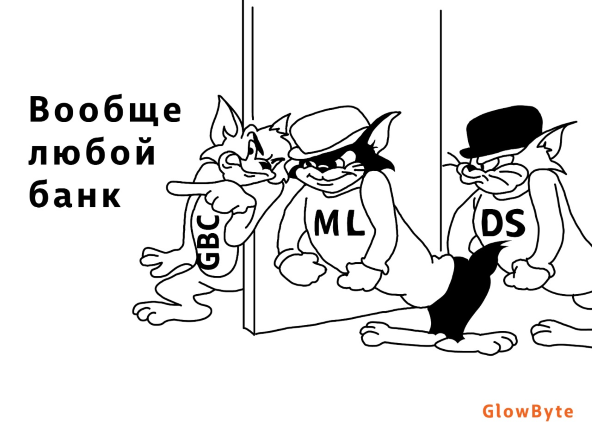
Hello.
We are the Advanced Analytics GlowByte team and are launching a series of articles on modeling in credit risk management. The purpose of the cycle is to briefly talk about the field, expand the vocabulary of professional terms and provide links to useful articles and books. In the introductory article, we will show the features of the application of ML and DS in the field of credit risk, without deep diving into the subject area.
Next, we will reveal the issues of modeling methodology, working with the components of credit risk, as well as approaches to calibration and validation, which take into account the specifics of the operation of models in a bank.
The basis of publications is our project experience in the development and implementation of analytical models in the banking sector.
And now under the cat.
What are the risks?
In simple terms, credit risk is the risk of customers violating the terms of payment of funds under loan agreements.
We will focus on three challenges that arise within the framework of credit risk management.
- Rating modeling;
- Credit offering;
- Calculation of the level of expected losses.
Why exactly on them?
- These tasks are always relevant for financial institutions;
- They can be transferred to other industries (telecom, industry, insurance);
- They have enough space for ML and DS methods.
For the general classification of risks of financial institutions and the context, see the review [1] .
Everyone's pipe (pipeline) or scheme of the credit process
Schematically, the credit process looks like this:

Part of this process from application to issuance is called the credit conveyor. There are simplifications in this scheme. For example, we consider the process within the framework of one loan product, i.e. marketing issues (Marketing Optimization, product cannibalization, customer churn, etc.) remain outside the brackets. The processes of prescoring, expert rating adjustment and application of stop factors by underwriters are excluded from the pipeline. Stop factors mean restrictions, the nature of which is, first of all, in the structure of the product that the bank offers to the client. An example is the entry of a client into the list of bankrupts or the presence of delinquency on loans in other banks.
Rating modeling
The task of rating modeling (RM) is to build a customer rating model for subsequent ranking. The rating is carried out in relation to various negative events - deterioration in creditworthiness, bankruptcy, etc.
Depending on the context, this task can be classified in different ways:
By stage of the customer's life cycle:
- Application (applicative) scoring is used for new clients or clients with a small (or long-standing and irrelevant) history within a financial company. In building such a rating model, the profile and profile of the client, data on his payment behavior in other financial institutions (available in the Bureau of Credit Histories) and data on entering different lists, for example, negative lists of the Central Bank for legal entities, are important. Application scoring is used to decide whether to grant a loan to an applicant.
- . — -. , .
:
- «» : ( ) , .
- «» : . , , , .
:
- «» . . .
- «» . ( ) . — Z-score [2].
:
- . .
- .
:
- Stand-alone — , . — . , .
- «Supply chain finance» — . , , , ( ) . , — , [3].
:
- : , , . — , ( , ).
- , .. . , .. .
The peculiarities of solving this problem in the first approximation can be found in [1] , [4] , [5] , [6]. We plan to talk about the design features in the next article in the cycle, dedicated to the development methodology.
Among the related tasks, it is worth mentioning the task of credit offering (see below) and the task of selecting a cutoff threshold based on a scoring score - determining the approval threshold. The latter problem is not covered in this article, but contains space for cutting-edge ML approaches. For example, there are attempts to use RL [7] .
We should also briefly mention the current trends to increase the quality of the developed rating modeling models:
Rating modeling is less and less encountered as an independent task and more and more in conjunction with others, being part of an applied application for solving more general problems. One of these is credit offering. We go to it.
Credit offering or how to make an offer you can't refuse

