In the first part of the article, I described the background for the study, its goals, assumptions, inputs and tools. Now we can say Gagarin's without further ado ...
Go!
We import the libraries and define the path to the directory with all the files:
import pandas as pd, numpy as np
#
ROOT_FOLDER = r'c:\_PROG_\Projects\us_crimes'
Death at the hands of the law
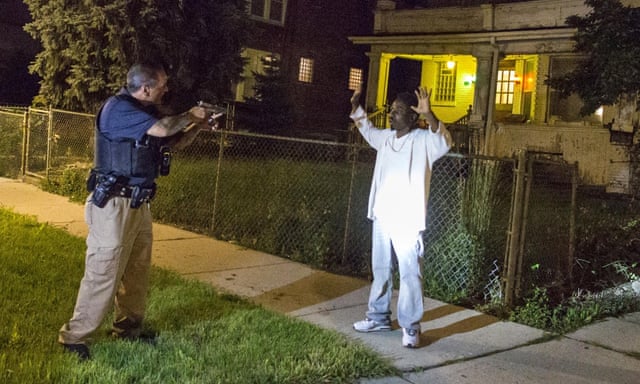
Let's start by analyzing data on police casualties. Let's upload a file from CSV to DataFrame:
# Fatal Encounters (FENC)
FENC_FILE = ROOT_FOLDER + '\\fatal_enc_db.csv'
# DataFrame
df_fenc = pd.read_csv(FENC_FILE, sep=';', header=0, usecols=["Date (Year)", "Subject's race with imputations", "Cause of death", "Intentional Use of Force (Developing)", "Location of death (state)"])
, , : , ( ), ( , ), , .
, " " . , , FENC , , . , ( ). Fatal Encounters Excel ( ).
:
df_fenc.columns = ['Race', 'State', 'Cause', 'UOF', 'Year']
df_fenc.dropna(inplace=True)
, . . FENC, , (Hispanic/Latino), (Asian/Pacific Islander) (Middle Eastern). . :
df_fenc = df_fenc.replace({'Race': {'European-American/White': 'White', 'African-American/Black': 'Black',
'Hispanic/Latino': 'White', 'Native American/Alaskan': 'American Indian',
'Asian/Pacific Islander': 'Asian', 'Middle Eastern': 'Asian',
'NA': 'Unknown', 'Race unspecified': 'Unknown'}}, value=None)
( ) :
df_fenc = df_fenc.loc[df_fenc['Race'].isin(['White', 'Black'])]
"UOF" ( )? , ( ) . , (, ) , . : 1) - (: , ; : ); 2) ; , , ( ) , . , :
df_fenc = df_fenc.loc[df_fenc['UOF'].isin(['Deadly force', 'Intentional use of force'])]
. CSV, :
df_state_names = pd.read_csv(ROOT_FOLDER + '\\us_states.csv', sep=';', header=0)
df_fenc = df_fenc.merge(df_state_names, how='inner', left_on='State', right_on='state_abbr')
df_fenc.head()
, :
Race | State | Cause | UOF | Year | state_name | state_abbr | |
---|---|---|---|---|---|---|---|
0 | Black | GA | Gunshot | Deadly force | 2000 | Georgia | GA |
1 | Black | GA | Gunshot | Deadly force | 2000 | Georgia | GA |
2 | Black | GA | Gunshot | Deadly force | 2000 | Georgia | GA |
3 | Black | GA | Gunshot | Deadly force | 2000 | Georgia | GA |
4 | Black | GA | Gunshot | Deadly force | 2000 | Georgia | GA |
, :
#
ds_fenc_agg = df_fenc.groupby(['Year', 'Race']).count()['Cause']
df_fenc_agg = ds_fenc_agg.unstack(level=1)
# UINT16
df_fenc_agg = df_fenc_agg.astype('uint16')
2 : White ( ) Black ( ), ( 2000 2020). :
# (- )
plt = df_fenc_agg.plot(xticks=df_fenc_agg.index, color=['olive', 'g'])
plt.set_xticklabels(df_fenc_agg.index, rotation='vertical')
plt.set_xlabel('')
plt.set_ylabel('- ')
plt

:
() , .
2.4 . , . , .
( ):
# CSV (1991 - 2018)
POP_FILE = ROOT_FOLDER + '\\us_pop_1991-2018.csv'
df_pop = pd.read_csv(POP_FILE, index_col=0, dtype='int64')
:
