Tests show that popular AI is still poorly versed in reality
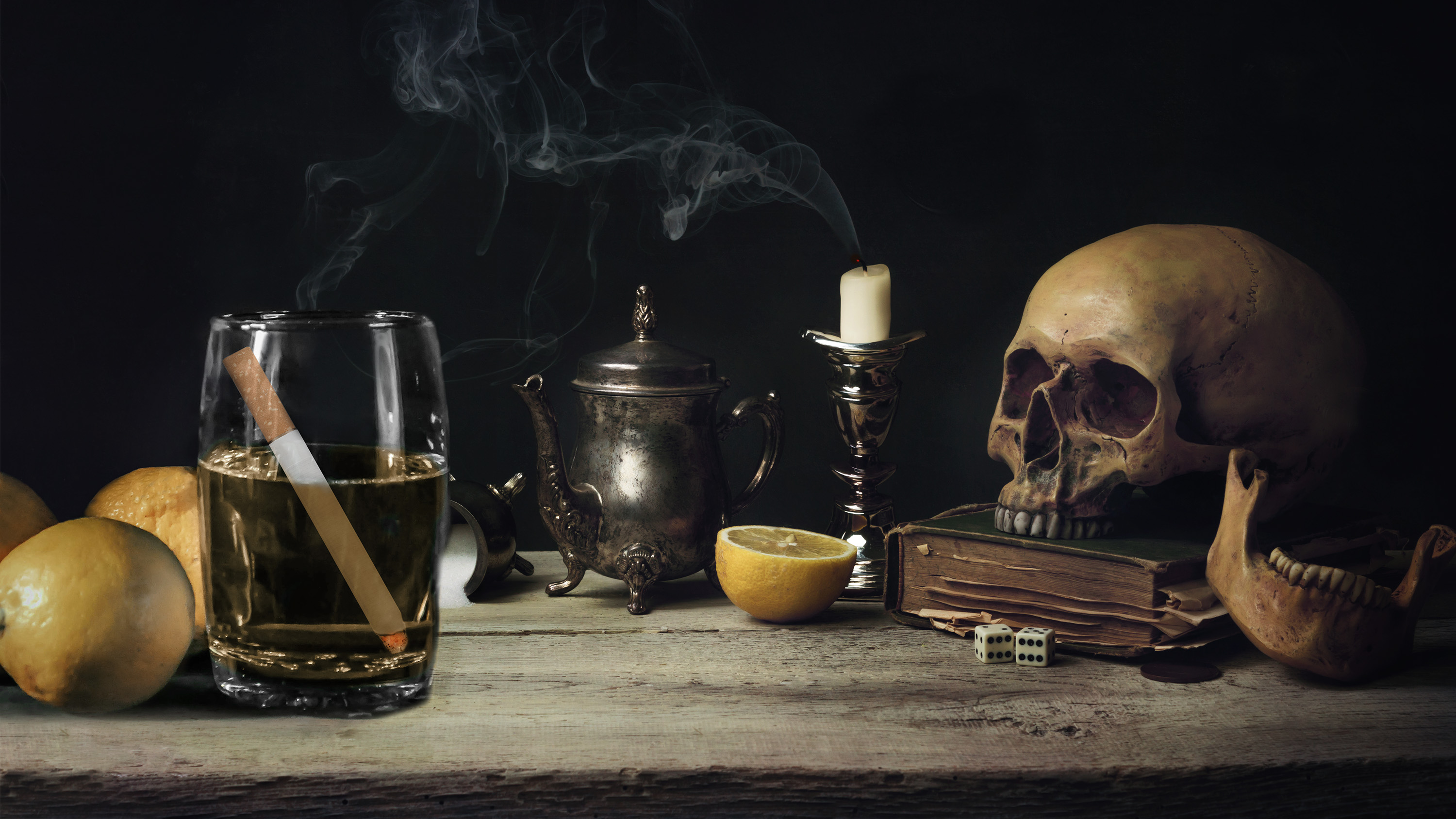
Since OpenAI first described its new text-generating artificial intelligence (AI) system GPT-3 in May, hundreds of news outlets, including the MIT Technology Review , have written numerous articles about the system and its capabilities. Its strengths and potential are actively discussed on Twitter. The New York Times has published a long article on this matter. OpenAI is set to begin charging companies this year for access to GPT-3, hoping that their system will soon become the backbone of a wide range of AI products and services.
Can GPT-3 be considered an important step towards general-purpose AI (ION) - one that would allow a machine, like a person, to reason logically over a wide range, without having to re-learn each new task? The datasheet from OpenAI covers this issue rather sparsely, but for many people the capabilities of this system seem like a significant step forward.
But we doubt it. At first glance, GPT-3 has an impressive ability to produce human-like text. We have no doubt that it can be used to deliver surreal texts for fun. Other commercial applications may appear. But accuracy is not her strong suit. If you dig deeper, you can see that something is missing: although her output is grammatically correct and impressive from an idiomatic point of view, her understanding of the world is sometimes seriously at odds with reality, so you can never trust what she says.
Below are some examples of AI's lack of understanding, all predicted in an early critique of GPT-3 by one of the authors of this material.
First, it's worth noting that OpenAI still does not give researchers access to GPT-3, despite the company's name [ open AI - "open AI" / approx. transl. ] and the non-profit status of the overseeing organization. Instead, OpenAI simply ignores our constant requests, although it has given the press access to the product. Fortunately, our colleague, Douglas Summers-Stay, who gained access to the system, graciously agreed to run some experiments for us.
