I stumbled upon him, sorting out applications for the accelerator of AI-projects " Archipelago 20.35 ". And there was a feeling that either something cool passed by us unnoticed, albeit still in the initial form of development, or it is something incomprehensible, which will then "fall off" by itself.
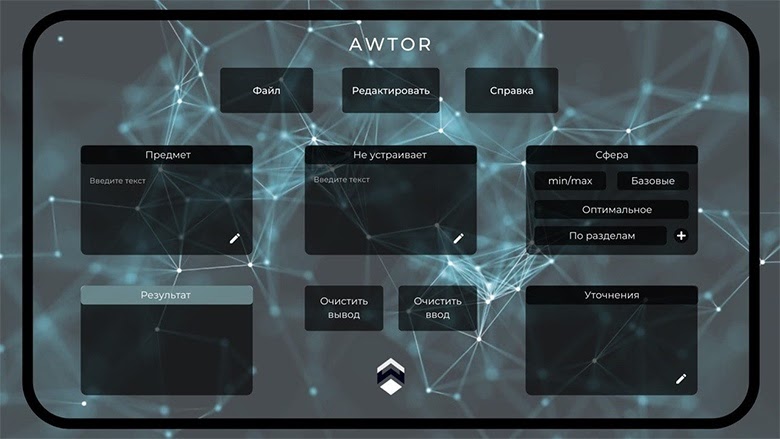
In any case, the position allows me to pull out contacts from the application, chat and write here what happened in the end. In short, this is not label recognition by a neural network or Malevich Gzhel. Everything is more complicated here. And selling such products is, of course, also difficult.
The interlocutor is Galina Pastukhova, mathematician, deputy director for science and innovation at the Academic School of IT at PSU and head of the programming department at PSS Corporation, who simultaneously manages the development of the system and participates in the creation of its architecture.
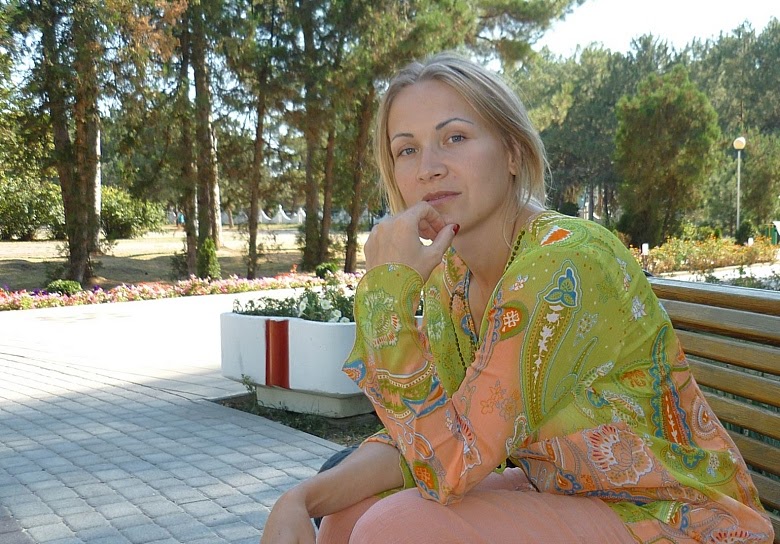
- You declare that all potential inventions can be found with your Awtor design. What does it mean?
- Any object and any invention is a combination of physical quantities. And some of them are in opposition to each other. For example, the weight and capacity of the battery. For example, the longer the drone flies and the more it lifts, the heavier it should have a battery. Recently, humanity has been following the path of detecting and removing these contradictions. Most inventions lie at the intersection of overcoming contradictions.
We took the Soviet base of patents from 1963-1989, processed it and gave it to the neural network. The goal was to identify how the key physical properties of invented things change over time. If the properties are defined and their sequence is set, then the further development of the subject will proceed in a certain order and in certain physical quantities.It doesn't matter what kind of object it is - a spoon, a bicycle or a pipeline.