The result of the rating model (the absolute value of the estimate of the probability of default - PD) can be used to solve the problem of credit offering. By credit offering we mean, first of all, the task of setting an initial limit for a client.
Of course, the PD value alone - the forecast of the probability of default - is not enough to determine the optimal limit. You need to understand the acceptable range of limit values that are reasonable to offer to customers. This is necessary so that the amount at least indirectly reflects the needs of the client and his ability to service debt.
A benchmark in this case can be, for example, the turnover of the client's own funds for non-credit products.
What else do you need to know? For a better understanding of the problem, you need to have an idea of the structure of the cost of the loan. It is schematically presented in the following diagram (peeped in [11] ):

"Resource" - the value of money at the expense of which lending is carried out (for example, the rate on deposits, which attracts depositors' money and provides the required money supply). "Margin" - the expected profit from the loan. "Risk" - deduction in case of loan default. "Expenses" - the costs of attraction and maintenance.
In this framework, rating modeling can be used to determine the size and structure of the Risk block. "Resource" is largely determined by the key rate of the Central Bank. "Costs" and "margin" are product components, often indicated in the product passport.
In other words, "Risk" is just one of the components that affects the final profitability of a trade.
What about others? It looks like an optimization problem arises. Let's try to formalize it. It is worth emphasizing that there can be many options, and it is primarily worth relying on the business task and the context of the development process.
Let's start with a simple option and then show the potential development points of the solution. The easiest way is to optimize the profitability of a trade.
Let the loan agreement be issued for the amount L (limit). This contract has a predicted probability of default PD. As a first approximation, we assume that the client at the time of default has a debt equal to L.
Then the optimization problem will look like this:

We see that PD is fixed and the dependence on L is linear. It would seem that there is nothing to optimize.
However, in real life, PD depends on L for the following reasons: the larger the limit, the more difficult it is to service debt and, accordingly, the higher the probability of default. In this case, our task really turns into an optimization one. However, there is also a nuance here. There are clients with different incomes in the sample, so absolute values will not be enough. It is best to build dependencies not on the limit, but on the level of debt load, i.e. parameter :

Addiction can be reconstructed on historical data or pilot. Also, product stops can affect the optimization task. For example, in the product passport, the acceptable limits of the risk level (probability of default) may be indicated. Then optimization is performed only up to the specified limit.
Further complicating, who is interested, then under the cat:
, (, ) (-, EAD — Exposure at default — ) . , , ( EAD, , LGD – Loss Given Default).
EAD . LGD , (, ..) ( LGD ). 0.9-1.
, PD . :
, (, ), , :
«» — , «» — . Marketing Optimization.
— . , , , ..
. -.

EAD . LGD , (, ..) ( LGD ). 0.9-1.
, PD . :

, (, ), , :

«» — , «» — . Marketing Optimization.
— . , , , ..
. -.
What else to google? Keywords risk-based limit, credit-limit management profit-based approach.
So the money is offered and given to the clients. But some of them are starting to go overdue. How to manage the situation?
Reserves and the role of DS for their calculation

Determining the amount of risk is key in the bank's activities: depending on the risk appetite, the bank determines which clients it is ready to work with. But in any case, to minimize possible losses, a cash reserve is formed in the form of cash or liquid securities. In the worst case, the bank loses the entire portfolio, but this is unlikely, therefore it is not very efficient to have a full reserve. Some balance is needed.
To do this, you need to accurately determine the amount of money that should be reserved. This is how the task of ensuring the capital adequacy (required capital) for the expected losses appears. (Expected Loss - EL). Capital adequacy requirements are determined and monitored by the regulator (Central Bank).
Historical reference:
, . . .
, DS ML .
1974 , .
Basel I 1988 . Basel I , 8% , (, – Risk-weighted Assets (RWA)).
, the Basel I Capital Accord RWA, .
, 100 (-, ):
.. 4.
. : XGBoost , , .
Basel I Basel II. -, Basel II ( ) , , , . Xgboost ML DS.
Basel III . . . [6].
? , , RWA:
1. – . — 590-.
( [12]):
, 5 , . , (, ), ( ) .
2. (, 483-) PD, LGD EAD.
:
, , , . , , , , data scientist’.
(Expected Loss – EL) (Unexpected Loss – UL).
, DS ML .
1974 , .
Basel I 1988 . Basel I , 8% , (, – Risk-weighted Assets (RWA)).

