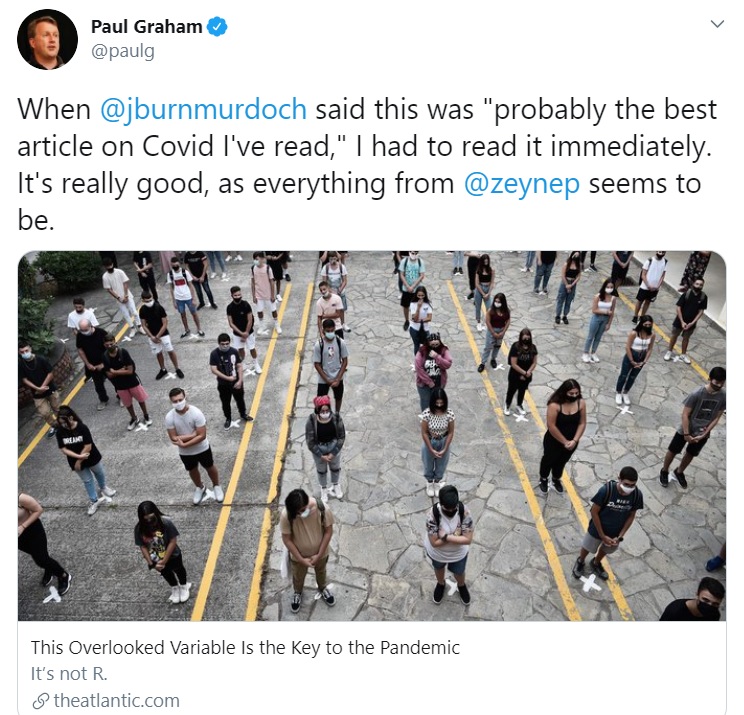
There is something strange about this coronavirus pandemic. Even after several months of extensive research carried out by the world scientific community, many questions remain open. Why, for example, in the northern part of Italy there were so many more deaths than in other parts of the country? 25,000 deaths (out of 36,000) occurred in just three contiguous regions in northern Italy. In Lombardy, about 17,000 people died. Almost all of these deaths occurred in the first months after the outbreak. What happened in April in Guayaquil, Ecuador, when a large number of people died so quickly that their bodies were thrown on the sidewalks and streets? Why did such a small number of cities account for a significant proportion of global deaths in the spring of 2020, while many other cities with similar density, weather, age distribution and travel patterns escaped this fate? What can we really learn from Sweden, which is simultaneously called both a great success (due to a low number of cases and deaths) and a failure (due to high mortality at the beginning of the pandemic), despite the fact that a second wave is already beginning in Europe? Why did the previously widely discussed forecasts of the Japanese authorities not come true? There are more and more such strange examples.
Over the past nine months, I've heard many explanations for differences in pathways - weather, elderly populations, vitamin D, previous immunity, herd immunity - none of them explain the period or scale of these fluctuations.
However, there may be an overlooked key to understanding the pandemic that would help answer these questions, reconsider current arguments and, most importantly, control the spread of COVID-19.
By now, many have already heard about R0 - the basic reproductive number of a pathogen, which makes it possible to assess its infectivity. But if you haven't read scientific journals, you most likely have not come across k - the measure of its variance. Determining k seems tricky, but it's basically just a way to figure out how a virus spreads - evenly or in large outbreaks, in which one person infects many other people at once. After nine months of collecting epidemiological data, we know we are dealing with an extremely widely dispersed pathogen, which means that it tends to spread in clusters. This knowledge has not yet fully entered our understanding of the pandemic (and our prevention practice).
The renowned R0 coefficient (pronounced "er-zero") is the average infectivitypathogen, that is, the average number of people who can become infected after contact with an infected person. If one sick person infects, on average, three others, then R0 is three. This parameter was considered as one of the key factors in understanding how the pandemic works. The news media has produced many explanations and visualizations of this parameter. The films, praised for their scientific credibility with regard to the pandemic, deserve praise for their characters explaining the "extremely important" R0. Appeared online tabletracking the value of R and Rt in real time in accordance with our actions (If people wear masks and are isolated, or immunity appears, the disease can no longer spread at the same rate, hence the difference between R0 and R).