Awtor's ideology
We have caught this evolutionary spiral. We can predict how an object consisting of certain elements, which are characterized by certain physical quantities, will change in the future. In this case, it is not necessary that each step corresponds to a real invention, the appearance of a new version of the object. Humanity can easily jump over some steps, some decisions may turn out to be economically inexpedient.
- What do they think about it?
- Yes, the problem of mistrust ... it is cosmic. Therefore, we decided not to make loud statements in various media at once, but first go to our Perm enterprises. We have many large science intensive industries in the region. And all the options that they decided with the help of our system, we began to collect on our website .
For example, there was a case with the Chelyabinsk Mechanical Plant. They have a problem with movement inside a certain mechanism: viscosity interferes.
They came and said: "We have heard about your system, but it seems to us that you are lying, this is impossible."We sit down together at the computer, drive in the initial data. The computer gives out 17 steps of the development of an object in a certain sequence. It turned out that the enterprise is on the fourth, and the previous three, they were just in the same order.
But there is also such a moment. The system says at the output: change the density, change the dipole moment. This is not very informative - I would like to see a complex answer, including specific ranges of values, examples of implementation. Our solution was initially tuned to physics, but businesses have a lot of challenges and a chemical level. Therefore, we are now building up the knowledge base in this area.
- That is, in essence, the machine finds what contradictions lie at the heart of the existing technological barrier and shows ways to bypass it?
- Analysis of contradictions is an important part. We even created our own classification. There are purely physical contradictions that come from the formula. The simplest example: time is equal to the distance divided by the speed. Oh, so time and speed are in inverse proportion: the more one is, the less the other. This is a contradiction of the first type.
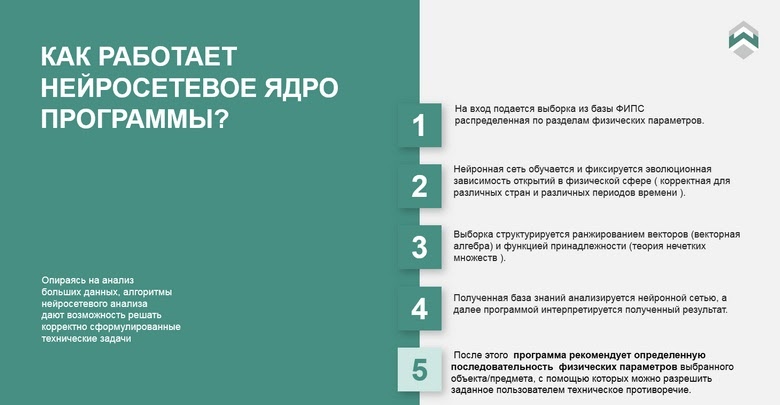
The source of data for training the neural network is the patent base of the Federal Institute of Industrial Property (FIPS).
The next type of contradictions are more complex systems, where one formula is mediated in the second, third, fourth. And when you expand them, you look at the left and right edges, you find contradictions of the second type. As an example, I will give the mass concentration, which can be expanded into the product of linear density () and units per meter: ... As a result, we see that it is inversely proportional to the length.
Finally, we come to the third contradiction, where socio-economic factors are added. For example, how is it that a sports KamAZ is both a reliable car, winning from year to year in competitions, and very inconvenient - only Russian men got used to driving it.
Or why do all hunters love to ride a "loaf"? For some reason, no one has moved to Lexus, everyone is riding "loaves" - taking into account the impassability of the Perm Territory. Why? Here is both reliability and maintainability, and if something falls off, it's not a pity - we will change, cut off, screw, weld, and so on. This is an interesting thing, it is difficult to formalize, but it must also be taken into account.
- What did you use as test problems for your system? How was everyone set up?
