OneSoil develops free apps for farmers that are used in more than 180 countries around the world. In our work, we use big data and machine learning, and a separate quest for us is to find ground truth data. Here's how we solve this non-trivial task.
Why OneSoil Machine Learning? In order to determine the boundaries of fields, crops, phenological stages, yield, sowing dates and harvest dates using satellite images. All this is either already in the OneSoil applications, or will appear there in the near future.
Let's look at the example of determining the boundaries of fields using satellite images. For a farmer, delineating the boundaries of his field is the very first step in the process of digitalizing his farm. This is the cornerstone without which no other application work is possible. And the task is not so simple: before, farmers solved it due to the fact that they traveled around their fields with GPS trackers on ATVs, struggled with orthophotomaps, in short, it was expensive and long. OneSoil, on the other hand, learned to recognize field boundaries using satellite images: you open the application, press the "add fields" button, select your own on the map with recognized fields - and that's it.
How did we do it? At first, we had data from only a few farms in Belarus and the Baltics, using which machine learning algorithms learned to predict field boundaries. It worked like this: for each real field (the boundaries of which we knew thanks to the farms), we calculated the area of ββcoincidence with the boundaries that the algorithms predicted. If the algorithm circled the extra sections, it received a fine for this. So he studied. This indicator is called intersection over union, it can take values ββfrom 0 to 1, where 1 is a perfect match. In our country, this indicator varies from region to region, but on average is 0.85β0.88.
Then we began to show the neural network millions of images of agricultural fields in order for it to learn how to determine where the field is and where not. The algorithm takes a long time to learn, we look at the results and improve it many times until the accuracy of determining the field boundaries for a particular region becomes good. How do we know that accuracy has improved? Again, we compare our calculations with real data on the fields. Now there are 57 countries in which we are good at defining field boundaries.

When we confidently determine the fields, say, in Ukraine, this does not mean that everything will work the same somewhere in Brazil - after all, there are their own fields and their own agricultural characteristics. Therefore, we need real data again to refine and improve our algorithm.
. , . ? .
OneSoil , , , . β R&D .
, . . (, ), , . , . , , . , . , . OneSoil , .
133 | 2,8 β , OneSoil. 2020 .
R&D , , . .
OneSoil Map 2018 (Guido Lemoine), Joint Research Center (JRC). (ESA) Data Science . Β« , - , β . β , Β». R&D , JRC .
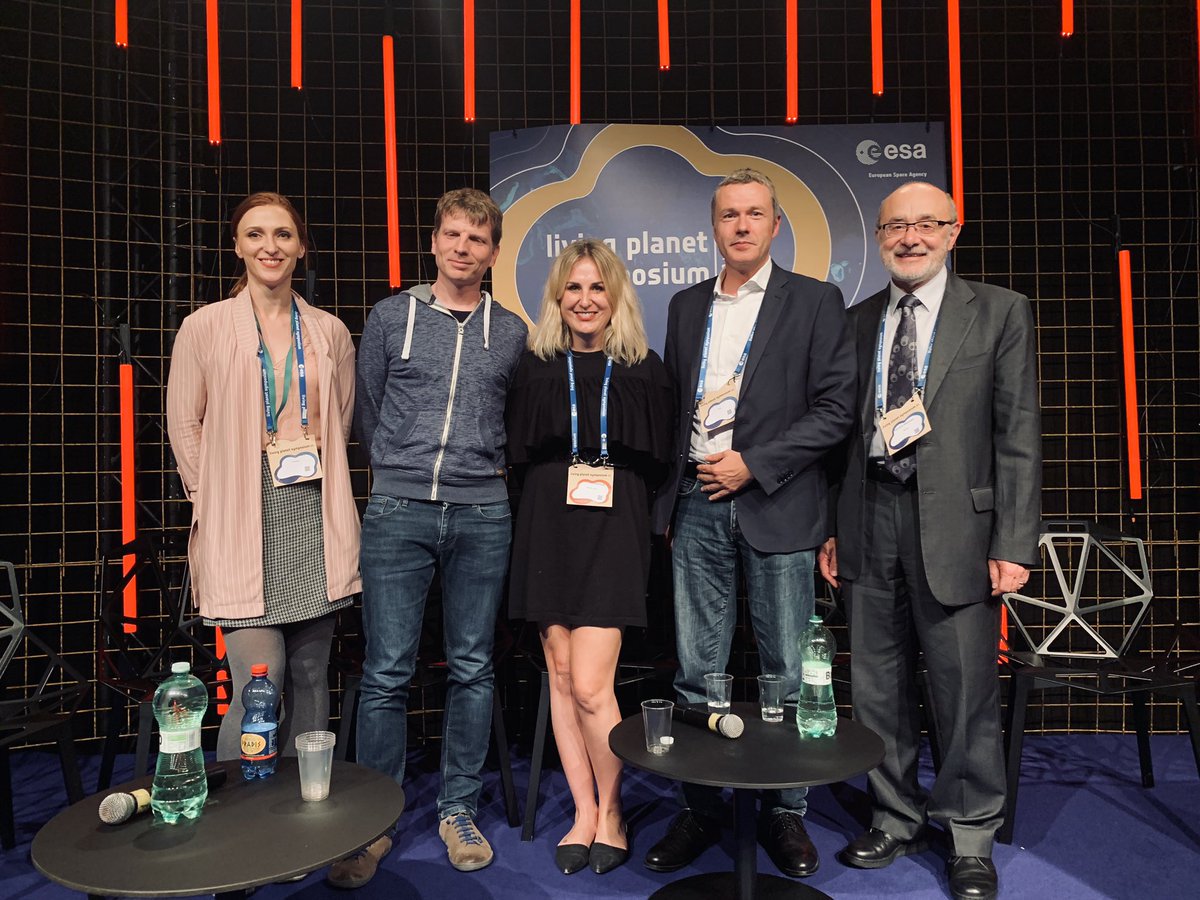
OneSoil β . , , . , , .
