Hello everyone! My name is Natalya Bessonova, I am the project director of the digital identity block of Rostelecom. In this article I want to talk about how we chose passive liveness from one face image for the Unified Biometric System.
There are not many articles on Habrรฉ devoted to liveness in biometric systems, and those that exist describe methods and algorithms for determining liveness, or, more simply, the belonging of biometric samples to a living person. Let's pay tribute to their authors - the articles are interesting and informative, but what if we are interested in how well they work on data that is not included in any of the open data sets? How do you know if the liveness is really good or bad and which one should you choose if necessary? In this article, I will describe an approach to choosing a passive singleshot-liveness (i.e., one image at a time) of a face for the Unified Biometric System .

Why do you need liveness
, ( , ). . , , ( , ):
O , ยซยป Deepfake /, / . , . - ยซยป , . - โ . .
โ : , , ?
. Deepfake Detection Challenge , Amazon, Facebook Microsoft. NtechLab 2,2 .
, โ . . , 5-10-20 . . . . , โ . โ .
. - . - , , โฆ ( ) : ?
, . , , .
, , โ , . .
โ :
, , . .
, - . : , 3D .
, , -, .
. , , . , , COVID-19, . : , , , , . , , . . .
, : ยซ ?ยป ยซ ?ยป. ( ), , , , ยป , . , .
: (-) . : , , , , .., , , . , , - .
, , . , , deepfake. , , , .
2020 58624, , . . , :
;
;
( , ).
. , , . 58624.3, :
, ;
, ; () , . ;
, ;
, .
, . , . , . .
.
, -,
, .
FIDO Biometrics , , . , , . , , , .
:
โ ;
2D- โ ;
โ , , ;
โ ;
3D- โ , 3D-.
, . , singleshot- deepfake , , , .