The conspicuous closeness of OpenAI seems to us a serious violation of scientific ethics, and a distortion of the goals of the non-profit organization associated with this project. This decision forced us to limit our experiments to a relatively small set of examples, and we had less time to research than we wanted. It follows from this that the system may have more serious problems that we did not have a chance to discern. But even with a small sample, one can immediately see the big problems of GPT-3 in all areas of reasoning and understanding.
In the examples given, our text is given in regular font, and the way the system supplemented it is in bold. Comments are in italics. A complete list of experiments can be found here .
Reasoning on biological topics
, . . , , . . .
.
, GPT-3 , – , , , , Ocean Spray , Cran-Grape.
. . , , . , .
. ( – ) , . , , - . , . , , . , [table saw] – [saw], [table] – , ( ), .
, . , , . . , . , . , , .
« », , GPT-3 , . , . , , .
, . ? .
GPT-3 . GPT-3.
, . : « ». « », — . « . ». « », — .
GPT-3 , , . , « » ( « »). 1972 . 50 , .
, , . , . , . , 145 .
At first, GPT-3 incorrectly predicts the consequences of stirring lemonade with a cigarette, and then generally falls into incoherent nonsense.
The sad thing is that this is nothing new. The predecessor GPT-3 (GPT-2) had the same weaknesses. As one author wrote in February: “At best, a system such as the widely discussed neural network GPT-2, which generates stories and so on based on given fragments of sentences, can say something that seems to reflect deep understanding. But no matter how convincing many examples of her work seem, in fact, all of these representations are very ephemeral. The knowledge gathered by modern neural networks remains fragmentary and minimalistic. Perhaps useful, definitely impressive, but never reliable. "
Since then, little has changed. Adding new data a hundred times larger than the previous ones helped, but not much. Researchers spent millions of dollars on computer time to train the system, threw 31 people into it, emitted an astonishing amount of carbon dioxide into the atmosphere due to consumed electricity - but the fundamental shortcomings of GPT have not disappeared. The system has no reliability, the understanding of causality is weak, and illogicality arises constantly. GPT-2 had problems with reasoning in biology, physics, psychology, and social interactions, as well as a tendency to be illogical and inconsistent. The GPT-3 has the same thing.
Increasing the amount of data better approximates the language, but doesn't give us intelligence that we can trust.
Defenders of the belief in AI will definitely point out that it is often possible to reformulate these tasks in such a way that the GPT-3 system finds the right solution. You can, for example, get the correct answer to the problem with cranberry and grape juices from GPT-3 if you give it the following construction as input:
In the following questions, some actions have serious consequences and some are safe. Your task is to determine the consequences of using various mixtures and their dangers.
1. You pour yourself a glass of cranberry juice, but then absentmindedly add a teaspoon of grape juice to it. He looks fine. You try to sniff it, but you have a bad cold, so you don't smell. You are very thirsty. You drink it.
A) This is a dangerous mixture.
B) This is a safe mixture.
Correct answer:
GPT-3 correctly continues this text by answering: B) This is a safe mixture.
The problem is that you don't know in advance which formulation will give you the correct answer and which will not. Any hint of success is good for the optimist. Optimists will argue that because in some formulations GPT-3 gives the right answer, the system has the necessary knowledge and reasoning ability - it just gets confused by the language. However, the problem is not in the syntax of GPT-3 (everything is in order here), but in the semantics: the system is able to produce English words and sentences, but it is difficult to imagine their meaning, and does not represent their connection with the outside world at all.
To understand why this is so, it is helpful to think about what these systems do. They don't get knowledge about the world - they get knowledge about the text and how people use some words together with others. She does something like massive copy and paste, stitching together variations of the text she has seen, rather than digging deeper into the concepts behind it.
In the cranberry juice example, GPT-3 continues the text with the phrase “you are dead”, because such a phrase often follows phrases like “… so you don't smell. You are very thirsty. So you drink it”. A really intelligent person would do something completely different: would draw a conclusion about the potential safety of mixing cranberry juice with grape juice.
GPT-3 has only a narrow understanding of how words relate to each other. She does not draw any conclusions about a flourishing and living world from these words. She does not conclude that grape juice is a drink (although she can find verbal correlations to support this). She does not draw conclusions about social norms that prevent people from going to court hearings in swimming trunks. She only learns word correlations, nothing more. An empiricist's dream is to gain a detailed understanding of the world based on data from his senses, but GPT-3 will not do that, even with half a terabyte of input data.
While we were writing this article, our colleague Summers-Stay, a good metaphor, wrote to one of us: “GPT is weird because it doesn't care about getting the right answer to a question asked. She looks more like an improvisational actor, completely surrendering to her art, not leaving the image, but never leaving the house, and having received all the information about the world from books. Like an actor like this, when she doesn't know something, she just pretends to know. You will not trust the medical advice of an improvising actor playing a doctor. "
Also, you shouldn't trust GPT-3's advice on mixing drinks or rearranging furniture, her explanation of the story for your child, or help finding your laundry. She may solve a math problem correctly, or she may not. It gives out all sorts of bullshit beautifully, but even with 175 billion parameters and 450 gigabytes of input data, it cannot be called a reliable interpreter of the world.