
We all want to be aware of what is happening, so we spend part of our time reading the news, and now more and more news comes not from news sites or newspapers, but from some kind of telegram channels. As a result, after some time, it turns out that you are subscribed to a dozen (and maybe dozens of channels) that constantly write something - as a result, either a huge amount of time is spent on "not missing something ". But if you look at it, most of them write about one thing, just differently. So the idea came to teach AI to select the news that really matters. Of course, there are different TOPs, like Yandex.News or something like the results of the day from some respected media outlet, but there are nuances everywhere. In this article I will try to describe these nuances and what we did and what we didn't.
Nuances and sources
, β , , , - β , " ". , ., , β , . β , .
:
-,
, , - (-, , )
β , , 100 . 10 . ( ) β . - . ,
100 , "" . , β , , - , GQ, .. - , , - .
, , , , , .. , - . , . β , ( )... - . , β , "" ( , , - ). , - , , .
:
" ", β
"", β .. ""
"" β .. - ,
, , :
( )
β NLP NER β ,
- ""
β 3 , : , ( ), β . , , - .
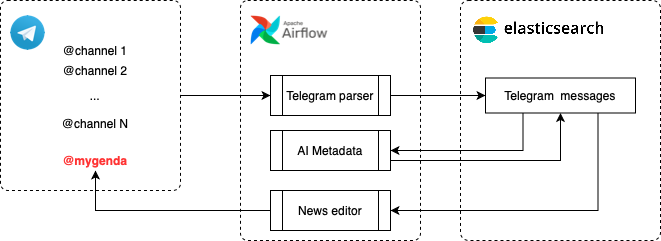
"" NLP
NLP, BERT, . . - . β , MVP :
. , NLP , β , .. - , . , , .
, BERT β , , , .
, MVP BERT , 20 β ( ), , BERT - , .. , . , , , β , ( ).
:
Spacy, , :
NLP , : NER, , ,
( BERT)
- , , Spacy.
, , : Natasha-spacy, , . , - , , .
, β , . β : . β , , . , NER PER, LOC, ORG , "" "" , .. .
- , , .. CONLLU, . :
-. - NER. . , . , - . β Β« Β», , . , ( CONLLU), CONLLU. , , .
"" "" β . , regexp-, .
, "" "". - :
, ,
, .. , "" "" , " "
β - , , , , 3- β
. , , .
, " ". Facebook( themeduza, forbesrussia) , , ria.ru. β - . ! - , , , . ~ .

, "" "" , , , , , .

, β . " " , - .
β , , . 4-5 , , , , - "".

4 GB RAM, 2 vCPUs 8% CPU, . , airflow, ( - airflow " " 16 GB RAM, 4 vCPUs 32%). , . , DAG-, β .

" X", . :
( , , , )
, β NER , "" , ( )
"source": {
"id": 1115468824,
"username": "lentadnya",
"title": " ",
"participants": 47148
},
"text": "Β«, , Β»: . , ",
"views": 405,
"link": "https://t.me/lentadnya/16263",
"interesting": 0.12,
"reaction": {
"enjoyment": 0.04400996118783951,
"sadness": 0.0019097710028290749,
"disgust": 0.8650462031364441,
"anger": 0.08112426102161407,
"fear": 0.00790974497795105
},
"entities": [
"",
" ",
"",
""
],
"tags": [
"",
" ",
"",
""
]
, .
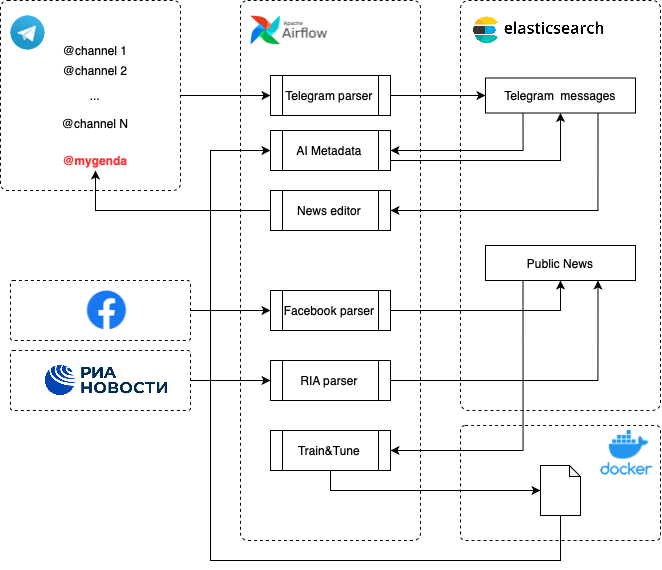
β1: "" , , . , β 3-4 , . .. , .. , . β .
β2: NER β . , , . . - 100 (, ) NER . 85%. . , BERT "" , - , -.
β3: , , , , , . . - "" . , "", . , ( ), NER " ". , , , . "" , - " " β . "" . , "" , , . .
Well, whoever has read up to this point, I hope it will be interesting, but what does our top look like: https://t.me/mygenda .
Well, as fashion bloggers say: subscribe, share comments and ask questions. I hope this was interesting.