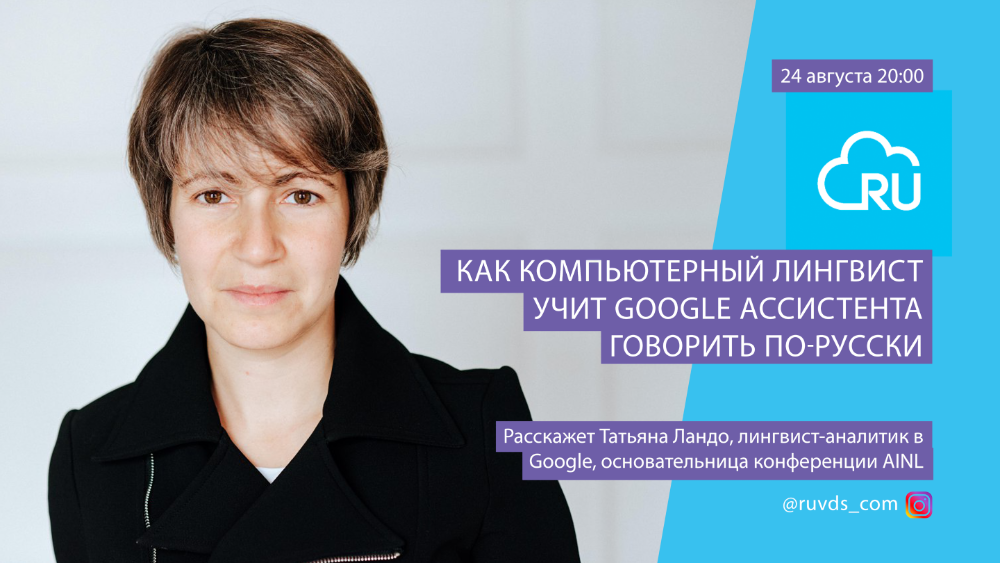
On August 24, we spoke live with Tatiana Lando, a linguist analyst at Google. Tatiana is working on Google Assistant and is involved in projects between production and development. She explores how people talk to each other and what strategies they use to use this in teaching the assistant more human behavior. Came to Google to work on an assistant for the Russian market and the Russian language. Prior to that, she worked at Yandex for 8 years. She was engaged in linguistic technologies, extraction of facts from unstructured text. Tatiana is one of the founders of the AINL: Artificial Intelligence and Natural Language Conference.
We share with you the transcript of the broadcast.
When I say that I am a linguist, at best they ask me if I have read the Strugatskys, where it was about “structural linguists”. In the worst case, I am asked how many languages ​​I know. Linguists are not people who know many languages. People with any specialty can know many languages, it is not connected. A linguist is a person who understands how language works, how communication between people works. This is a large scientific discipline, it has a lot of aspects besides what I do. There is an analysis of texts to establish authorship, there is linguistics in forensic science - when linguists determine whether there is extremism in a text, for example. This is an example.
Computational linguistics, which I do, is mainly aimed at developing speech interfaces between technology, computers and humans, on the one hand, as well as introducing numerical methods into language processing. There is a whole area of ​​corpus linguistics - when we try to automatically process texts in large quantities in order to draw conclusions about how the language works based on the data obtained. I'm working on a Google Assistant - it's a voice interface between a phone or computer and a person.
Before moving to London and starting work at Google, I worked at Yandex for 7.5 years, where I also studied computational linguistics. The tasks that I was engaged in at Yandex covered a fairly wide range of tasks in computational linguistics. We studied morphology, syntax - how words change, how words are combined; In this regard, the Russian language is more complicated than English, since there are no cases in English, there are only two forms of nouns, everything is relatively simple (in Russian - 6-9 cases, a strange plural formation - well, all native speakers know these features). Therefore, when I switched to Google, I was hired as a specialist in the Russian language, although now I am already doing something else.
Are there any vacancies related to the development of the Russian version of the Google Assistant? How do you get a Google Assistant job?
