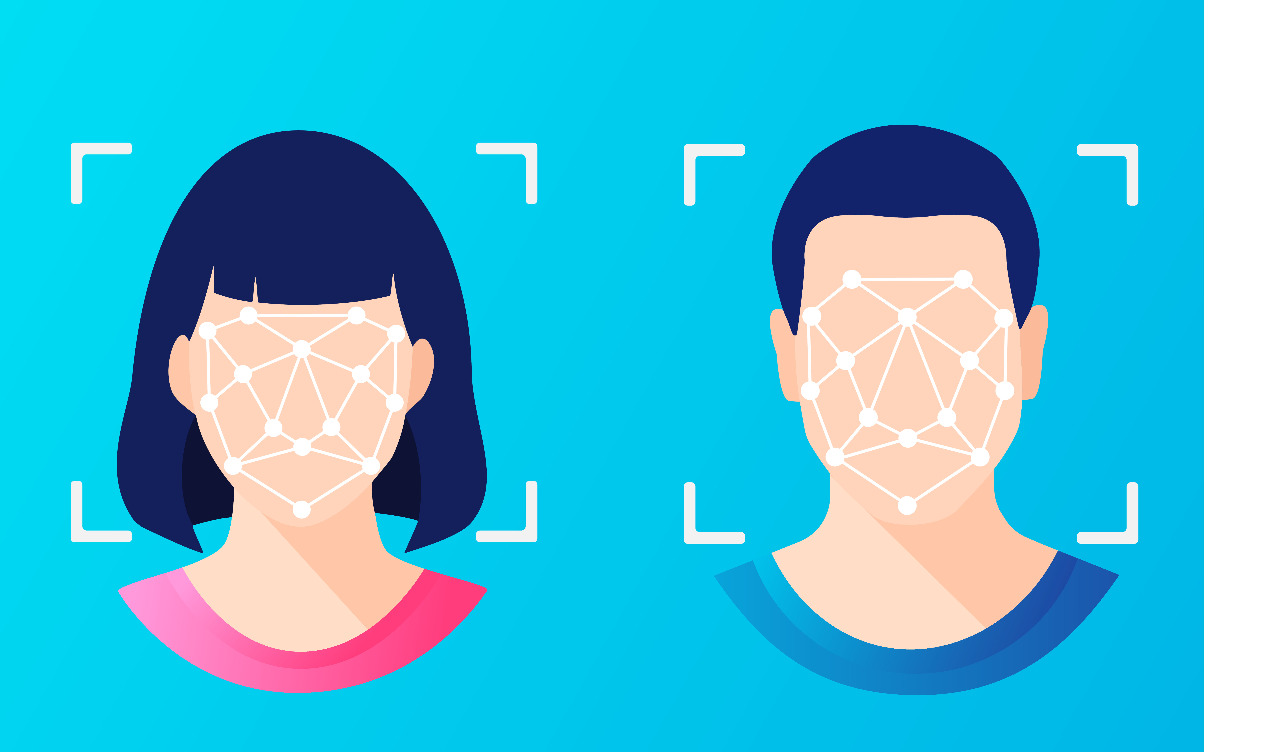
Programmed racism
Face photo data is the basis for computer vision systems. These sets are often labeled according to the race of the individuals in a particular dataset. However, in reality, race is an abstract and vague concept. When creating categories, little attention is paid to the validity, structuring, and stability of this information. This means that people forming datasets have the opportunity for conscious or unconscious manifestation of racism when forming data sets.
Researchers at Northeastern Massachusetts University Zayed Han and Yun Fu examined face tags in datasets in the context of racial categories. Scientists claimthat tags are unreliable because they systematically encode racial stereotypes. Some datasets use characteristics that are too vague, such as "India / South Asia" or "people with ancestors from countries in Africa, India, Bangladesh, Bhutan and other countries." And sometimes labels are used that can be interpreted as offensive - for example, "Mongoloid".
The researchers write that the commonly used standard set of racial categories (Asian, Black, White) is unable to represent a significant number of people. For example, this scheme excludes Native American peoples. It is unclear what label to put on the hundreds of millions of people living in the Middle East or North Africa. Another discovered problem is that people perceive the racial identity of certain individuals differently. Thus, in one of the datasets, Koreans were considered more Asian than Filipinos.
It is theoretically possible to expand the number of racial categories, but they will be unable to describe, for example, mestizo. National or ethnic origin can be used, but country borders are often the result of historical circumstances that do not reflect differences in appearance. In addition, many countries are racially heterogeneous.
The researchers warn that race prejudices can be multiplied and reinforced if left unaddressed. Facial recognition algorithms are susceptible to various biases. Datasets should have as many correctly described races as possible to avoid any discrimination. All ethnic groups should be represented in the digital world, no matter how small they are.
Programmed sexism
As for the algorithms for generating texts and images, they can also broadcast incorrect beliefs. In a sense, they are the personification of the collective unconscious Internet. Negative ideas are normalized as part of learning algorithms.
Researchers Ryan Steed and Eileen Caliscan conducted an experiment - they uploaded photographs of the faces of men and women to services that add cropped images. In 43% of cases, the algorithm offered men a business suit. For women in 53% of cases, the algorithm generated a top or a suit with a deep neckline.
In 2019, researcher Keith Crawford and artist Trevor Paglen discoveredthat tags in ImageNet, the largest dataset for training computer vision models, contain offensive words. For example, "slut" and incorrect race names. The problem is that these datasets are based on data from the Internet, where many stereotypes about people and phenomena circulate.
The researchers emphasize that images are very fuzzy data, burdened with many ambiguous meanings, insoluble questions and contradictions. And the developers of machine learning algorithms are faced with the task of studying all the nuances of the unstable relationship between images and values.
Need more photos
Researchers Deborah Raji and Genevieve Fried examined 130 face datasets (FairFace, BFW, RFW, and LAOFIW) collected over 43 years. As it turned out, as more data grew, people gradually stopped asking for consent to use their images for use in datasets.
This resulted in the datasets including photos of minors, photos with racist and sexist descriptions, and low quality images. This trend may explain the reason why police regularly mistakenly arrest people based on facial recognition data.
At first, people were very wary of collecting, documenting, and verifying facial data, but today no one cares anymore. โYou just can't track a million faces. After a certain point, you can't even pretend that you have control. We collect private information from at least tens of thousands of people, which in itself is the basis for harm. And then we accumulate all this information that you cannot control to build something that is likely to function in a way that you cannot even predict, โsays Deborah Raji.
Therefore, you shouldn't think of machine learning algorithms and data as entities that objectively and scientifically classify the world. They are also subject to political, ideological, racial prejudices, subjective assessment. And judging by the state of large and popular datasets, this is the rule, not the exception.
Blog ITGLOBAL.COM - Managed IT, private clouds, IaaS, information security services for business: