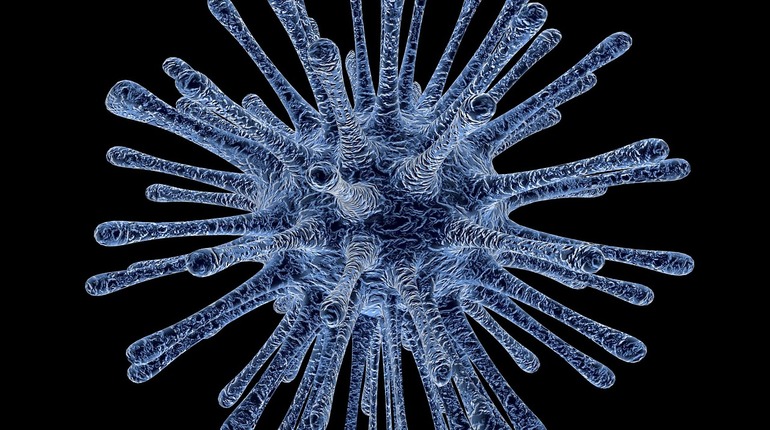
Bioinformatists have used an algorithm designed to simulate the human language to predict how viruses might evolve to defend against the immune system.
The copy is incorrect
Viruses lead a rather primitive cyclical existence. They penetrate the cell, perform a kind of hacking of its reproductive mechanisms, creating a copy machine for their own kind. Virus replicas spread throughout the body with the same purpose: to capture and subdue. And so on ad infinitum.
Quite often in the sequence of this copy-paste, something goes wrong - failures during copying give rise to mutations. Sometimes a mutation concerns the absence of some important protein or amino acid - such an unlucky virus is sent to the dustbin of evolutionary history. Sometimes a mutation does not affect anything at all: when the terms are rearranged in the sequence of proteins, the sum does not change.
But from time to time, the mutation works into the hands of the virus. The changes that have occurred not only do not prevent the virus from continuing to capture healthy cells, but also help it to do so with greater efficiency. Mutations can make the virus unrecognizable for a person's immune defense. Such an invader manages to evade the antibodies developed in people who have been ill or vaccinated, or to "slip away".
Scientists are always on the lookout for potential virus escape attempts. This is also true for SARS-CoV-2: new strains appear and scientists are investigating how critical these changes are for the existing vaccine (PS So far, everything is in order). The most difficult thing is for researchers of the influenza virus and HIV, which best of all "elude" the immune defenses of our body.
Virologists are trying to be ahead of the curve, so they create their own mutants in the laboratory and see if they can escape the antibodies taken from ex-patients and vaccinated. But this work is akin to looking for a needle in a haystack: the variations of mutations are so diverse that it is not possible to check everything. Such studies are conducted rather in order not to lose relative control over the situation.
Viral spelling
Last winter, Brian Hee, a bioinformatist at MIT and a big fan of John Donne 's poetry , pondered this problem and came up with an interesting analogy. What if we view viral sequences the same way we view written language? According to the scientist, each viral sequence has a kind of grammar - a set of rules that it must follow in order to be this particular virus.
If the mutation provokes a "grammatical error", the virus enters an evolutionary dead end. Just like language, viral sequence has a kind of semantics that the immune system can either read or not. If she reads it, then the immune system is able to understand the virus and stop it using antibodies or other means of protection. Continuing the analogy, viral "escape" can be seen as a change that follows the rules of grammar, but changes its semantics to one that is not read by immunity.
The analogy was not only beautiful, but also gave Brian Hee the idea of its practical application. Over the past few years, artificial intelligence has made great strides in the field of linguistics, correctly modeling the principles of grammar and semantics of the human language. Neural networks are trained on data sets consisting of billions of words and ordered by sentences and paragraphs, from which the system deduces patterns. As a result of training, AI algorithms "understand" how to correctly construct sentences and where to place commas. It can also be said that he “understands” the meaning of certain sequences of words and phrases and even takes into account the context - all this is based on correctly selected coefficients of the layers of the neural network.
The architecture of patterns for choosing a particular word is large-scale, and it is being prescribed in more and more detail. Thus, the most advanced natural language processing algorithms such as GPT-3 from OpenAI learn to create grammar-ideal texts while maintaining the style.
Both in literature and in biology
The main advantage of artificial intelligence algorithms is their scalability to different areas of science. For a machine learning model, a sequence is a sequence, no matter where it is in lyric sonnets or amino acids.