There are no special vacancies associated with the Russian version of the Google Assistant. Google tries to develop methodologies aimed at the maximum number of languages ​​at once, and the specificity of languages ​​should be solved using data, and not using specific methodologies. That is, the algorithms for Russian, English, German, Chinese - all supported languages ​​are made the same, albeit with some nuances. There are a lot of common parts, and people who deal with a particular language mainly monitor the quality of the data and add special modules for individual languages. For example, for Russian and other Slavic languages, morphology is needed (this is what I just talked about - cases, plural formation, complex verbs). And in the Turkish language there is an even more complex morphology - if there are only 12 noun forms in Russian,then in Turkish it is much more. Therefore, we need some kind of special modules that process language-dependent parts. But this is done with the help of linguists who know their native language and general language engineers who write algorithms using data; we work together to improve the quality of these algorithms and data. Accordingly, we do not have special vacancies for the Russian language, but there are vacancies in the development of Google Assistant, mainly in Zurich, California, New York, quite a bit - London.We do not have special vacancies for the Russian language, but there are vacancies in the development of Google Assistant, mainly in Zurich, California, New York, quite a bit - London.We do not have special vacancies for the Russian language, but there are vacancies in the development of Google Assistant, mainly in Zurich, California, New York, quite a bit - London.
, ?
4 years ago when I moved London was still Europe. Actually, he is now Europe, although not the EU. I really like London, everyone speaks English, no need to learn another language. Not that I didn't want to learn another language, but the entrance to the move was minimal. There are also excellent theaters here, I really love going to the theater. Of course, now all the advantages of London with culture and entertainment are somewhat leveled, but let's hope for the best. In addition, it is much easier with a visa here than in Zurich - for some reason, visa legislation in Switzerland changes regularly. It turned out to be much easier to put me and a few other guys in London, and we settled here. I like it here, I don't want to move anywhere. Business trips to California drive me into melancholy - there you have to take a car, huge distances. Although there are more career opportunities there.
?
It is more difficult than English. Actually, almost all languages ​​are more complicated than English. There are two different sides to this complexity. First, the very complex morphology. In addition, the Russian language has a free word order, which complicates the work when constructing an algorithm. If it is fixed, then for the conditional "mama soap frame" one sentence is needed to teach the system to understand "subject-predicate-object". But if these words can be in any order, more data is needed. At the same time, there are a lot of ready-made resources for the English language; the entire scientific world is engaged in the English language, all corporations are engaged in the English language because they compete in the American market. And much less is involved in other languages, there is less funding, fewer companies. If there are still chunks or ready-made data for German or French,which can be reused, then the Russian language is worse. There are ready-made cases, but they are small and not suitable for everything. That is, much more data is required for the Russian language than for the English one, but there are less data available. Plus additional grammatical layers; You can try to understand the same morphology separately, then you can reduce the amount of data that is needed for high-quality work of the parser in Russian (compared to English).which are needed for high-quality work of the parser in Russian (compared to English).which are needed for high-quality work of the parser in Russian (compared to English).
Therefore, it turns out that the Russian language is quite difficult, like other Slavic languages. Turkic languages ​​are even harder; Oriental languages ​​have other problems: for example, Chinese has no spaces between words, you need to understand how to break an array of text into pieces in order to determine what each piece says. In general, each language has its own jokes, but basically everything can be solved due to the large amounts of language data on which the tasks are solved. Everything rests on the fact that there are not very many of them for the Russian language, and therefore it is difficult to work with it, and for English - on the contrary.
Can computational linguistics reconstruct incomprehensible places, for example, in the negotiations of pilots with the ground?
Oh sure. Basically, the algorithm that would do this would be similar to search suggestions. When you enter a query into a search (for any language), the system offers you the most popular queries, with some filtering. The algorithm, of course, is somewhat more complicated, but we will forget it for clarity. The system suggests the most frequent combinations of words in the query, starting with those that you have already entered. A similar technique can be used to restore obscure places. There are a lot of negotiations, this is a huge corpus of texts, and you can analyze them and see which parts are most common. I myself have never dealt with the topic of negotiations between pilots and the ground, but I suspect that there should be many similar proposals, the structure of information is quite codified, the way of communication is standard - such things are quite easy to predict, so they can be easily restored.