- Our experimental model was a syringe, it was he who we first began to functionally disassemble. We set a goal - to increase the speed of drug administration. And the system's responses were used to track whether our developments were going in the right direction. At a certain stage, the machine came up with an interesting proposal for a needle: make not one exit hole in it, but ten. As a person, I understand that this is unprofitable, based on today's ideas about the syringe. But the solution itself is unique. And the car gave him away.
Among the responses was a proposal to change the chemical composition of the needle. But this solution already exists: ice microneedles exist. Ice, of course, not from water, but from a suitable substance with a medicine that can be frozen. He stuck it in super-safe - and left it. The drug melted, and everything resolved.
βThis whole story with ten holes is a bit like a watering system.
- This is a good example of functional isomorphism. From a functional point of view, in terms of a set of key physical characteristics, the syringe and the watering system can be considered one essence. But at the same time, a large number of holes in the irrigation system is the norm, and in the syringe it still seems to be something unusual. The goal of our program is to say that it is possible. And, as it turned out later, such solutions exist in principle, although they are used for somewhat different purposes.
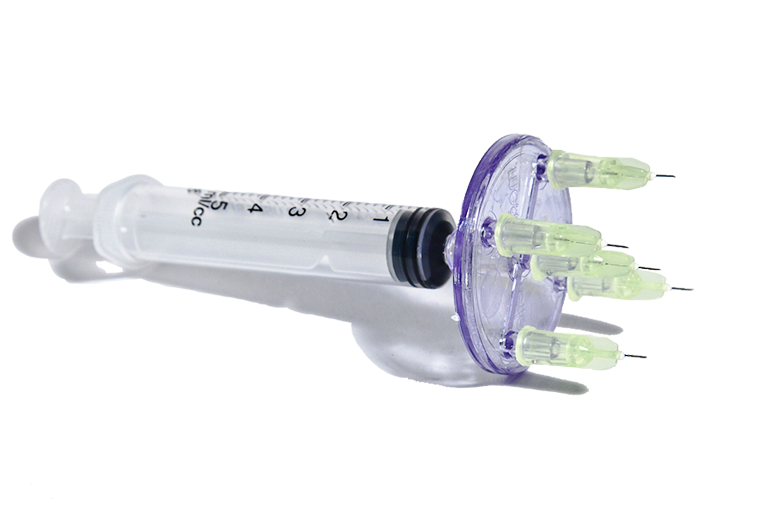
5-Needle Mesotherapy Multi-Injector Syringe
- What other challenges can Awtor solve? Can you give an example when the result produced by the system was used in practice?
- One of the requests came from the NTI industry union "NeuroNet". There was a problem with non-invasive sensors for the head: poor signal transmission, wild interference. We entered the initial data, we look at the results.
And among other primitive responses (like a hole in the skull), the machine suggested changing the temperature. Simply heat the sensors to bring them closer to scalp temperature.When this option began to be implemented, it turned out that when the sensors were heated, the head began to sweat. And sweat became an excellent signal transmission medium, it further enhanced the heating effect.
By the way, on 03/21/2019, when we finished working on the patenting module, our system sent a patent application to FIPS. She was accepted. And we are the first in the world.It is clear that we were deployed there, because only a person can apply for a patent, since the process of invention is a human activity. But we have created a precedent.

And then we began to argue, who is the author here? Created a whole classification: as if there are centaurs of the first level, second and third.
Level 1 centaurs are those who enter data into the neural network. Then those who interpret it. In general, we will soon complete a video about this and post it on our YouTube channel.
- Tell us about the stuffing of Awtor: what are the architecture and mathematical apparatus, what language is it written in?
- Awtor is developed by my team, I am the scientific leader and technical director of the project. It is based on Kohonen's neural networks and our know-how - an algorithm for configuring and training them. The program is written in Python and is a typical monolith. Placed on a specific workplace, protected with a HASP key. It works under Windows as an ordinary lightweight application (the distribution kit of the current version is 40 MB), without any special hardware requirements. We are not considering cloud architecture yet, primarily due to information security risks.
- And what does the process of working with the system look like?
