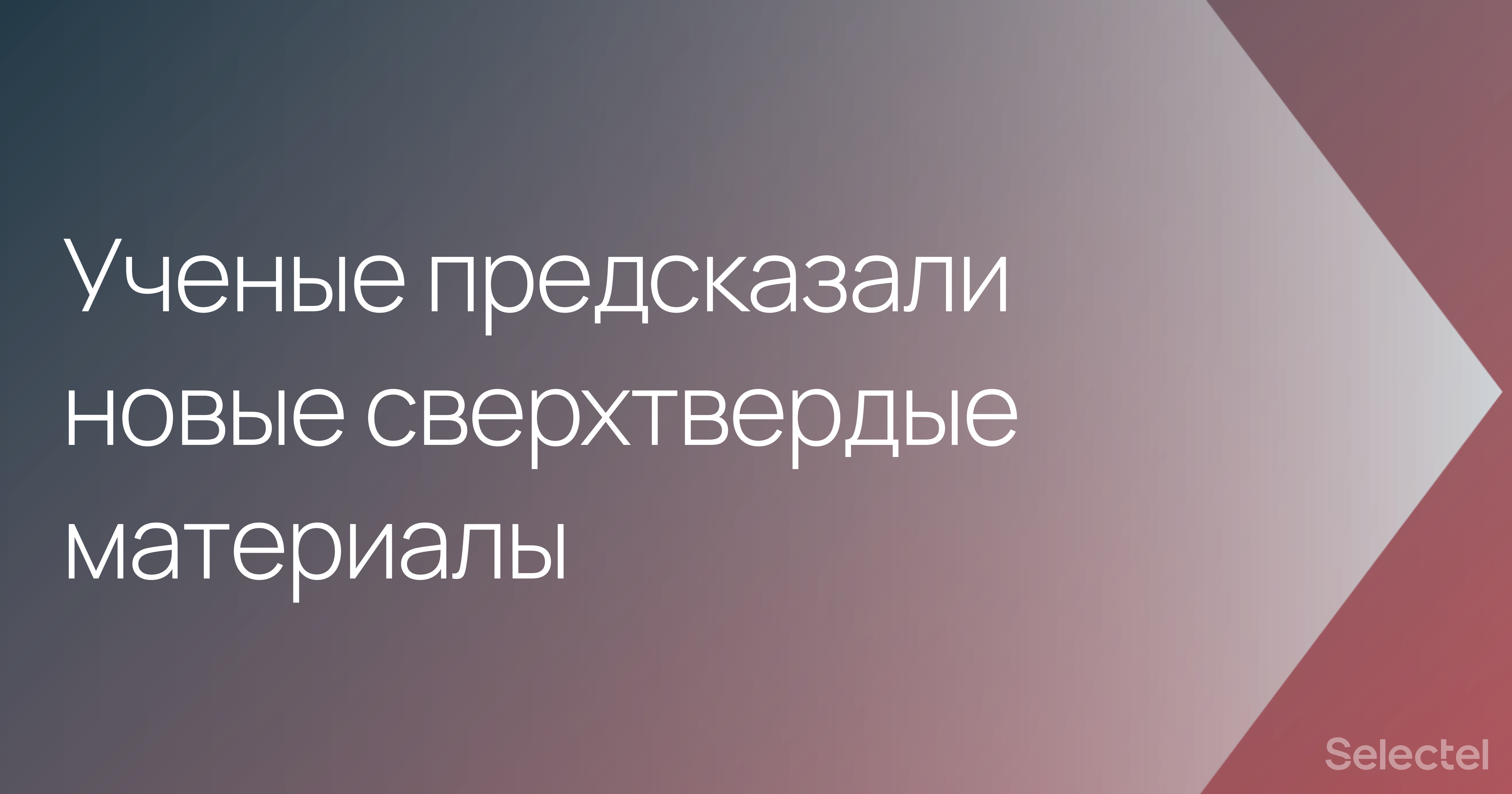
Skoltech scientists have created a neural network model that computes superhard materials. It was found that in addition to diamonds, the existence of other superhard substances is possible.
Scientists are interested in superhard materials because they are applicable in many industries: oil production, metalworking, jewelry, machine tools, microelectronics, and high technology production. They are used for drilling, cutting, polishing, grinding. Therefore, it is important to discover new compounds with properties of superhard materials.
At the moment, diamond is the hardest material known. But its application is not always expedient and expensive. Therefore, for example, heads for drilling rigs have long been made from pobedit - a compound of tungsten with cobalt, containing diamonds interspersed. Another well-known super-strong material is lonsdaleite - a carbon crystal, a type of diamond. It is based not on a cubic lattice, but on a hexagonal one. According to calculations, lonsdaleite can be 1.5 times harder than diamond and can cut it. However, in nature, it is almost impossible to meet it in its pure form.
Hardness and fracture toughness are two important properties of superhard materials. The first - speaks of resistance to fracture, and the second - to the formation of cracks and splits. Machine learning helps you discover the properties you want using computational materials science techniques. The underlying theoretical model makes it possible to identify the corresponding properties.

The model was created by 25-year-old Efim Mazhnik , a graduate of the Moscow Institute of Physics and Technology and a post-graduate student at the Skoltech Center for Energy Technologies, the Laboratory for Computer Design of New Materials. The scientific management of the work was carried out by Artem Oganov , professor at Skoltech and MIPT. Oganov's study focuses on the theoretical design of new materials, the state of substances at high pressures, and the development of methods for predicting the structure and properties of substances. In 2018, he and his colleagues predicted the existence of tungsten boride WB5 with crystals whose hardness is superior to vicite alloys.
Scientists have developed a method for predicting the properties of a material from its crystal lattice using convolutional neural networks on graphs.
Convolutional neural networks are a special architecture of artificial neural networks that focuses on pattern recognition and is part of deep learning. The network got its name from the "convolution" operation, during which each fragment of the image is multiplied pointwise by the elements of the convolution matrix. The summed result is referred to a similar position in the output image.
Such networks can be trained to understand the properties of even those materials with which they have not previously encountered.
Efim Mazhnik explains that due to insufficient data on the necessary models, they used intermediate properties - elastic moduli, for which there are more available characteristics.
Artem Oganov added that the model was used to calculate the properties "for more than 120 thousand known and hypothetical crystal structures." “Our model confirms that diamond is the hardest known substance, but also points to several dozen other potentially highly hard and superhard materials,” Oganov explained.
The research results are published in the Journal of Applied Physics . The research grant was supported by the Russian Science Foundation (RSF).
The Skoltech Laboratory for Computer Design of New Materials was created a year ago as part of a joint project between the Russian Science Foundation and Gazpromneft STC.