Tell us about acoustics and sound signal purification in voice assistants
About this I know almost nothing, I only deal with written text. Phonetics, acoustics are not my areas. But I'm sure there are audio methods that help clear the signal, and at the intersection between audio signals and the predictive engine, you can filter out hypotheses and restore text.
What is the most difficult language to process and understand?
They are all complex. There is no such thing that any of them is more complicated or simpler in itself; there is a lot of data for English, so it's easier to work with it, and everyone is doing it.
It turns out a vicious circle: when people at conferences want to try out a new algorithm, they take well-known corpuses and watch how the numbers on the metrics grow. To measure your algorithm, you need a good corpus; there are many good corpuses in English, so they run algorithms in English on them, get an increase, and thereby stimulate other researchers to make even more corpuses to find holes in the algorithms. This is a task for which it is easy to get a grant. For example, there are 10 algorithms to automatically answer questions; on the existing corpus, these algorithms are more or less similar, and the researchers decide to create a new corpus to see the difference between them. This is a good task, it allows better tuning of algorithms. They do it, and there are more corpuses for English - and the more corpuses, the more algorithms appear.If you look at the work in the field of NLP over the past few years, conferences measure not even percentages, but fractions of a percent increase in quality.
In general, this is not very helpful in practical problems, and it is not really computational linguistics. This is how engineers deal with language if they are not working with linguists (that is, either mediocre or very theoretical).
Why is there not enough data for the Russian language?
This question torments me all the years of studying the Russian language, from the university where I studied applied linguistics.
I dont know. Maybe there is simply not enough money in Russian science, and therefore little data is being made for the Russian language. Conferences in Russia are generally a bit outdated; there are conferences on applied linguistics, on automatic natural language processing, but there are very few large teams that are engaged in this. Scientists go to big companies - Yandex, ABBYY, now MTS also hires linguists; the profession has become more in demand with the advent of voice assistants. They also go abroad; There are many linguists in startups, Amazon and Google.
The only large corpus is the National Corpus of the Russian Language. There is also a corpus made by my friends - the Open Corpus of the Russian Language; but, in general, it is very difficult to obtain funding for this, and few are interested in it.
There were competitions of algorithms for which small corpora were created for specific tasks - for example, comparing how the system answers questions in Russian or understands commands in Russian - but this data is not enough to train large systems. It also turns out to be a vicious circle in the other direction: there is nothing to train, so there is nothing to train, so there is nothing to measure, so there is no data. Plus, more data is needed than for English. After receiving my diploma, I almost immediately went to Yandex, so it's hard for me to say why it doesn't work out here.
What approach is Google taking more towards language processing? Neural networks or algorithms?
Neural networks are also algorithms. I don't understand the dichotomy a bit, but I'll try to tell you what (and in general) approaches we have in the analytical processing of language in computational linguistics.
The oldest historical approaches are rule-based approaches. Linguists write rules by hand, almost like a programming language. Suppose, in the perceived text, first there is the subject, then the verb, then the predicate; if the predicate is in one case, then - one conclusion, and so on. For example, for cases when the user says something like "ok Google, set the alarm for 7 am", you can make the following rule: put - any word - alarm clock - on - number - in the morning. This is a template that can be described and taught to the system: if you use such a template and it works, then you need to set the alarm at the time indicated in the form of a number. Of course, this is a very primitive pattern, you can do it much more complicated. They can be combined: for example, one template extracts the date and time, then a template for setting an alarm is written over it.