.

300 ( ), 1%. - :
Logitech C270;
Logitech C930;
Logitech B920;
Logitech Brio;
Intel Realsense D415;
Xiaomi Redmi6.
, , , .
, .
, :
1) CPU - Intel(R) Core(TM) i5-6600 CPU (4 , 3.30 , AVX AVX2 );
2) GPU - Nvidia GeForce GTX 1070 (8 );
3) - 16 DDR4;
4) - SSD SAMSUNG 970 EVO 500.
12 . , , singleshot-, API RGB , liveness score, . 3D- Intel RealSense D415 , , RGB , liveness score. .
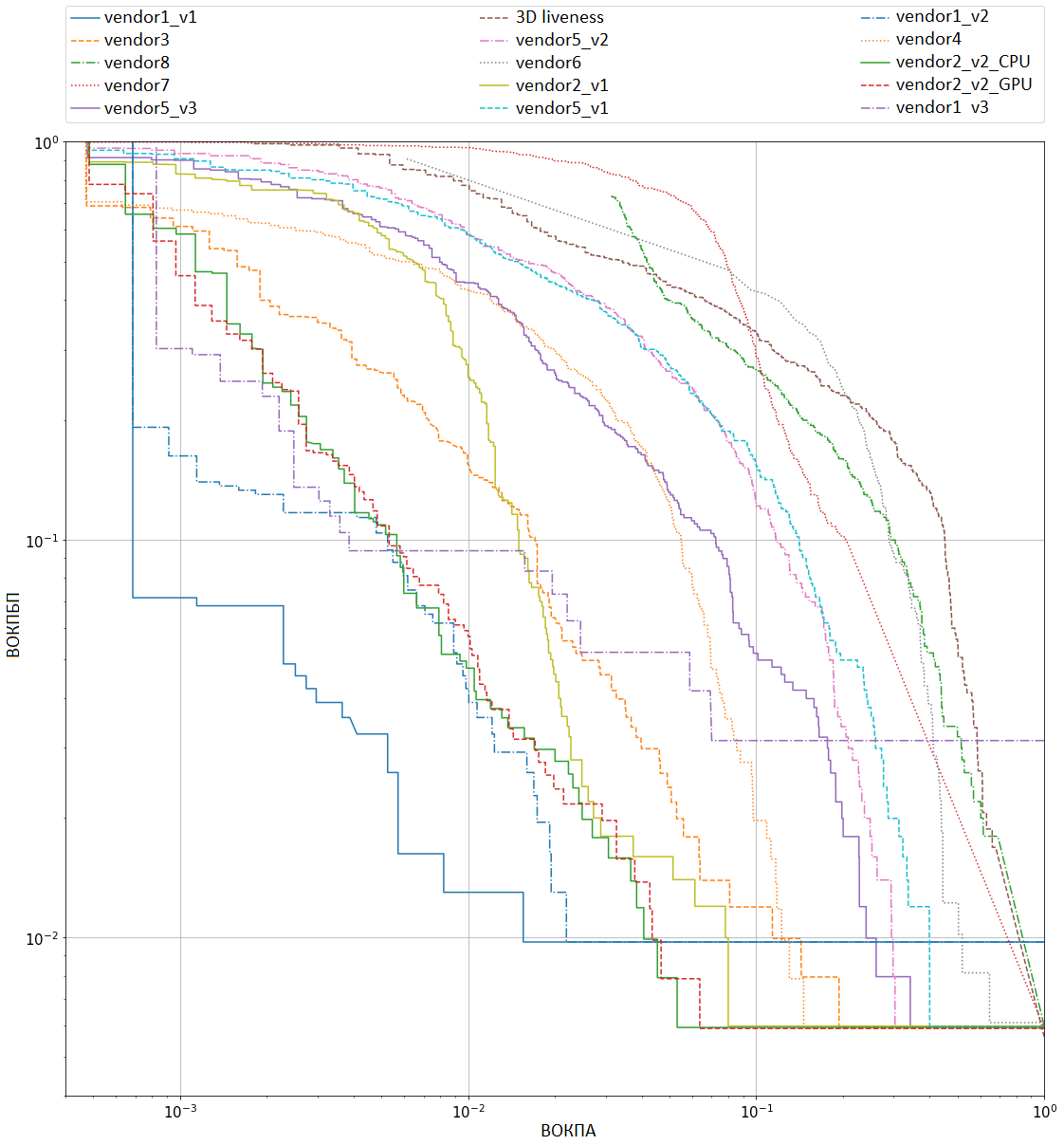
|
@0.01 |
, |
_, |
|
, |
_, |
|
vendor1_v1 |
0.013 |
266.501 |
160.091 |
0.395 |
542.772 |
471.081 |
0.320 |
vendor1_v2 |
0.042 |
155.626 |
108.508 |
0.395 |
381.548 |
367.438 |
0.320 |
vendor2_v2_CPU |
0.048 |
874.527 |
21.748 |
0.008 |
910.202 |
60.381 |
0.039 |
vendor2_v2_GPU |
0.057 |
863.651 |
26.629 |
0.006 |
903.743 |
62.957 |
0.037 |
vendor1_v3 |
0.094 |
110.198 |
100.997 |
0.811 |
293.092 |
242.255 |
0.437 |
vendor3 |
0.153 |
146.217 |
82.217 |
0.012 |
360.056 |
324.149 |
0.016 |
vendor2_v1 |
0.255 |
874.294 |
35.319 |
0.002 |
1060.088 |
121.127 |
0.036 |
vendor4 |
0.423 |
444.085 |
35.818 |
0.006 |
512.427 |
106.987 |
0.010 |
vendor5_v3 |
0.442 |
160.426 |
298.351 |
0.014 |
683.258 |
882.690 |
0.025 |
vendor6 |
0.477 |
73.560 |
111.626 |
0.035 |
433.866 |
527.738 |
0.044 |
vendor5_v2 |
0.584 |
165.370 |
310.281 |
0.014 |
720.696 |
891.637 |
0.025 |
vendor5_v1 |
0.586 |
200.883 |
373.670 |
0.014 |
767.726 |
997.728 |
0.025 |
3D liveness |
0.777 |
|
|
|
|
|
|
vendor7 |
0.967 |
3021.262 |
46.769 |
0.006 |
3105.798 |
165.991 |
0.007 |
vendor8 |
1.000 |
754.956 |
155.772 |
0.002 |
1618.374 |
1789.670 |
0.006 |
, . : 1,3% 1%. : , 3D-, , .
