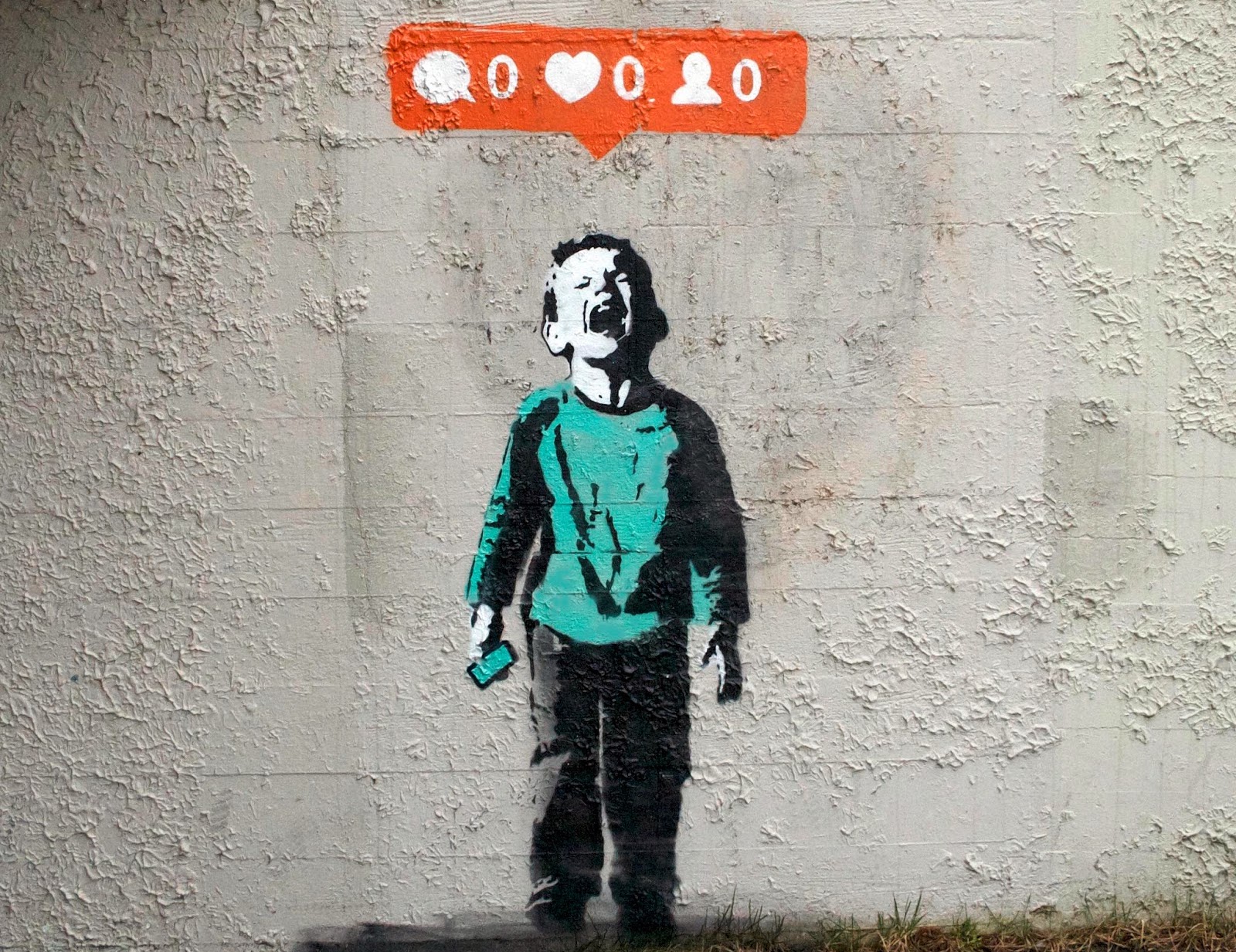
Praise, approval, acceptance and compliment - all these elements of social interaction are very pleasant and go through a thin line throughout a person's life. As a child, we are praised for finishing our dinner or drawing an adorable drawing (which only an abstract art expert can understand), for a good grade in school, etc. As adults, praise becomes less necessary for us, but still brings joy.