, the Basel I Capital Accord RWA, .
, % | |
0 | |
50 | |
100 |

.. 4.
. :
Basel I Basel II. -, Basel II ( ) , , , . Xgboost ML DS.
Basel III . . . [6].
? , , RWA:
1. – . — 590-.
( [12]):
« , 590-, . , , ( ) . .».
, 5 , . , (, ), ( ) .
2. (, 483-) PD, LGD EAD.
:

, , , . , , , , data scientist’.
(Expected Loss – EL) (Unexpected Loss – UL).
Losses in rubles are the product of three components:
- probability of default (PD - Probability of Default)
- the amount of the payer's debt at the time of default (EAD - Exposure At Default),
- a share of this amount that will remain unpaid (LGD - Loss Given Default).
In general, this formula:

we will come across more than once in a series of articles - this is a refrain of the problem of provisioning in credit risk.
After this kind of decomposition of EL (ECL), it becomes possible to simulate (DS and ML, hello!)) Each of the mentioned values PD (binary classification model), LGD (regression model), EAD (regression model), where, within the limits specified by the controller requirements at different stages of modeling (development, calibration and validation), it becomes possible to use statistical methods and machine learning algorithms.
For those who like more complicated things:
EL UL (Value at Risk – VaR) – , ( 99%) .
PD, LGD, EAD , .
3. 9. .
9 :
9 :
9 DS ML-.

PD, LGD, EAD , .
3. 9. .
9 :
- ( );
- ( «Lifetime-» «Lt») PD, LGD, EAD, ; ECL — Expected Credit Losses;
- ( ).
9 :

9 DS ML-.
?
- 29.12.2012 N 192- « »
- 6 2015 . № 483- « »
- 15 2015 . N 3624- « »
- 6 2015 . № 3752- « , »
- [13].
The regulations and instructions were concerned, the books were read, but where is DS? As promised - DS is in the
Rating
modeling |
Credit offering | Calculation of the level of
expected losses |
|
---|---|---|---|
Problems
Solved with DS / ML |
- Determination of
the rating algorithm ; - Determination of the threshold of approval; - Calibration. |
- Development of an
optimizer; - Development of models used to select a loan proposal. |
of PD, LGD, EAD components; - Calibration. |
conclusions
The main conclusion after writing an introductory article for us (abv_gbc, alisaalisa, artysav, eienkotowaru) is as follows: it is extremely difficult to briefly describe even three problems arising in the calculation of credit risk. Why?
A detailed methodology has been developed for these tasks, which provides good food for ML and DS thinking. These reflections develop approaches to respond to increasingly complex market challenges. Tools based on such approaches, from complementary ones, are gradually becoming the main ones in decision making. All this together allows transferring the best practices and intuitions of risk modeling to other industries (telecom, insurance, industry). Which ones? We will tell in the next articles of the cycle.
List of used terms
- Default - failure to fulfill obligations under the loan agreement. Usually, default is considered to be non-payment under the contract within 90 days.
- PD - probability of default - default probability.
- EAD – exposure at default – . , , = + .
- LGD – loss given default – EAD, .
- EL – expected loss – .
- EL – expected credit loss – .
- – , .
- - – .
- SCF – supply chain finance – — - .
- RWA – risk-weighted assets – , ; .
- (IRB) – , , , .
- 9 (IFRS9) – , , , .
- VaR – , .
[1] Leo Martin, Suneel Sharma, and Koilakuntla Maddulety. "Machine learning in banking risk management: A literature review." Risks 7.1 (2019): 29.
[2] en.wikipedia.org/wiki/Altman_Z-score
[3] www.youtube.com/watch?v=rfCamyEURyw&list=PLLQmSdmAWzkKeiOC1b-nxpoACqgfTc0G5&index=7.
[4] Breeden Joseph "A Survey of Machine Learning in Credit Risk." (2020).
[5] Sorokin Alexander. "Building score cards using a logistic regression model." Online Journal of Science of Science 2 (21) (2014).
[6] Baesens Bart, Daniel Roesch, Harald Scheule. Credit risk analytics: Measurement techniques, applications, and examples in SAS. John Wiley & Sons, 2016.
[7] github.com/MykolaHerasymovych/Optimizing-Acceptance-Threshold-in-Credit-Scoring-using-Reinforcement-Learning
[8] riskconference.ru/wp-content/uploads/2019/10/%D0%A1%D1%83%D1 % 80% D0% B6% D0% BA% D0% BE_% D0% 92% D0% A2% D0% 91.pdf
[9] Masyutin Alexey. "Credit scoring based on social network data." Business Informatics 3 (33) (2015).
[10] habr.com/ru/company/vtb/blog/508012
[11] vc.ru/finance/83771-kak-formiruetsya-procentnaya-stavka-po-kreditam
[12] Farrakhov Igor. "IFRS 9: Provisions for Estimating Expected Credit Losses." Banking Review. Application "BEST PRACTICE 2 (2018).
[13] Bellini Tiziano. IFRS 9 and CECL Credit Risk Modeling and Validation: A Practical Guide with Examples Worked in R and SAS. Academic Press, 2019.