- The interface is very simple. In the "Subject" field, enter the subject that needs improvement. For example, we need to improve a pump in order to lift oil that has thickened at low temperatures from fields. So, we drive in the "Pump".
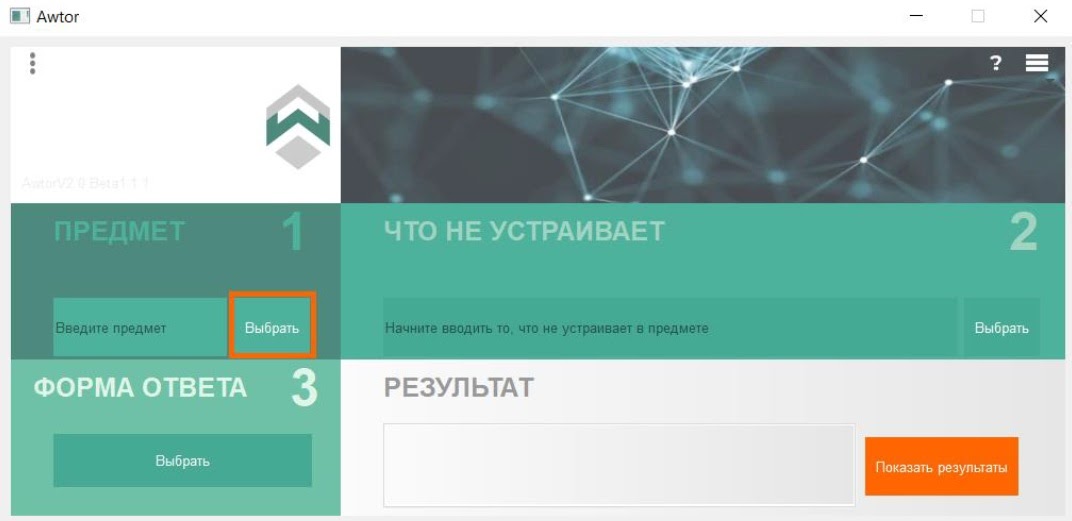
At the next stage, the system asks us to tick off several physical quantities that are fundamental in this subject. We note the density, molar mass, whatever.
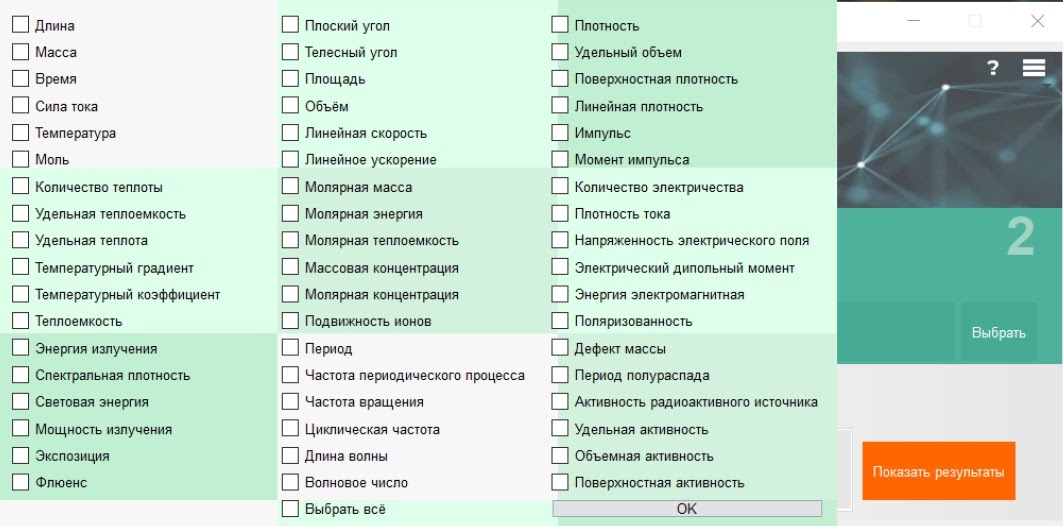
Then in the field "What does not suit" enter "Viscosity". We start to gain, the system tells us options, says that there are four types of viscosity: dynamic, kinematic, and so on. Select "Dynamic Viscosity" and press the "Execute" button.
