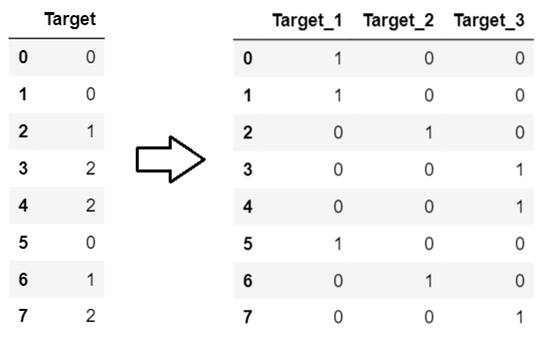
, Target_1 0 Target. 1 Target 0, 0 . Target_2 1 Target.
2: , .
class_names = y_onehot.columns
for class_ in class_names:
enc = ce.TargetEncoder(smoothing = 0)
print(enc.fit_transform(X,y_onehot[class_]))
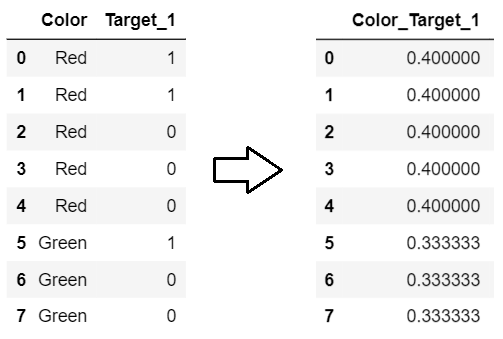
0
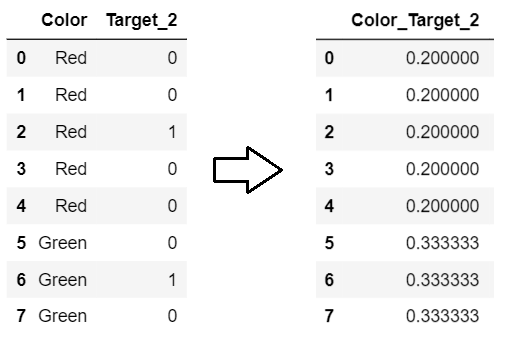
1

2
3: , , 1 2 .
!
, :

, Color_Target. , , . , , , Color_Target_3 ( - ) .
, ?!
Below is a function that takes as input a data table and a target label object of type Series. The df function can have both numeric and categorical variables.
def target_encode_multiclass(X,y): #X,y are pandas df and series
y=y.astype(str) #convert to string to onehot encode
enc=ce.OneHotEncoder().fit(y)
y_onehot=enc.transform(y)
class_names=y_onehot.columns #names of onehot encoded columns
X_obj=X.select_dtypes('object') #separate categorical columns
X=X.select_dtypes(exclude='object')
for class_ in class_names:
enc=ce.TargetEncoder()
enc.fit(X_obj,y_onehot[class_]) #convert all categorical
temp=enc.transform(X_obj) #columns for class_
temp.columns=[str(x)+'_'+str(class_) for x in temp.columns]
X=pd.concat([X,temp],axis=1) #add to original dataset
return X
Summary
In this article, I've shown what's wrong with the TargetEncoder from the category_encoder library, explained what the original article says about targeting multi-class variables, demonstrated it all with an example, and provided a working modular code that you can plug into your application.