According to Jeremy Howard, an artificial intelligence researcher at the University of San Francisco and an expert on natural language processing algorithms, the use of AI algorithms in biological research can be beneficial.
Having a sufficient amount of data, for example, from the genetic sequences of infectious viruses known to science, the model can detect patterns and patterns in their structure.
"It will be an extremely complex model."Says Jeremy Howard. Brian Hee knew it too. His scientific advisor, mathematician and programmer Bonnie Berger, has previously done similar work with colleagues in the lab, using AI to predict protein folding patterns.
Language models for influenza, HIV and coronavirus
This spring, Berger's lab brought Brian Hee's idea to life. The research results are published in the journal Science . The team was initially interested in influenza and HIV, which are notorious for their masterful evasion of vaccines. But when they started the study in March 2020, the genome of the new coronavirus became available, so they decided to add that to the study as well.
For all three viruses, the scientists focused on the protein sequences they use to enter cells and replicate, explains Brian Bryson, a bioengineer, MIT professor and co-author of the study. These same sequences are the main target for the immune response and the key to creating an effective vaccine. Here, antibodies cling to the virus, preventing it from entering the cell and sentencing to destruction (for SARS-CoV-2, this is the S-spike protein.) For each virus, the MIT team trained a language model using genetic sequence data instead of the usual paragraphs and sentences.
After a while, scientists checked the result of training the model. According to the hypothesis of scientists, sequences that have similar semantics should infect the same "hosts". Thus, the genetic "language" of swine flu should be semantically similar to other swine flu and different from another subtype of influenza, for example, bird flu. The scientists' hypothesis was confirmed. In addition, they found that spread over time strains of influenza (for example, the 1918 and 2009 bird flu) were judged by the AI as semantically similar.
Then they turned to grammar. How correlated is the score of the virus for the "grammar" of the sequence and its viability in real life? Scientists have collected data from past studies evaluating the adaptability of mutated viruses (how well they attacked cells and replicated) for all three viruses. They then rated how grammatically correct these sequences were according to the model. The researchers assumed that a high score for this parameter meant a high adaptability of the virus.
Bryson and Hee also wanted to know if AI could predict the emergence of an "escape" virus. Then they compared the predictions of their model with the known cases of the actual "escape" of the virus. The influenza virus model was found to be the most predictive. Not surprisingly, the dataset they used to train this model was the most complete - it included flu sequences accumulated over several years, including surviving mutations.
As for SARS-CoV-2, scientists have tested their hypotheses on artificially bred mutants. The existing virus was repeatedly passed through the serum with antibodies until the virus mutated to a stage tolerant to them (we really have nothing to worry about yet). The success rate was lower here. The model singled out most of the true fugitives, but was sometimes wrong.
Nevertheless, the results obtained are a good starting point for further research by virologists who want to understand how the mechanism of natural mutation works. “This is a great way to narrow down the universe of potential mutant viruses ,” comments Benhour Lee, a microbiologist at Icahn School of Medicine on Mount Sinai.
The scientist added that predictions are as good as the data on which the model is trained. It should also be borne in mind that the AI model misses some nuances, because the escape property is not always a consequence of mutation. HIV is proof of that. Sometimes the sequence of this virus does not change, and its proteins are even recognized by antibodies, but they are well protected by a polysaccharide called glycan.
Benhour Lee noted that AI predictions primarily help researchers confirm existing knowledge. So, the model correctly identified two parts of the SARS-CoV-2 spike protein, which, as it was found earlier, are more susceptible to mutations, and a region of the virus sequence that is stable, which means it is a good target for antibodies.
Time will tell what other discoveries the forecasts of the AI model will lead to. So far, scientists have pinned special hopes on it in terms of identifying the so-called combinative mutations, which include many changes superimposed on each other.
The next step, which Bryson's staff will take, will be to create in the laboratory some of the predicted mutants of SARS-CoV-2 and monitor their response to antibodies taken from the ill and vaccinated people. They will also test several sequences collected from attempts to sequence virus samples from patients with Covid-19, which the model believes are more prone to escaping, Bryson said.
Scientists also want to test whether their analogy applies to other situations. Could a similar model predict whether the immune system will become intolerant of a particular cancer treatment or whether cancer cells might mutate and stop responding to treatment? With enough data in hand, Bryson's lab wants to test that too.