This is a very old approach, it is already 70 years old - this is how Eliza, the first chatbot pretending to be a psychoanalyst, was written in 1966. Then people were very surprised. There were stories that the creators of this chatbot showed it to their colleagues, and they kicked them out of the room to talk to a "real" psychoanalyst. And this bot was written only on the rules - then it was a breakthrough approach. Now, of course, we do not want to do this, because there are a lot of rules needed - imagine how many different phrases you can use just to set an alarm, if you use pure rules, you would have to describe each of them manually. We switched to hybrid systems a long time ago: they can perceive patterns, but in general we try to use neural networks for machine learning and apply supervised approaches to supervised learning. I.e,we mark up the data and say: ok, in this array of what the user can say, this part is time, and it normalizes like that; this part is the device on which the user wants to set the alarm, and this part is, for example, the name of the alarm. So that you can set an alarm clock on the iPhone at 7 am with the name "School". Next, we set a large corpus, train the parser on it, then the parser is applied to user requests, and thus we recognize them. This is how Google Assistant works now, and this is the approach we are using now.Next, we set a large corpus, train the parser on it, then the parser is applied to user requests, and thus we recognize them. This is how Google Assistant works now, and this is the approach we are using now.Next, we set a large corpus, train the parser on it, then the parser is applied to user requests, and thus we recognize them. This is how Google Assistant works now, and this is the approach we are using now.
It sounds primitive, now in the literature and news information often leaks out about how neural networks learn on their own on huge corpuses, they answer everything and support conversations. This, of course, is true, and it's cool, but such approaches are useless in cases where you need the system not only to answer, but also to change its state - at least with the setting of an alarm clock. All the same, an internal representation is required, to which it is necessary to somehow bring what the user said. Even if we have a huge array of texts in which the user asks to set an alarm, and it will not be marked, then we will not be able to train the parser so that it changes the system. We will be able to train him so that he would say “Yes, I’m setting the alarm” and did nothing. But to train the system so that it changes its state, using unlabeled data,not yet possible. So what's recently released by OpenAI and DeepMind - part of Alphabet, the parent company of Google - is cool, they are good chatbot techniques that respond to humans, but there are no techniques that exclude manual labor to change the state of the system. Therefore, unfortunately, the industry now has rather low standards in this sense - not only for the Google Assistant; all assistants work on approximately the same approach with a lot of manual work - either processing data for parsers, or writing rules (which we don't want to do). We try to do manual work with the help of partner companies.excluding manual labor to change the state of the system, no. Therefore, unfortunately, the industry now has rather low standards in this sense - not only for the Google Assistant; all assistants work on approximately the same approach with a lot of manual work - either processing data for parsers, or writing rules (which we don't want to do). We try to do manual work with the help of partner companies.excluding manual labor to change the state of the system, no. Therefore, unfortunately, the industry now has rather low standards in this sense - not only for the Google Assistant; all assistants work on approximately the same approach with a lot of manual work - either processing data for parsers, or writing rules (which we don't want to do). We try to do manual work with the help of partner companies.We try to do manual work with the help of partner companies.We try to do manual work with the help of partner companies.
Tell us about the promising directions in the development of the Google Assistant.
Actually, what we just discussed. Direction - to come up with a new training system that could change the state of the system from data without requiring manual data processing. That would be cool. But so far, although I already have a lot of experience working with assistants, it is difficult for me to imagine even how such a system would work in principle. I understand how neural networks, teaching methods, hybrid approaches are becoming more complicated now, how the preparation of data for training is becoming more complicated - but I don't understand how to make a direct connection between data without a teacher and changing the system. There must be some kind of internal representation that would allow doing this. If someone can revolutionize and reinvent this part, that would be great.
Plus, now a lot is invested in the generation of this internal representation - that is, if we cannot go directly from data without markup to changing the state of the system, we need to make an algorithm that will help to do something in between. Let's say generate a system view from text and then people would clean it up - instead of building it from scratch. This is a very promising direction, and although there is little progress in it, researchers are looking exactly there.