Communication and social engagement have changed dramatically with the advent of the internet and social media. Distance and time are no longer decisive factors in communication between people living in different cities. Convenience, of course, has increased, although at times the content of the messages has diminished. At the same time, everyone and everyone got the opportunity to look into the life of another person, without exchanging a word with him. From the point of view of the mechanics of social networks, the concept of "likes" is also important. Someone does not care about them, for someone it is just a curious function, and someone cannot sleep peacefully if a photo from a recent vacation has not gained the desired number of hearts or thumbs up.The question arises - why are some people so hungry for approval on the Internet? What drives them? Scientists from New York University have found similarities between the craving for likes in humans and the process of finding food in laboratory rodents. What are the similarities, what is the neurological foundation underlying the craving for likes, and how can the obtained data be applied in practice? We learn about this from the report of scientists. Go.
Basis of research
The Internet and social networks allow us to exchange information, share ideas, thoughts and experiences, and communicate with people from all over the world. This is a truly amazing and extremely useful invention that does not carry a single drop of harm. I would like to say if we did not know human nature, which is capable of, to one degree or another, seduce anything.
Sometimes it seems like social media has become a place where some people brag about their accomplishments and others hate them for it. This, of course, is a very pessimistic view of the situation, but even there is a grain of truth in it.
We all know the phrase "someone is wrong on the Internet", which at its root indicates an infinite number of opinions about something. Someone can calmly accept this, but someone cannot live without constant verbal skirmishes on the network, which never end the way they would like.
While some users of "social networks" are looking for thrills in the form of Internet disputes, others are looking for approval, complimentary comments and universal love. These are completely normal manifestations of the human psyche, but everything has its own healthy limit. If likes are for a person a by-product of social activity on the network, then this is reasonable. If likes become the reason, purpose and engine of human existence, then it's time to think seriously.
Considering this problem from a scientific point of view, many researchers tried to understand why a person behaves differently on the Internet, why he becomes dependent on social networks, why he craves likes, etc. Naturally, the answers to these questions can be very individual, because how many people - so much psychological baggage accumulated in the past.
In general terms, many studies are aimed not so much at understanding human nature as at improving software so that this nature can be used for the benefit of the social network behind this research. Nevertheless, as scientists say, even in such works, a lot of interesting things can be learned.
For example, it was found that receiving a negative reaction to a post (dislike, etc.) led to a deterioration in the quality of subsequent posts, and not to its improvement. Other studies have shown that a person will spend more time on a forum where they receive more responses. These cases point to the reward mechanism underlying both human behavior on the Internet and many living organisms in nature.
In the work we are considering today, scientists decided to test whether it is possible to comprehensively characterize interaction in social networks as a form of learning for a reward. The study analyzed more than a million messages (posts) from 4,000 users on different platforms. The data were evaluated using computer simulations.
The hypothesis, which the authors of the study operate on, is based on the theory of computational learning, which is used in the development and analysis of machine learning algorithms. This theory can convert human behavior on the network into a "reward for learning" format.
The underlying empirical understanding is that when an animal (such as a rodent in Skinner's box) can choose the timing of its instrumental responses (such as when and how often to press the lever), response latency (the inverse of response rate) negatively affects the amount of rewards accrued.

An example of a Skinner box diagram - a special laboratory chamber required for the controlled study of animal behavior.
In other words, the worse the reward, the greater the response delay. In experiments with rodents, subjects very quickly realized that faster actions lead to more frequent rewards, while slower actions increase the delay time until the next reward. If you translate this into the framework of this study, then the more reward a person receives, the shorter the average delay between responses should be, because slower action leads to a longer delay until the next reward.
Preparing for experiments
To test the theory that social behavior on the Internet in the form of messages (posts) follows the principles of the theory of reward for learning, scientists analyzed datasets from various social networks using computer simulations. In total, 1,046,857 posts from 4,168 users were used in the study. The research was divided into several stages.
At the first stage (2039 users), the hypothesis was tested on a large dataset of Instagram posts (average number of posts per person = 418). This social network has about a billion users, and the format of the posts is quite simple, as is the response in the form of “likes”. However, according to the authors of the study, Instagram, like many other modern social media. networks, financial benefits play an important role. This can lead to multiple fake users and fake likes.