Unfortunately, averages do not always help assess the entire distribution, especially if its elements may differ significantly from each other. If Amazon CEO Jeff Bezos walks into a bar with 100 patrons, the bar's average welfare will suddenly surpass $ 1 billion. If I enter this bar too, little will change. Obviously, the average is not very helpful in understanding the wealth distribution in this bar or how to change it. Sometimes the mean doesn't say anything. At the same time, if there is a person infected with COVID-19 in a bar, and there is poor ventilation and noise in the room (because of which people will have to speak loudly at short distances), then almost all guests can be infected - a similar pattern has been observed many times since the start of a pandemic,and it is simply not taken into account by the indicator R. Here we come to the concept of variance.
There are situations in which one person can infect 80% or more of the people in the room in just a few hours. At the same time, in other cases, COVID-19 may be surprisingly less contagious . The overdispersion and super-spread of this virus has been observed in studies around the world. A growing body of research estimates that most infected people may not infect any other person. In recent workin Hong Kong, where extensive testing and contact tracing has been carried out, it was found that about 19% of infections were responsible for 80% of transmission, while 69% of cases were not transmitted to any person. And this finding is not anomalous: many researchers assumed from the outset that only 10 to 20 percent of infected people could be responsible for 80 to 90 percent of transmission, and that many people do not transmit the disease at all .
This highly skewed and uneven distribution suggests that a series of unsuccessful events at the very beginning of spread can lead to super-spread of infection and the emergence of clusters - and that is why events in even similar countries can be radically different. Scientists looked at early events from a global perspective , when infected people traveled to different countries, and found that in some countries this did not lead to death or the spread of infection, while in others there were significant outbreaks. Using genomic analysis, researchers in New Zealand looked at more than half of the country's confirmed cases and found a staggering 277 infections in the first months, and only 19% of them resulted into at least one additional infection. Recent analysis suggests that a similar effect can occur even in crowded areas (such as nursing homes), and that it is possible that several widespread spreads are necessary for an outbreak to occur . Meanwhile, in Daegu, South Korea, only one woman, named “Patient 31,” has caused more than 5,000 known infections in a single cluster - the megachurch.
Unsurprisingly, SARS-CoV, the previous generation of the SARS-CoV-2 virus that caused the SARS outbreak in 2003, was also super-spread in this way.: Most of the infected people did not transmit it, but most outbreaks were triggered by multiple events that led to super-spread. MERS, another cousin of the SARS coronavirus, also seems to be super-common , but luckily it doesn't transmit very well in humans.
Indicator k takes into account exactly a similar pattern of alternation of levels of infectivity - what escapes the eye of indicator R... Samuel Scarpino, Assistant Professor in the Department of Epidemiology and Complex Systems of the Northeast Region, told me that this was a huge problem, especially for health authorities in Western countries, where pandemic guidelines focused on influenza - and it made sense because the influenza pandemic can be incredibly dangerous. However , influenza does not have such a clustering distribution .
We can view the picture of the disease as deterministic or stochastic: in the first case, the outbreak propagation is more linear and predictable, in the second, chance plays a much larger role, and it is difficult (if not impossible) to make predictions. In deterministic trajectories, we expect that what happened yesterday will give us a good idea of what to expect tomorrow. Stochastic phenomena, however, do not work this way: the same inputs do not always give the same outputs, and phenomena can quickly transition from one state to another. As Scarpino told me, "Diseases like the flu are pretty close to deterministic, and R0 (albeit imperfect) paints the right picture (it's almost impossible to stop the spread until a vaccine is available)." This statement is not necessarily true for super common diseases.
Nature and society are rife with imbalanced phenomena, some of which operate on the Pareto principle, named after the sociologist Wilfredo Pareto. The Pareto idea is sometimes called the 80/20 principle - 80 percent of the results of interest are due to 20 percent of the contributions - although the numbers don't have to be that strict. Rather, the Pareto principle means that a small number of events or people are responsible for most consequences. This should come as no surprise to those who have worked, for example, in the service industry, where a small group of problem clients can cause all the additional workload. In such cases, excluding these customers from the business or giving them a large discount can solve the problem, but if complaints are evenly distributed, then a variety of strategies will be required. The same way,focusing on the R score or leveraging the experience of an influenza pandemic will not necessarily work well in an overly diffuse pandemic.
Hitoshi Oshitani, member of the National COVID-19 Cluster Research Group under the Japanese Ministry of Health, Labor and Welfare and a professor at Tohoku University, who told me that Japan initially focused on over-proliferation, compares his country's approach to looking at the forest and tries to find clusters , not trees... He believes that the Western world is staring at the trees and is lost among them. To effectively tackle the super-spread of the disease, politicians must understand why it is happening and they must understand how it affects everything - including our contact tracing methods and testing policies.
The super-spread of a pathogen can have many reasons . Yellow fever spreads mainly through the Aedes aegypti mosquito, but until the insect's role was discovered, its transmission drove many scientists crazy. Tuberculosis was thought to spread with droplets until it was proven through clever experimentsthat it is transmitted through the air. Much is still unknown about the SARS-CoV-2 super-spread. It is possible that some people are super-distributors of the virus - that is, they spread it much more than others. As with other illnesses, contact patterns certainly play a role: a politician on the campaign trail or a student in a dorm differs significantly in the number of contacts with other people compared to, for example, an elderly person living in a small house. However, through the analysis of epidemiological data accumulated over nine months, we have important information about a number of factors.
Many studies show that in the vast majority of cases, clusters of the spread of COVID-19 occur in poorly ventilated areas where large numbers of people gather: weddings, churches, choirs, gyms, funerals, restaurants, that is, places where people speak loudly or sing without masks. For super-proliferation to occur, a number of other aspects need to be manifested, and the risks of their occurrence may differ from place to place and from activity to activity, according to Müge Cevik, instructor of courses in infectious diseases and medical virology at the University of St Andrews and co-author of the recent extensive research on the conditions of transmission of COVID-19 .
Cevik argues that "prolonged exposure, poor ventilation, [and] crowds" are key factors in super-spread. Super-proliferation can also occur in rooms outside the one and a half meters directive, as SARS-CoV-2, the pathogen that causes COVID-19, can travel through the air and accumulate, especially if the room is poorly ventilated. Given that some people infect others before symptoms develop (and sometimes symptoms are very mild or absent), we cannot even know if we ourselves are contagious. We don't even know if there are other factors that contribute to super-spread. However, we don't need to know all these factors to avoid the necessary super-spread condition: crowds, especially in a poorly ventilated room, and (even more especially) not wearing masks.As Natalie Dean, a biostatist at the University of Florida, told me, given the huge numbers associated with these clusters, targeting them to eradicate them could significantly reduce infection rates.
Excessive scattering should be considered when working on contact tracing. We may need to do the opposite. Currently, many states are engaged in what is called direct (or prospective) contact tracing. Once an infected person is identified, we try to figure out who he interacted with after that so that we can alert, check, isolate and quarantine the people involved in this interaction. But this is not the only way to track contacts. Due to excessive variance, this technique is not always beneficial. Instead, in many cases, we should try to work in the opposite direction to see who first infected the subject.
Due to over-dispersion, most people will be infected by a person who has also infected other people - as statistically only a small percentage of those infected infect a large number of people at the same time. Most infects either one person or no one at all. As Adam Kucharski, an epidemiologist and author of Infection Rules , explained to me , if we can use retrospective contact tracing to locate the person who infected our patient, and then directly trace the infected person's contacts, we usually find many more casescompared to the simple application of direct contact analysis - it will simply indicate a number of potential infections, many of which will remain potential because many chains of distribution do not work.
The reason why backtracking is important is similar to what sociologist Scott L. Feld called the friendship paradox: Your friends will, on average, have more friends than you. (Sorry!) This is obvious when you look at the web view. Friendships are unevenly distributed; Some people have a lot of friends, and your circle of friends is likely to include these social butterflies, because it couldn't be otherwise. They have made friends with you and with other people. And these they increase the average number of friends that your friends have compared to you, the average person. (Of course, this does not apply to social butterflies themselves, but excessive dispersion means that there are much fewer of them.) Likewise, an infectious person transmitting disease is like a pandemic social butterfly: on average, they infect many more people than the average person, who will transmit infection much less frequently. Indeed, howKukharsky et al. argue mathematically, overdispersion means "that direct tracking alone can reveal at most an average number of subsequent infections (ie, R)." In turn,
"backtracking increases this maximum number of tracked persons by 2-3 times, since the cases under consideration are more likely to come from existing clusters rather than create new ones"
Even in an overly diffuse pandemic, it makes sense to do direct tracking to be able to warn and validate people - if there are additional resources and testing capabilities. But it doesn't make sense to do direct tracking without allocating enough resources to track back and find the clusters that are doing so much harm.
Another significant consequence of over-scattering is that it emphasizes the importance of performing certain types of fast, cheap tests. Consider the current dominant testing and tracking model. In many countries, health authorities are trying to trace and find direct contacts of an infected person: everyone with whom he has been in contact since the moment of infection. They then try to test them all with expensive, slow but highly accurate PCR (polymerase chain reaction) tests. In a situation where clusters affect the spread of infection so strongly, this may not be the best strategy.
PCR tests identify segments of coronavirus RNA in nasal swab samples - as if looking for its signature. Such diagnostic tests are assessed on two things: Do they do a good job of detecting non-infected people (specificity), and do they do a good job of detecting those who are infected (sensitivity)? PCR tests are very accurate on both parameters. However, PCR tests are also slow and expensive, and require a deep and uncomfortable smear performed in a medical facility. Also, due to the long processing time, people sometimes do not receive information when they need it. Worse, PCR tests are so responsive that they can find tiny remnants of coronavirus signatures long after someone is no longer infectious , which could lead to unnecessary quarantines.
Meanwhile,Researchers have demonstrated that rapid tests (which are very accurate at identifying people without the disease, but not so good at identifying infected individuals) can help us contain this pandemic. As Dylan Morris, Ph.D. in ecology and evolutionary biology at Princeton, told me, cheap, low sensitivity tests can help contain a pandemic, even if it is not overly diffuse - but they are especially valuable for identifying clusters within an overdispersed pandemic. This is especially helpful because some of these tests can be performed using saliva and other less invasive methods, and can also be distributed outside of healthcare settings.
In an overly dispersed regime, identifying infections is more important than identifying infected individuals. Consider an infected person and 20 direct contacts - people he met after infection. Let's say we test 10 of them with a cheap quick test and get the results in an hour or two. This is not the best way to determine which of these 10 is sick, because our test will miss some of the positive results, but for our purposes this is fine. If they are all negative, we can act as if no one is infected, because the test finds negative results pretty well.
However, the moment we find several cases of infection, we know that perhaps we are dealing with a super-spread, and we can tell all 20 people that they are most likely infected and should isolate themselves. If we find one or two infections, then the matter is most likely in cluster distribution. Depending on age and other factors, we can test these people individually using PCR tests that can pinpoint who is infected - or ask them all to wait.
Scarpino told me that overdispersion also enhances the usefulness of other methods - such as testing wastewater (especially in crowded places like dorms or nursing homes), allowing us to detect clusters without testing absolutely everyone. Wastewater research also haslow sensitivity , it can miss positive results if too few people are infected, but this is normal for screening. If testing wastewater signals that there is most likely no infection, then we do not need to test everyone to identify all potential cases of infection. However, as soon as we detect the signs of a cluster, we can quickly isolate everyone - again, pending further individualized testing using PCR tests , depending on the situation.
Unfortunately, until recently, many of these cheap tests have been restricted by regulatory agencies in the United States. In part because they were concerned about their relative inaccuracy in detecting positive cases compared to PCR tests - this concern has overlooked their usefulness at the population level for this particular over-diffused pathogen.
Returning to the mysteries of this pandemic, why did such different propagation trajectories appear in the early stages in similar countries? Why didn't the usual analytical tools (case studies, country comparisons) give us more accurate answers? Intellectually, this is not satisfying, but due to excessive variance and randomness, there may be no explanation - except that in the most affected regions, there were several outbreaks of super-spread at the initial stage. It's not just luck: population density, elderly populations and crowds, for example, make cities around the world more susceptible to outbreaks compared to rural areas, places with low population density, and cities with younger populations with fewer populations. mass transit or healthier citizens.But why in February the outbreak occurred in Daegu and not in Seoul - despite the fact that the two cities are in the same country, under the same government, they have similar people, they have about the same weather and much more? As distressing as it is, sometimes the answer can only be where the 31st patient ended up and the megachurch she attended.
Over-variance makes us hard to learn the lessons the world teaches us because we fail to think about cause and effect. For example, this suggests that the events that lead to the spread and non-spread of the virus are asymmetric in terms of what we can learn. Take a look at the replicated casein Springfield, Missouri, in which two infected barbers (both wearing masks) continued to work with clients with symptoms of infection. It turned out that there were no clear cases of infection among 139 clients (67 were directly examined; the rest did not report that they were sick). While there is ample evidence that masks are critical to attenuating transmission, this event alone does not tell us if masks are working. In contrast, studying transmission, a rarer event, can be highly informative. If these two hairdressers transmitted the virus to a large number of people, despite everyone wearing masks, it would be important evidence that masks may not help prevent super-spread.
Comparisons also give us less information than phenomena in which input and output are more closely related to each other. We can check for a factor (say, sunlight or vitamin D) and see if it correlates with the effects (degree of infection). But everything is much more complicated if the consequences can vary greatly depending on several random events - in mid-February in South Korea, the wrong person was in the wrong place . This is one of the reasons that when comparing multiple countries, it is difficult to identify dynamics that explain the difference between trajectories at different locations .
Once we recognize that super-proliferation is a major factor, countries that look too relaxed in some respects will look very different, and our usual polar debates over the pandemic will be discounted as well. Take Sweden, for example, which, depending on who you ask, has had great success or failed horribly with herd immunity without a lockdown. In fact, while Sweden has become one of many countries that has failed to protect older people in their communities, its anti-proliferation measures have been stricter than many other European countries. Despite the fact that Sweden did not go full lockdown, as Kuharsky told me,in March Sweden imposed a restriction on mass gatherings - no more than 50 people. This restriction has not yet been lifted, despite the fact that many European countriesweakened their measures after the end of the first wave (many return to tightening measures due to the worsening of the situation). In addition, compared to most European countries, Sweden has fewer multi-generational homes, further limiting transmission and clustering opportunities. Schools in Sweden remained completely open without social distancing and masking, but only for children under 16, who are unlikely to be super-spreading the disease. Both the risk of transmission and the risk of illness increase with age, and Sweden has moved high school and college students online - the opposite of what it has done in the United States. Also in Sweden, social distancing was encouraged, and institutions that did not follow the rulesclosed . In terms of over-variance and super-proliferation, Sweden is not among the softest (in terms of measures) countries, but it is not the most stringent either. You just don't need to refer to it so often in discussions and evaluations of various strategies.
While over-variance makes it difficult to apply conventional methods of studying causality, it is possible to study errors in understanding what conditions turn bad luck into disaster. We can also study lasting success, because bad luck will eventually hit everyone, and the response to it is important.
The most informative examples may well be those who initially, like South Korea, were terribly unlucky but managed to achieve significant containment. In contrast, Europe, on the other hand, was praised for opening early, even though this was premature; many countries are now experiencing widespread increases in cases, and in some respects these countries are similar to the United States. In fact, Europe's success this summer and relaxation (including allowing large-scale private events) is instructive about another important aspect of managing an over-dispersed pathogen: compared to a more resilient system, success under a stochastic scenario can be more fragile than it seems.
Once there are too many outbreaks in a country, the pandemic seems to go into a “flu mode,” as Scarpino put it, which means a high, sustained level of infection at the community level - even though most infected people may not transmit the infection. further. Scarpino explained that if not really drastic measures are taken, then after COVID-19 enters this regime, it could continue to spread due to the huge number of existing chains. In addition, huge numbers could eventually lead to the emergence of new clusters, further aggravating the situation.
The relatively quiet period could hide how quickly things can escalate into large outbreaks, and how several related amplification events can quickly turn a seemingly uncontrollable situation into a disaster, Kukharsky said. We are often told that if Rt, the measure of the real-time average spread, is above one, then the pandemic will escalate, and if below one, then it will decline. This may be true for an epidemic that is not overly diffuse, and if Rt is less than one (which is certainly a good thing), don't relax too much, as a chain of random events can again lead to huge numbers. No country should forget the South Korean patient 31.
However, over-dispersion is also cause for hope, as shown by South Korea's aggressive and successful response to the outbreak - with massive testing, contact tracing and isolation. Since then, South Korea has also shown continued vigilance and demonstrates the importance of backtracking . When a series of nightclub-related clusters erupted in Seoul recently, health authorities aggressively tracked and tested tens of thousands of people associated with these places - regardless of their interactions and social distancing. This is a reasonable measure, given that we know the pathogen is transmitted by airborne droplets.
Probably one of the most interesting cases was Japan, a country with average luck that got hit early and took measures that seemed out of the ordinary - did not conduct mass testing, did not go to a full lockdown. By the end of March, influential economists were issuing dire-warning reports predicting hospital system congestion and huge spikes in deaths . However, the predicted disaster never materialized, and although the country faced several waves, it did not have large spikes in mortality despite an aging population, continuous use of public transport, urban density and lack of a formal lockdown.
It is not that in the beginning the situation in Japan was better than in the United States. Oshitani said that Japan, like the US and Europe, did not have the ability to conduct mass PCR testing. The authorities also could not impose a full lockdown or regulate the order of stay at home, even if it was desirable - in Japan this is not legally possible.
Oshitani told me that Japan had noticed over-dispersion in the spread of COVID-19 in February, and so they developed a strategy focused mainly on destroying clusters, trying to prevent one cluster from being “set on fire” by another. Oshitani said that, in his opinion, "the distribution chain cannot be sustainable without a chain of clusters or megaclusters." Thus, Japan has implemented an approach that has applied aggressive tracing back to detect clusters... Japan has also focused on ventilation, advising its population to stay out of confined spaces where crowds gather and interact closely, especially if they are talking or singing. Thus, in Japan, scientific knowledge of overdispersion was combined with information on airborne transmission, as well as pre-symptomatic and asymptomatic transmission.
Oshitani opposes the Japanese strategy (within which all the important features of the pandemic in its early stages were worked out) with the western one, in which they seek to eliminate cases of infection one by one- although this may not be the main distribution method. In fact, cases in Japan have dropped, but vigilance has not been lowered: When the government began to notice a rise in community cases, it declared a state of emergency in April and struggled to get businesses that might be related to shut down. with super spread cases. We are talking about theaters, music venues, stadiums. Now schools are returning to face-to-face studies, and even stadiums are open - but chanting is prohibited .
It's not always about the severity of the rules and restrictions, but whether they are aimed at the right source of danger. As Morris said, "Japan's commitment to cluster destruction has allowed it to achieve impressive results with judiciously chosen constraints." Countries that ignored super-proliferation risked the worst of both worlds: burdensome restrictions that failed to translate into significant mitigation. The UK's recent decision to restrict outdoor gatherings to six people and allow pubs and bars to remain open is just one example of many. ”
Can we get back to normal by focusing on limiting the conditions for super-spreading events by aggressively burning clusters anddeploying cheap, fast mass tests (once we get our numbers low enough to carry out such a strategy)? Many countries with low rates of pathogen transmission may start now. Once we look and see the forest, we can find a way out.