Plus - new signals for answers and for the conversational sanity of assistants. And assessment methods. Now we (that is, in the industry in general) do not have a single adequate method for assessing the quality of dialogue systems, not a single metric by which one could compare the Assistant, Alice, Alex, Siri, and so on. You can poll users, try to solve similar scenarios with different assistants and draw conclusions from this, but no suitable quantitative metric exists. For machine translation, there is a metric, and more or less normal, but for this - nothing; this is one of the problems that are now being discussed in the industry at conferences. Dialogue corpora for English have finally begun; there are also very few of them. There is nothing to count the metrics, there is no one and it is not clear how. If anyone has a good idea,then this person will collect all the laurels at conferences, make a startup and sell it anywhere. I would have torn off any project that would help quantitatively, in numbers, measure the difference between assistants.
Separately, there is hybrid study, which I am doing the most now - in the last year I switched from Russian to this topic. I am doing projects on how to make the Assistant more conversational, more natural. We watch people talk to each other. It is not only what words are used here ("set / set the alarm"); in fact, this part is primitive and has already been solved, although a lot of routine remains to be completed. Unresolved tasks are, for example, when the user says in various forms: "Set me an alarm", "Can you set an alarm?", "Could you set an alarm?" Roughly the same thing, but in one case the command is used, in others - questions. We are looking at this level of linguistics - pragmatics, that is, what goes above meaning - semantics. For those who have studied linguistics or language,semantic markup in text is not a new term, but pragmatics adds additional context to it; that is, not only "what they say", but also "how they say", "why they say". Roughly speaking, if the user says to the assistant “are you stupid?”, He does not want a “yes / no” answer - this is another signal, and you have to ask what was wrong in the system's behavior before; this should not be classified as a question, but as a complaint.
My group - I now have three linguists left after moving to another department - is now trying to understand how people interact with each other, how people interact with assistants, and how to turn this into signals for machine learning and really train the system to better understand things transmitted non-verbally and indirectly.
How about the number of retries and stubs in relation to the question in the problem statement?
This is a good metric, but, unfortunately, it only takes into account the number of phrases that the user has not understood by the assistant. It doesn't tell us how difficult those phrases were, how pleasant the user experience was. Write to me later after the broadcast, everyone who has ideas for comparing assistants, you can talk about this separately - but something very complicated is required there. By separate sections, we have already learned to understand something - for example, what percentage of the user's requests the system does not understand, or understands from the Nth time, and where there were errors - in the translation of speech into text, on the text itself, somewhere further when changing systems. But to assess how adequately the system responded is already more difficult: in fact, what is an “adequate response”? There are also all sorts of "cherries on the cake" like cases when a user addresses the system as "you"and she answers "you" - is it good or bad? It seems to be bad, the assistant is a subordinate figure.
In general, there are a lot of small chips that are difficult to take into account in quantitative measurements.
Is there an intermediate, common language for simplified processing and then switching to the desired language?
This is a great question, but it should not be me who should be asking it, but ABBYY representatives who have been trying to do just that for many years. That is, in linguistics there is the idea that all languages ​​are built in a similar way, and you can create a universal grammar, dictionary and everything else to get a middle link between all languages. Then one could translate from one language into a meta-language and then into any other language. A lot of man-years were spent on this task, but it turned out - despite the beauty of the idea - that the languages ​​are still quite different, and it is almost impossible to carry out such a mapping. And it is not clear how to do this automatically - not by hand. As a result, the topic died out.
It turned out that if you pour in a lot of data and run a neural network, then machine translation turns out to be of sufficient quality and without a meta-language. Neural networks are good at calculating patterns, including grammatical ones, therefore, if the amount of data is sufficient, then they themselves cope without prompts from an intermediate link. In machine translation, everything works well when there are good parallel texts. For example, there are many parallel texts between Russian and English, but there are none at all between Russian and some American Indian language - but there are many parallel texts between it and English, that is, English can play the role of an intermediate language. Often a translation from Russian is done first into English, then into a third language; this technique is common enoughso that English in practice replaces the same meta-language - after all, the most translated data is into and from English. Quality suffers, of course, but it's better than nothing. If there is nothing to train the system on, then it is better to train on such a step-by-step translation than to do nothing.