For this reason, the first phase was expanded to 2,127 users, thereby transforming into the second phase. Additional data was taken from thematic sites (discussion forums for men's fashion, women's fashion and gardening), where financial motives are less likely (average number of posts per person = 91).
The third stage was to conduct an online experiment, which involved 176 people. The experiment was designed to mimic key aspects of social media, where scientists could manipulate the level of social reward to test its causal effect on response delays.
Human action on a social network (for example, Instagram) was compared to the action of a rodent in Skinner's box: posting - activating a lever; like is a reward.
Having established the fact that social media behavior is dependent on reward, scientists have developed a generative model based on the theory of the free operand in non-human animals. A key tenet of this theory is that subjects must balance the cost of response effort and the opportunity cost of passivity (i.e., publication-related rewards that a person misses without posting) in order to maximize the average net (i.e., profit minus losses) interest rate. The consequence of this is that the average response delay should be less when the average reward rate is higher. This technique is applicable both in the case of instant reward, and in the case when a certain time passes between the post and the reward.
Based directly on these principles, the model created determines how subjects adjust their response latency to maximize the average level of net reward ®.
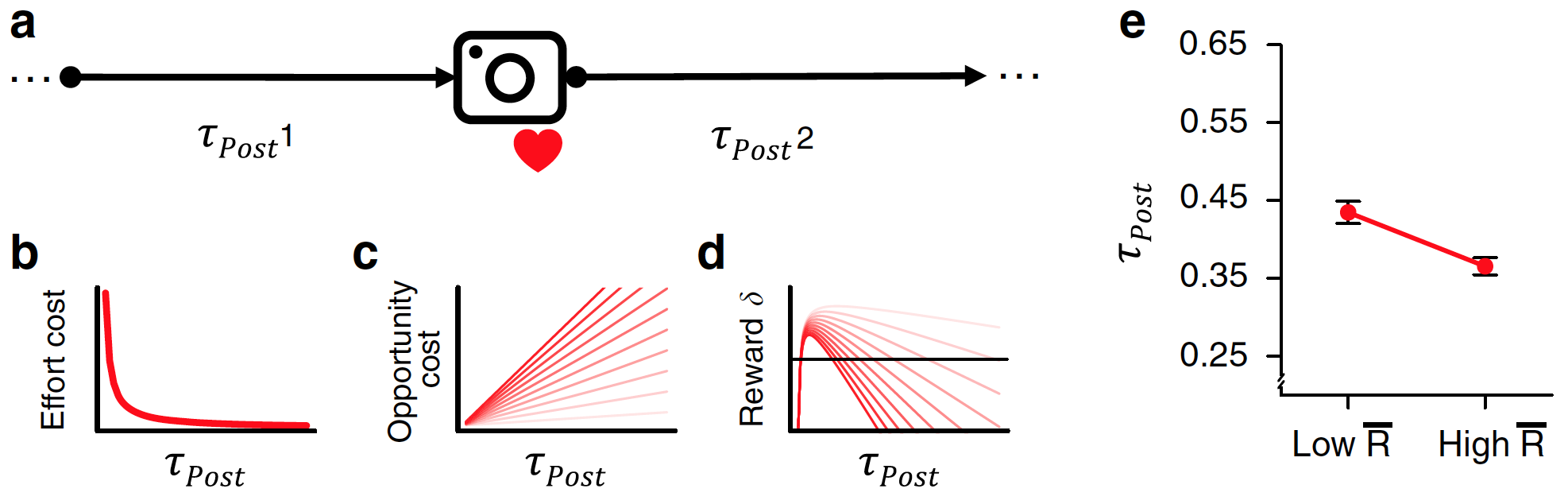
Image # 1
Formally, the model describes the use of social media as a sequence of decisions regarding the delay between successive publications (τ Post , 1a ), where a person maximizes the reward ratio by adaptively adjusting τ Post after observing each reward received.
Psychologically τ Postcan be seen as the accumulation of motivation for the threshold value for publication. The threshold determining τ Post is dynamically adjusted based on the forecast error of the net remuneration (δ) - the difference between the received and the reference level of remuneration.