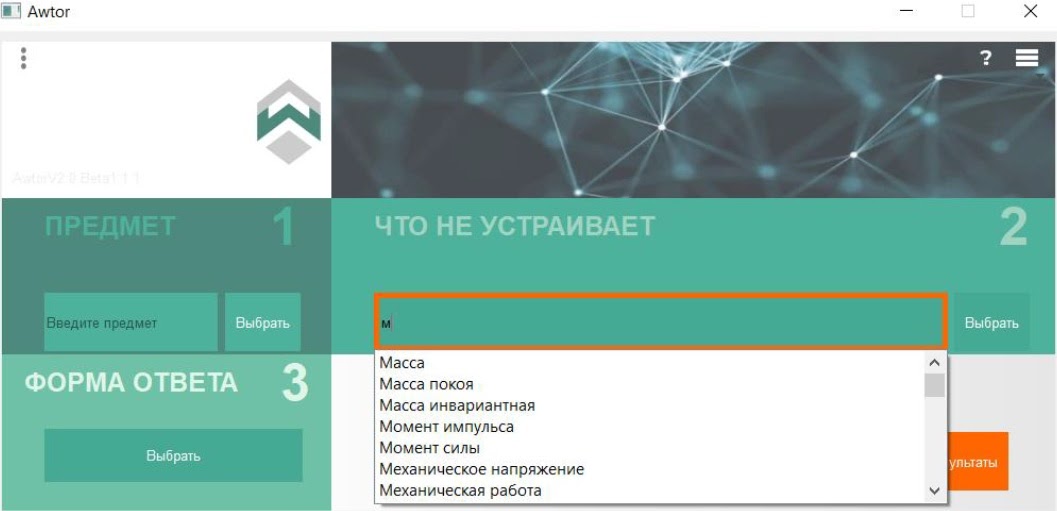
The system proceeds to refine the key parameters. It is necessary to indicate what it is: a phenomenon, a process or a quantity. The classifier works by the degree of entropy - how well the user knows this characteristic of the object. If nothing is known, select "Phenomenon". If something is known - "Process", and if there is exact data - "Value".
The program makes a calculation and displays in the appropriate field a sequence of physical parameters of the object, with the help of which it is possible to resolve a technical contradiction specified by the user.
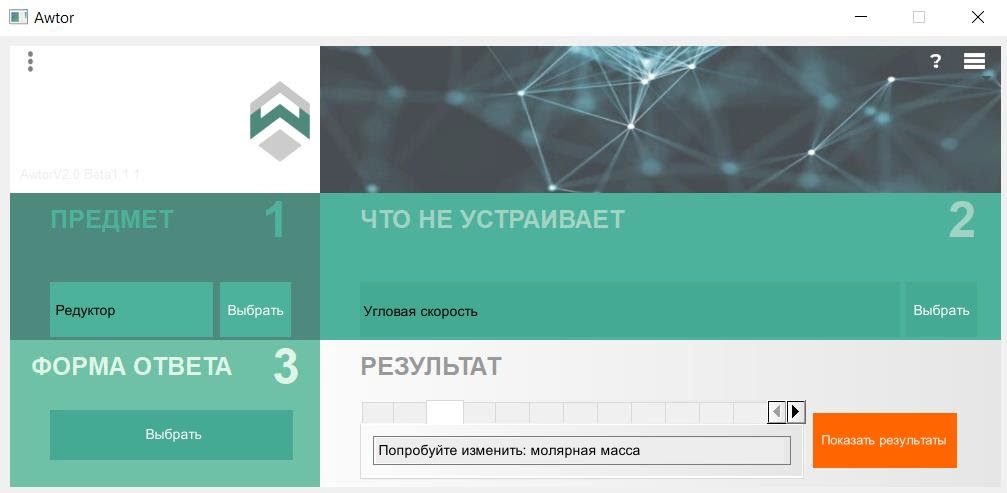
"Reducer" - a set of physical quantities, and in the "Result" field - answer options, arranged according to tabs with sections of physics.