In general, from a theoretical point of view, the idea is beautiful, but in practice English is used.
Can you give examples of problems you are working on at Google? What is the percentage of interesting and routine tasks?
When I launched the Russian Assistant, there were a lot of routine tasks - simply because the algorithms are universal, and most of the work was reduced to manually fixing bugs and preparing Russian language data. We checked the quality, sometimes we wrote the data by hand; it sounds very sad, but we could not use user data, and we had to take it from somewhere. In order to take data, you could also write a rule and generate data; or open data. Interestingly enough, it was possible to deal with morphology, to see that the generation and understanding of the text were cleverer and it was not necessary to write out all forms of the word "alarm clock" in a column. Unfortunately, there were a lot of “run-see-quality-fix-again-run-correct data-and so on” cycles; It was fun at first, but quickly became routine. Now,since I'm more in research, we make our own agenda, in a sense. I'm just creating new data that can be useful in the future for metrics, and researching how people talk to each other and to assistants to understand what signals can be used to train models. We analyze quality. Part of the work that I am currently doing is product, we are trying to create a roadmap of problems in dialog interactions between a person and an assistant, to understand how to classify it, to understand what we can solve now, what later. That is, now I have almost no routine tasks left, and I am satisfied with my setup.which could be useful in the future for metrics, and researching how people talk to each other and to assistants to understand what signals can be used to train models. We analyze quality. Part of the work that I am currently doing is product, we are trying to create a roadmap of problems in dialog interactions between a person and an assistant, to understand how to classify it, to understand what we can solve now, what later. That is, now I have almost no routine tasks left, and I am satisfied with my setup.which could be useful in the future for metrics, and researching how people talk to each other and to assistants to understand what signals can be used to train models. We analyze quality. Part of the work that I am currently doing is product, we are trying to create a roadmap of problems in dialog interactions between a person and an assistant, to understand how to classify it, to understand what we can solve now, what later. That is, now I have almost no routine tasks left, and I am satisfied with my setup.we are trying to create a roadmap of problems in dialogue interactions between a person and an assistant, to understand how to classify it, to understand what we can solve now, what later. That is, now I have almost no routine tasks left, and I am satisfied with my setup.we are trying to create a roadmap of problems in dialogue interactions between a person and an assistant, to understand how to classify it, to understand what we can solve now, what later. That is, now I have almost no routine tasks left, and I am satisfied with my setup.
This balance looks different for different linguists. We recently had an anniversary - 100 linguists in the company, now there are a little more. It's cool, because when I joined the company 4 years ago, there were 30 of us. Our demand is definitely growing.
Do you use context definition to analyze texts?
I do not know if the author of the question is still looking - reformulate, please, I did not understand.
We're using context, of course; without it there is no dialogue interaction. Of course, we are trying to solve problems so that the user, for example, does not say “alarm clock” every time, but can use natural pronouns (“set the alarm to 7, no, change it to 8”). This already works well for English; I don’t remember if it was already launched for Russian.
Why hasn't the Russian language column been launched?
From what I can tell, Google has a lot of different languages. Priorities should be arranged according to the volume and value of markets, and the Russian market is not the most interesting. It is a little stimulating that there is Yandex in Russia, and you can compete with it, but from a practical point of view, nobody wants to. Plus, Google has closed development offices in Russia after the adoption of the law on personal data.
Are there open libraries for speech recognition and generation, data arrays for training neural networks? How open is this technology?
Yes, full of open source algorithms. Google recently released Bert, a super-new library; now conferences are full of updates to it, which are called various funny words, inside which there is "Bert" (Albert). This is all open source, made by our excellent scientists in Berlin. You can train anything; scientific communities have data on which to train neural networks and see what happens. As I said, these data are not enough for Russian, more for English, so everyone has fun with him.
That is, you do not have user log texts?