The reference level is defined both by individual sensitivity to the cost of effort (for example, the subjective cost of photographing and uploading), and by the subjective assessment of the average net remuneration rate ( 1b and 1c ). Effort cost and opportunity cost depend on the response delay τ Post... In other words, the optimal response latency balances these two costs to maximize the net reward δ ( 1d ).
As a result, a model was created with ~ 250,000 data points from 1000 simulated users with random parameter values. According to learning theory, τ Post should be lower when the average remuneration rate is relatively higher. To test this, R was standardized and then the variable was dichotomized * by 0 to obtain a qualitative predictor of “Low vs High R” (low versus high R).
Dichotomization * - treating continuous data as if it were binary variables.The analysis revealed a clear effect of low and high R on τ Post , as expected. In other words, the model predicts (given a set of simulation parameters) that the average response delay should be ~ 18% longer when the average reward rate is low versus high ( 1e ).
Simulation results
As we already know, the first phase of the study was related to data analysis of online behavior on Instagram. Initially, a comparison was made of two models that can explain human behavior in the network, namely the used model of “learning for reward” (RL for reinforcement learning ) and the model without learning. As a result, it was found that the RL model is suitable for more than 70% of the reviewed users and their posts ( 2a ).

Image No. 2
Interestingly enough, the discovery that people with a large number of Instagram followers showed a non-linearly decreasing subjective value (usefulness) of likes. In other words, there is an addiction to likes - the larger and more stable the flow of likes, the less a person is involved in their further receipt and increase.
According to the theory of scientists, the response of a person in the network should be faster if the reward is greater. This was also confirmed on Instagram (N Obs = 851946, N Users = 2039), where the delay between posts got smaller when the number of likes ® was relatively high: β = -0.18, SE = 0.003, t = -54.59, p <0001 ( 2b). When recalculated, this means that an increase in reward (likes) from a low to a relatively high level resulted in an 18% decrease in latency between posts (equivalent to 8 hours). In the case of a linear increase in likes of 1%, there was a decrease in the time between posts by 0.34% (about 5 minutes).
Graphs 2c and 2d show the relationship between τ Post , R and the structure of the RL model using the example of the activity of one user over two years.
At the second stage of the study, the results obtained in the first one had to be verified by introducing additional variables. In this case, they were additional social platforms, namely forums with a narrow theme (men's fashion, women's fashion and gardening). Obviously, most of the posts on such a platform are in the form of text, and not images, as in Instagram. Nevertheless, such forums had separate topics with mostly graphic content (for example, “What are you wearing today?”, “Share photos of your garden,” etc.), each of which contained several thousand posts.
Once again, it was verified that the RL model is the fundamental mechanism of online behavior, as in the case of Instagram. In all three datasets (190,721 posts from 2,127 people), regardless of the specific topic, when comparing the models, it was found that RL models, rather than non-training models, are suitable for the vast majority of cases.