Let's say the machine produced a chain of 18 answers. It is reasonable to return this wave of answers to the machine again. If before that we marked five physical quantities, now we will mark ten, adding those that are in the response of the machine. Suddenly she will give us a more appropriate answer at the next iteration. Because there are really many answers, and you can see from them how they become more complicated in terms of the technologies used. The latest answers are generally from the field of ultrasound or photonics, for which humanity is simply not physically ready.
The response from the machine is one or more physical quantities. For example, temperature. That is, it is methodically clear how to proceed further. Need non-viscous oil - heat it up. But I want to get not only the value, but also the interpretation: how exactly to change the temperature in order to achieve the result.
- And the system does it?
- Unfortunately, the interpretation is too tough for the machine. For this, not deep neural networks are needed, but some other mechanism, because the choice in the end will always rest not on technology and technology, but on the economy. What is more profitable will be changed by engineers at their enterprises. But so far the economic parameters are a big distant dream.
While the interpretation of the answers is a human prerogative. Only a specialist will understand, looking at them, what is economically feasible, what one can afford to change in existing technologies. Now it is such a specialized tool for engineers.
- Are there systems similar to Awtor?
- There are no direct competitors, but there are similar ones. For example, the developments of the founder of AI in Belarus Valery Tsurikov: True Machina and Invention Machina. They have a completely different machine. In True Machina, scientific works are subjected to semantic analysis, from which the essence of the discovery is highlighted. And then, using combinatorial methods, the discovery is run through the hierarchy of technical functions - for what it can be used. A base of improvement concepts for each of the functions is being formed. And when a user formulates a request, the system simply gives out thousands of suitable concepts for it.
Another type of similar systems is a family of TRIZ-based tools. But the role of a person is great in them, and decisions strongly depend on the knowledge and train of thought of a particular user. Too subjective.
- I saw on the website that in the future Awtor will be able to solve social and economic problems. What does this mean?
- Yes, this is a reasonable next step. Physics was done, chemistry was done, then it was the turn of other sciences, primarily economics. It is very important to teach the system to operate with such concepts as ergonomics and convenience. True, you will have to create standards for these terms. What is beauty? Each has its own. What is ergonomics? For each his own. Therefore, it is necessary either to define universal standards that do not depend on a specific person, or to form a profile of a specific user and give the result in accordance with his ideas about what is good and what is bad.
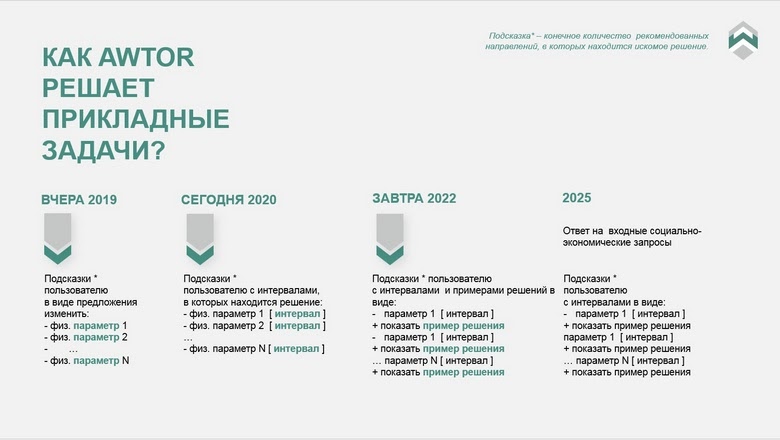
This is a cosmically immense task. While we are thinking how to approach it. But at the same time, without this last module, the whole project loses its meaning and remains a niche utility for engineers.