We cannot read user logs. The only exceptions are those cases when users are complaining and they are specifically asked for permission to share the last so many statements. But even in such cases, we see only a retelling of the problem from specially trained supports and aggregated statistics of the form "the assistant answered that he does not understand, such and such a percentage of times." Google takes user data very carefully. Part of what my team is doing is thinking about how to generate realistic data or collect it from specially paid people. This is an important direction for all industrial teams because privacy should never be violated. We have to look for new methods of data collection, write new tools;I've been doing this pretty closely for the past six months. Unfortunately, the details have not yet been published. We wanted to write an article for the conference, but the epidemic got in the way. When you work in a company, you, in principle, need an article only in order to go to a conference at the expense of the company and hang out. And now all conferences have gone online, and you won't be able to hang out - the motivation to write an article has really disappeared. Now the epidemic will end, and we will do it anyway.Now the epidemic will end, and we will do it.Now the epidemic will end, and we will do it anyway.
, , ?
I won't show you any test items, but I can tell you about the interview. As I said, we now have 100 linguists, and interviews now take place in the format known to engineers - an interview in the pool. We interview linguists in a row, and when there is a good linguist, we look where there is a vacancy and assign them to teams. We have linguists of various profiles; someone programs, someone practically does not program. I'm from the second category: I'm more in the research part, I don't train models myself - there are guys in the team who do this. We now have 8 teams of linguists of different sizes, and we also have an internalization team, in which I was a specialist in the Russian language: these are people who monitor the quality of the assistant (now I am just a linguist-researcher and I do tasks not related to a specific language) ...Depending on their profile, linguists fall into one of these teams.
Where tasks are for a specific language, speakers of specific languages ​​are needed; they receive special assignments in this language - we are trying to find out if the person understands the features well. For example, if we were hiring for a position with the Russian language (now it does not exist, however), we would ask why Russian is more complicated than English, what methods exist to overcome these difficulties, how Russian morphology works, what is needed for a computer to understand this, how it affects by the amount of data. If the role is general, then we will ask about how well a person understands the current realities of linguistics, how much he can think about algorithms. Although I hardly ever program myself, I understand well how Machine Learning works, what is needed to train the system, about signals, supervised / unsupervised learning, and so on. Actually, this is whatwhich I usually ask in interviews. The most common example: “How would you fix typos? There is an infinite amount of money and developers, but there is no product yet - how to build it from a linguistic point of view, what are the steps? " In general, you can ask about any component that includes natural language. How is it built, according to the respondent? How is a person going to build a similar one, what problems does he see? How to transfer the experience from English to Chinese, from Chinese to Russian, from Russian to Hindi? How will he organize his work with a language that he does not know? There are a lot of options.what are the steps? " In general, you can ask about any component that includes natural language. How is it built, according to the respondent? How is a person going to build something similar, what problems does he see? How to transfer the experience from English to Chinese, from Chinese to Russian, from Russian to Hindi? How will he organize his work with a language that he does not know? There are a lot of options.what are the steps? " In general, one can ask about any component that includes natural language. How is it built, according to the respondent? How is a person going to build a similar one, what problems does he see? How to transfer the experience from English to Chinese, from Chinese to Russian, from Russian to Hindi? How will he organize his work with a language that he does not know? There are a lot of options.
Do you monitor user behavior: what command did the user give, what action was taken by the device?
We cannot monitor user behavior. We can only come up with methods to simulate it and test how the system responds. This is what we are actually doing: trying to figure out how to measure it, and trying to collect data to train the algorithms.
How is the context of the conversation tracked? How much memory is used for this? Is there persistence between sessions?
Is there a lot of memory use - I don't know the details, it's not even for the department where I work. Our department is more concerned with quality, but there are departments that look to see if there is enough memory, if there are leaks, if the assistant stupid for 10 minutes to set the alarm.