. , . , . , , , 9% , , , .
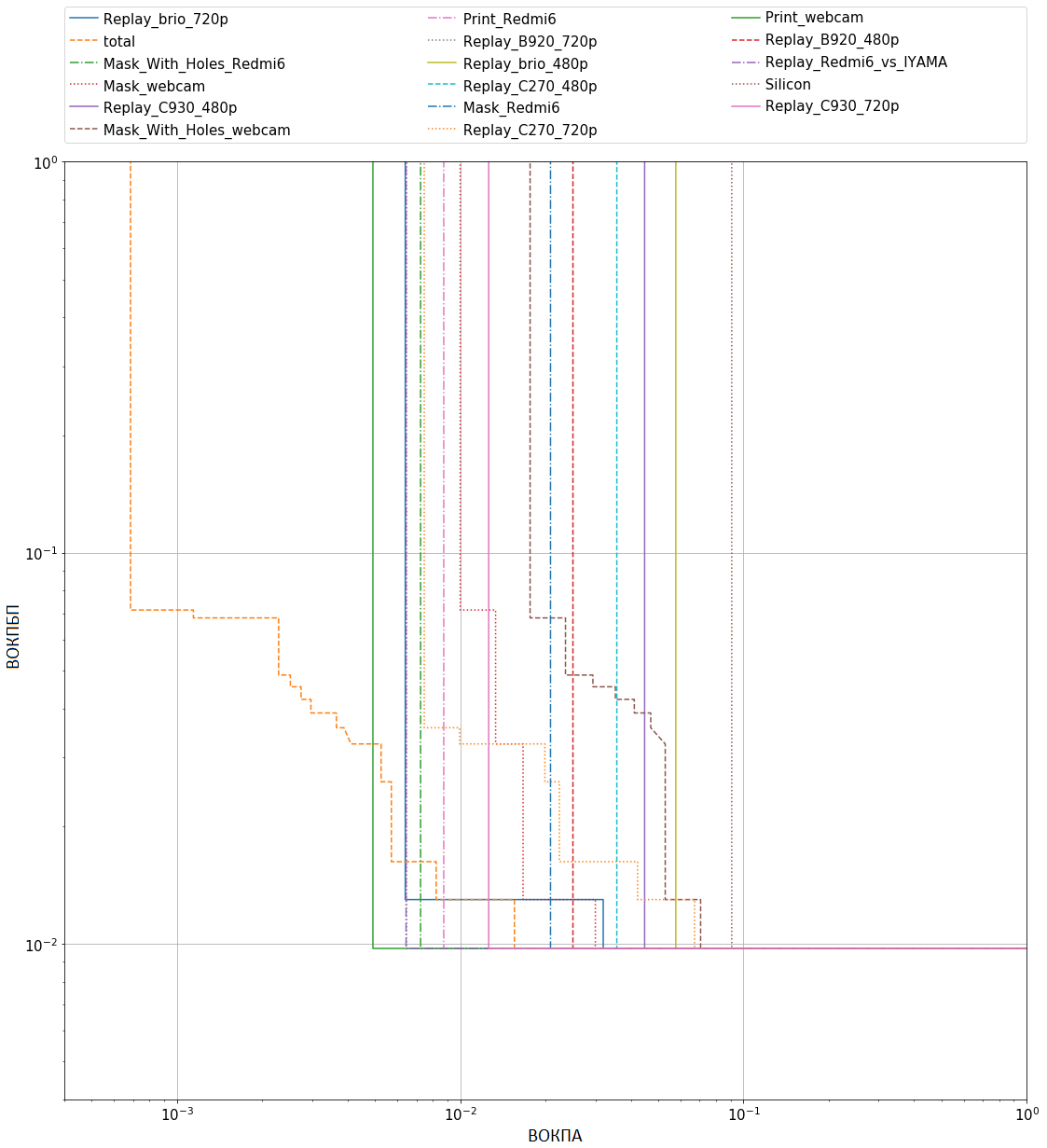
. - . , , :
;
;
.
, โ , , , , . , , . , , , .
, .