4 7 . , , β R&D. 2β3 . β . Β« 2020 100 Β» β .
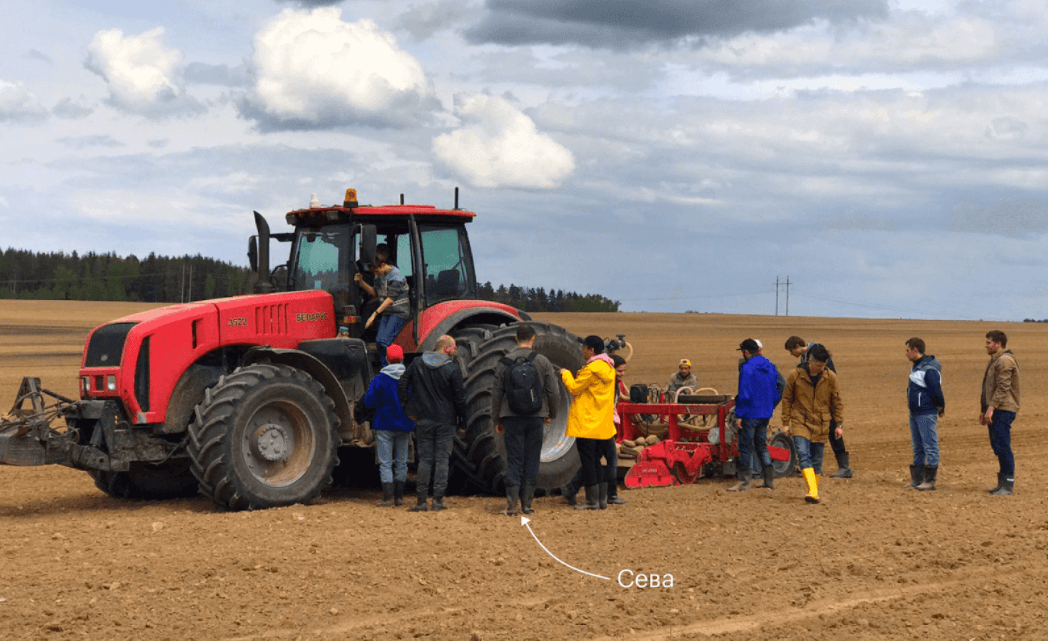
2018 CEO . , . , : Β« Β». .
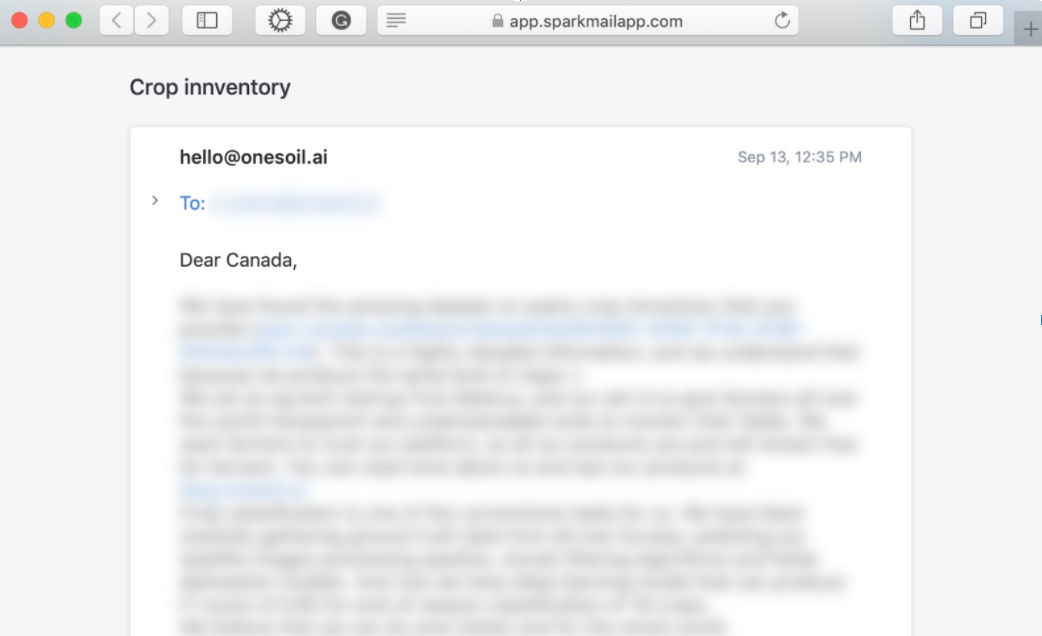
, . . 50 , OneSoil .
392 | 126 β ground truth . 2020 .
When we have a lot of data from open sources and from different partners, we improve our algorithms that we already use in OneSoil applications (or will be in the near future). When we have a lot of data from users, we again improve the accuracy of our calculations. This is how data and technology work for each other.