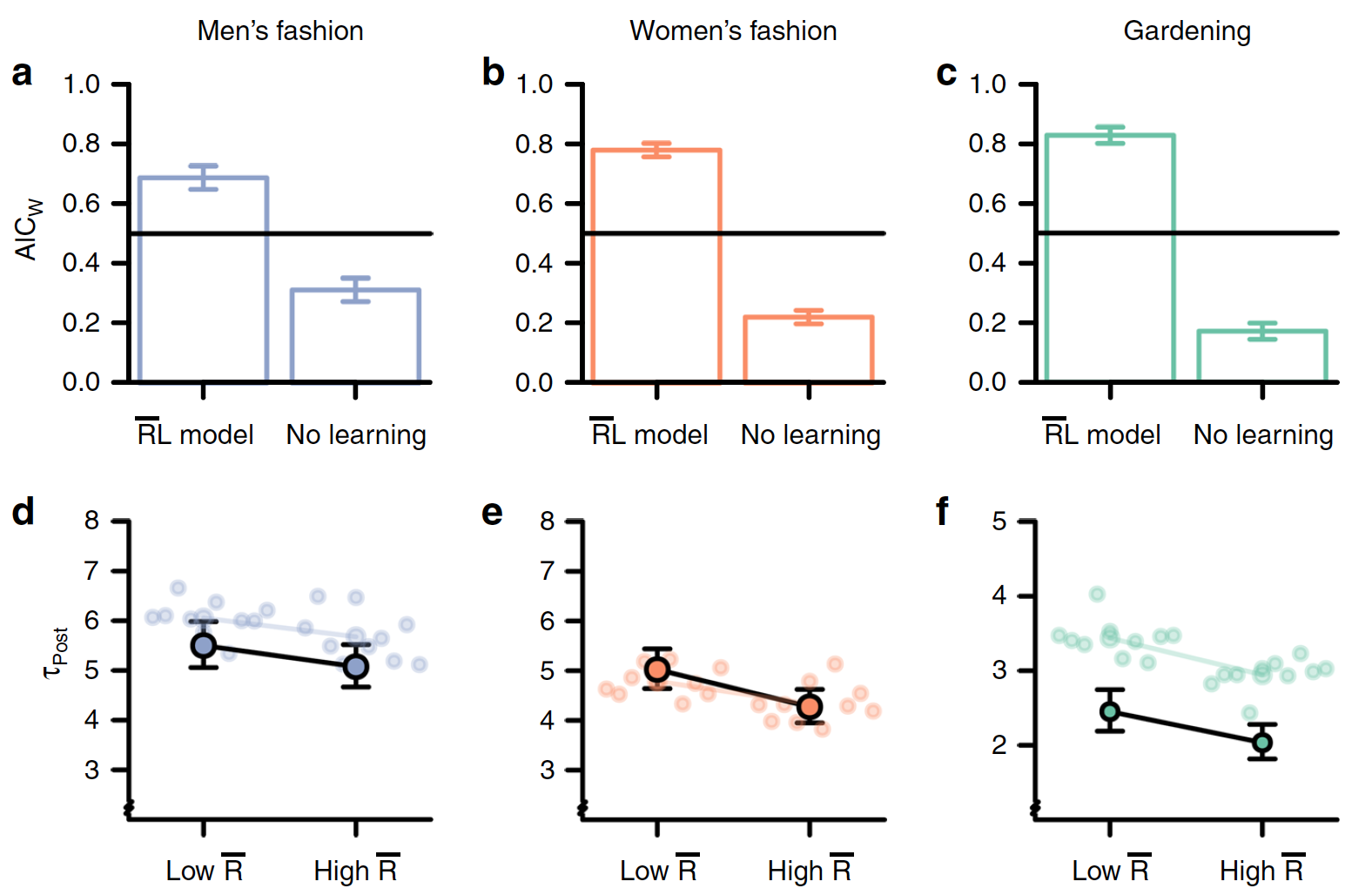
Image # 3
As expected, higher R predicted faster response in all three datasets ( 3d - 3f ):
- men's fashion (N Obs = 36 139, N Users = 541): β = –0.08, SE = 0.016, t = –5.1, p <0001;
- (NObs = 36 434, NUsers = 773): β = −0.16, SE = 0.02, t = −7.1, p <0001;
- (NObs = 118 148, NUsers = 813): β = −0.18, SE = 0.02, t = -12.09, p <0001).
Thus, in these datasets, delays between publications were 8%, 16%, and 18% less when the average remuneration rate was high rather than low. Therefore, for every 1% increase in subjective remuneration rate, there is a 0.18%, 0.41%, and 0.38% decrease in average latency between posts, respectively.
The expansion of the analyzed data by combining platforms that are quite different in nature (Instagram and thematic forums) shows that regardless of the social network, human behavior will follow the principles of the RL model.
Now it was necessary to establish whether there are individual differences in the behavior of different people within the framework of the RL model, and also what they can be caused by. For example, individual differences in learning rate have previously been associated with both genetic differences and developmental differences between people. And individual differences in sensitivity to the cost of effort were associated with the dopaminergic system (synthesis and production of the neurotransmitter dopamine, which is involved in feelings of satisfaction).