We keep track of the context. So far, unfortunately, only within one session. This is one of the tasks that our department - my team, neighboring engineering teams - will be solving over the next year. A very cool and interesting task: how long to keep the context, at what point to consider that the conversation is over, whether to keep the user's context always, what information about the user the system will store in itself. Relatively speaking, if a user says “I hate pizza,” then it’s probably good to keep this context forever and ask once a year if the situation has changed. And, if the user answers that he hasn't changed, do not offer pizza delivery. While assistants do not know how to do this - unfortunately, they are still very far from perfect, they would need many more different contexts.
Now we can understand pronouns within one session (“set the alarm, set it at 8 am”). We are working to expand this context, and separately conduct research to understand what context is useful, how much is needed, where and how much to store it. Of course, Google has many servers, so we don't need to save money, but we want the assistant not to process each request for 3 hours. It's pretty fast now, but not perfect; and if we make it perfect, we would like it to continue to work quickly.
Do you have competition between teams?
Yes and no. Of course, according to how the work inside Google is arranged, you can make a separate broadcast. We have a lot of local initiative; people can try to make prototypes of anything, suggest it to the bosses - bosses are always very open, and they will propose to the top bosses. Quite a lot of projects arise from the fact that someone tried to do something, he succeeded, and it was decided to do it in a quality suitable for release. Of course, there are situations when in 5 places at the same time people come to the same idea, and 10 prototypes are obtained. At such a moment, sometimes you have to choose one of the prototypes, but usually they try to unite people into a team so that they can develop a new feature together, release it into production, and everyone was happy.
So, there is competition, but we try to keep the competition healthy, so that there is not too much politics. Overall, the atmosphere is great. We are now sitting between research and production, bringing ideas, we are in the epicenter with product managers, and with projects, and with everyone - everyone is coordinated and cooperated. Chaos, but everything is spiritual.
What will happen faster - will they make an intelligent voice assistant that will understand no worse than a person, or will Elon Musk invent a neural interface?
I do not know anything about the stage at which the neural interface is. But a smart voice assistant who will understand as well as a person is still very far away. So far, no one understands how to make the assistant understand. All chatters on powerful neural networks are imitation. I saw a question about the Turing test - this is also a test for imitation, for how well the system pretends to be a person and pretends that it understands, but after all, no system understands anything. There was the chatbot Eugene Goostman, which won one of the tests - passed a certain percentage of the judges who had to be tricked. He pretended to be a 15-year-old boy from Odessa with his dad, a gynecologist and a guinea pig (not a joke - he was talking about himself). The bot kept the context a little, asking: "Where are you from?" - and remembered the city from the answer,and then used the memorized after a while, causing a wow effect. Although now it's not too difficult to create a wah effect with voice assistants; they are not perfect. In addition, since the competition was in English, the irregularities in the bot's speech were attributed to the fact that he was "from Odessa" - they believed in a foreign boy.
The question is what to call “understanding”, what is “understanding as good as a human,” and what we basically want from chat bots. Nobody knows the answer to this question either. Do we want him to chat with us like a best friend, or for him to steadily control the smart home and the car and set alarms? What can he do? Can he call us names and taunt us? It's not that our best friends all call names and scoff, but there are special ways to communicate with loved ones. Perhaps we would not want the system to talk to us nicely and hound jokes?
What happened before
- Ilona Papava, Senior Software Engineer at Facebook - how to get an internship, get an offer and everything about working in a company
- Boris Yangel, Yandex ML-engineer - how not to join the ranks of dumb specialists if you are a Data Scientist
- , EO LastBackend — , 15 .
- , Vue.js core team member, GoogleDevExpret — GitLab, Vue Staff-engineer.
- , DeviceLock — .
- , RUVDS — . 1. 2.
- , - . — .
- , Senior Digital Analyst McKinsey Digital Labs — Google, .
- «» , Duke Nukem 3D, SiN, Blood — , .
- , - 12- — ,
- , GameAcademy — .
- , PHP- Badoo — Highload PHP Badoo.
- , CTO Delivery Club — 50 43 ,
- , Doom, Quake Wolfenstein 3D — , DOOM
- , Flipper Zero —