- Are there any plans to create a mobile version of the system? Open API? Connect her with voice assistants, with the same "Alice"?
- The mobile version of Awtor is a smart step in the development of the system, as is the integration of voice input. Then the system could, for example, ask clarifying questions to the person. But the important thing here is the transition to another target segment, from engineers to the user market. We have already thought about this. But this is a huge power in the context, the analysis of a specific person comes to the fore.
I would like Awtor to be available and useful to everyone, including the child. We will most likely come from the side of the children.
, .
We have a hypothesis that interaction with the system can be significantly improved by eliminating a number of iterations if we understand how a child learns to work with the program on his own. We even have a "Young Inventor" competition: ask the machine, it will answer, and you make an interpretation.
- Probably selling such a complex system, explaining why and why it is needed, is also a space task?
- This is a new product, and you have to get used to it. The market niche and target group have to be formed from scratch, consumers have not yet realized their needs. Therefore, only one logical trick passes. You come to the plant and ask: "What is your problem?" - "I have a problem with wave attenuation in the crystal." - "Wonderful. Is there a skeet chief, an engineer, with whom we will quickly sit down and see? "
And when the manager sees the answers, and the chief engineer confirms that the sequence of answers is exactly the same as they followed for the last 15 years, and that now they are here, and there are still 12 options ahead ... That's it, this client is ours.
- And for what purpose are you participating in the educational intensive "Archipelago 20.35"?
- We hope to qualify as we need help. From the point of view of science, we are doing well: back in 2018, at a conference at Moscow State University, we turned to physicists, asked them to break our machine, catch it on erroneous answers or on missing important options. Nobody managed to do it.
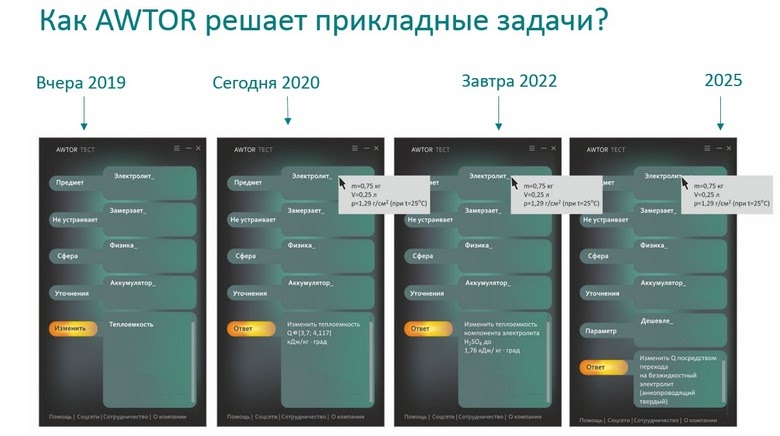
But on the sales side - a failure. Permians know everything about us, but they cannot go beyond the borders of the region. They showed their decision to investment funds, but did not find understanding. Perhaps we are somehow not telling about ourselves that way. I would like to learn how to correctly fit my idea into market realities.
- I wonder why you got involved in such a specific topic as the evolution of inventions? There are things that are more popular and visual, some kind of computer vision?
- As a person who has been studying and developing artificial neural networks for 20 years, I will say this. Enough kindergarten, XXI century in the yard. What is this achievement: the neural network has drawn a picture or a portrait? Each pixel is easy to describe. This is not artificial intelligence, but just some sort of iterator. The neural network learned what was driven in there. Again subjectivity, again a human decision.
Go to another problem. At least in the task of classification. Take a subject area that describes only 10%. Okay, good, 50%. Does not work? So, maybe we will spend strength and perseverance on identifying the necessary gradations, characteristics, creating classifiers?
The classification of contradictions is a worthy topic that no one tackles. Everyone is talking about them, about the technological barriers of NTI. But it is realistic to describe, develop a device for overcoming them - there is no progress here.