Image No. 4
To search for possible subgroups in the multivariate data, three parameters of the original RL model, estimated for each person from stages I and II (total number of users = 4168), were used as input to clustering k-means. A quantitative assessment using several standard criteria showed that four main clusters can be distinguished, i.e. 4 subgroups ( 4a ). These clusters ranged from 41% (1,739 people) to 7% (299 people) of the total dataset.
Figure 4bshows four putative computer phenotypes that are behavioral in nature. For example, people in cluster 1 have a relatively low learning rate (ɑ). These people are the least sensitive to social reward in their social media behavior. People from cluster 2 are characterized by low effort costs and average learning rates, while cluster 4 demonstrates the opposite relationship between learning rates and effort costs (cluster 3 is intermediate). Thus, people in clusters 2 and 4 readily post in response to social rewards, although the underlying mechanisms are different.
During the final stage of the study, direct evidence was obtained that the level of social reward affects the delay in posting. To do this, an online experiment was conducted in which scientists could manipulate social rewards and observe responses.
Participants (n = 176) were able to post "memes" anytime they wanted during the 25 minute session (total number of posts = 2206). Then they received likes (from 0 to 19) both from the same participants in the experiment and from fake users.
To test whether a higher social reward rate causes a shorter post response latency, the average number of likes received by participants between the first and second half of the session was increased or decreased (low reward: 0-9 likes per post, high reward: 10-19 likes per post).
As the scientists expected, the average latency between posts was significantly greater when the reward was low (0-9 likes per post) compared to higher (10-19 likes per post), which corresponds to a difference of 10.9% (image # 5 ).

Image No. 5
It's also funny that the participants in the experiment, who had a large number of followers in their real social networks, showed a weaker influence of likes on their behavior during the experiment itself.
This small experiment in practice showed the validity of the theory that there is a direct correlation between reward (likes) and further behavior of a person (response).
For a more detailed acquaintance with the nuances of the study, I recommend that you look into the report of scientists and additional materials to it.
Epilogue
The fact that many people literally cannot live without social networks, likes, reposts, etc., we know without any research. However, this work was able to explain in detail the connection between a person's behavior on the network and his thirst for reward, even if in the form of an illusory heart under a post on the screen of his device.
Basically, life on the Internet is not very different from life in the real world, with the exception of some elements. A person's behavior can change depending on where he communicates, but his behavioral, psychological and even physiological characteristics remain unchanged. The Internet is just a tool that either exaggerates them or visually transforms them, but fundamentally everything remains the same.
The authors of the study themselves believe that their work can be extremely useful in studying the problem of dependence on social networks, which is quite real in our modern world. However, one should not forget that social networks have long ceased to be a “club for insiders”, but have become multibillion-dollar corporations. Therefore, it is likely that this kind of research, although well-intentioned, can indirectly affect the expansion of the range of tools that social networks use to expand their audience. At the same time, I would like to note that there is no need to panic, because the overwhelming majority of progress products have always had and will have two sides of the coin. And the Internet can be pretty accurately described by one of the interpretations of the famous phrase said by Paracelsus: "the difference between poison and medicine is in the dosage."
Thanks for your attention, stay curious and have a good work week, guys. :)
A bit of advertising
Thank you for staying with us. Do you like our articles? Want to see more interesting content? Support us by placing an order or recommending to friends, cloud VPS for developers from $ 4.99 , a unique analogue of entry-level servers that we have invented for you: The Whole Truth About VPS (KVM) E5-2697 v3 (6 Cores) 10GB DDR4 480GB SSD 1Gbps from $ 19 or how to divide the server correctly? (options available with RAID1 and RAID10, up to 24 cores and up to 40GB DDR4).
Is Dell R730xd 2x cheaper in Maincubes Tier IV data center in Amsterdam? Only we have 2 x Intel TetraDeca-Core Xeon 2x E5-2697v3 2.6GHz 14C 64GB DDR4 4x960GB SSD 1Gbps 100 TV from $ 199 in the Netherlands!Dell R420 - 2x E5-2430 2.2Ghz 6C 128GB DDR3 2x960GB SSD 1Gbps 100TB - From $ 99! Read about How to build the infrastructure of bldg. class with the use of Dell R730xd E5-2650 v4 servers at a cost of 9000 euros for a penny?