# - 2000 - 2018 .
df_pop = df_pop.loc[2000:2018, ['White_pop', 'Black_pop']]
# ,
df_fenc_agg = df_fenc_agg.join(df_pop)
df_fenc_agg.dropna(inplace=True)
#
df_fenc_agg = df_fenc_agg.astype({'White_pop': 'uint32', 'Black_pop': 'uint32'})
. 2 , ( 1 . ):
df_fenc_agg['White_promln'] = df_fenc_agg['White'] * 1e6 / df_fenc_agg['White_pop']
df_fenc_agg['Black_promln'] = df_fenc_agg['Black'] * 1e6 / df_fenc_agg['Black_pop']
, :
Black | White | White_pop | Black_pop | White_promln | Black_promln | |
---|---|---|---|---|---|---|
Year | ||||||
2000 | 148 | 291 | 218756353 | 35410436 | 1.330247 | 4.179559 |
2001 | 158 | 353 | 219843871 | 35758783 | 1.605685 | 4.418495 |
2002 | 161 | 363 | 220931389 | 36107130 | 1.643044 | 4.458953 |
2003 | 179 | 388 | 222018906 | 36455476 | 1.747599 | 4.910099 |
2004 | 157 | 435 | 223106424 | 36803823 | 1.949742 | 4.265861 |
2005 | 181 | 452 | 224193942 | 37152170 | 2.016112 | 4.871855 |
2006 | 212 | 460 | 225281460 | 37500517 | 2.041890 | 5.653255 |
2007 | 219 | 449 | 226368978 | 37848864 | 1.983487 | 5.786171 |
2008 | 213 | 442 | 227456495 | 38197211 | 1.943229 | 5.576323 |
2009 | 249 | 478 | 228544013 | 38545558 | 2.091501 | 6.459888 |
2010 | 219 | 506 | 229397472 | 38874625 | 2.205778 | 5.633495 |
2011 | 290 | 577 | 230838975 | 39189528 | 2.499578 | 7.399936 |
2012 | 302 | 632 | 231992377 | 39623138 | 2.724227 | 7.621809 |
2013 | 310 | 693 | 232969901 | 39919371 | 2.974633 | 7.765653 |
2014 | 264 | 704 | 233963128 | 40379066 | 3.009021 | 6.538041 |
2015 | 272 | 729 | 234940100 | 40695277 | 3.102919 | 6.683822 |
2016 | 269 | 723 | 234644039 | 40893369 | 3.081263 | 6.578084 |
2017 | 265 | 743 | 235507457 | 41393491 | 3.154889 | 6.401973 |
2018 | 265 | 775 | 236173020 | 41617764 | 3.281493 | 6.367473 |
2 - . :
plt = df_fenc_agg.loc[:, ['White_promln', 'Black_promln']].plot(xticks=df_fenc_agg.index, color=['g', 'olive'])
plt.set_xticklabels(df_fenc_agg.index, rotation='vertical')
plt.set_xlabel('')
plt.set_ylabel('- \n 1 ')
plt

:
df_fenc_agg.loc[:, ['White_promln', 'Black_promln']].describe()
White_promln | Black_promln | |
---|---|---|
count () | 19.000000 | 19.000000 |
mean ( .) | 2.336123 | 5.872145 |
std (. ) | 0.615133 | 1.133677 |
min (. ) | 1.330247 | 4.179559 |
25% | 1.946485 | 4.890977 |
50% | 2.091501 | 5.786171 |
75% | 2.991827 | 6.558062 |
max (. ) | 3.281493 | 7.765653 |
:
1. 5.9 1 . 2.3 1 . ( 2.6 ).
2. () 1.8 , . ( , , .)
3. - 2013 . (7.7 ); - 2018 . (3.3 ).
4. ( 0.1 - 0.2 ), 2009 . 2011 - 2013 .
, :
- , , ?
- , . 2.6 , .
, - , , .
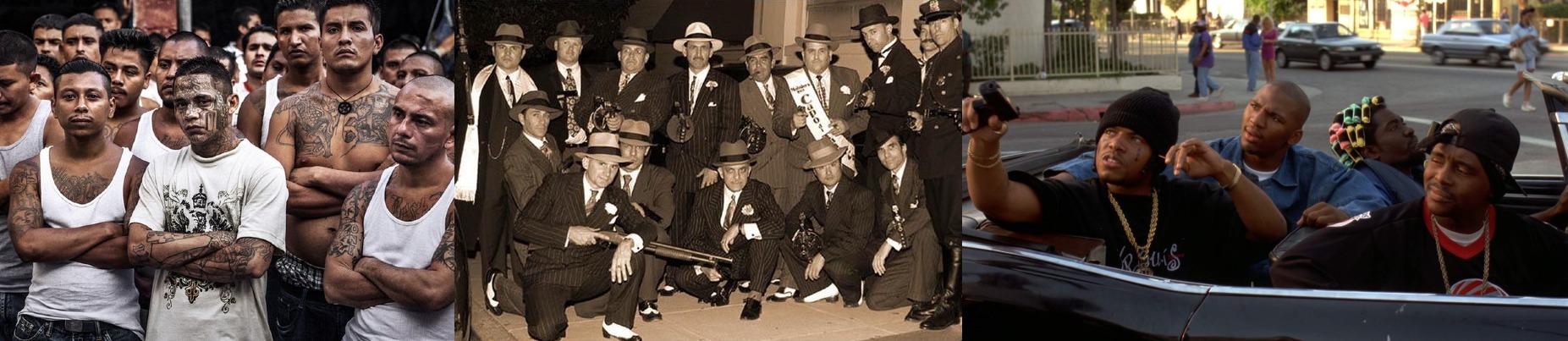
CSV :
CRIMES_FILE = ROOT_FOLDER + '\\culprits_victims.csv'
df_crimes = pd.read_csv(CRIMES_FILE, sep=';', header=0, index_col=0, usecols=['Year', 'Offense', 'Offender/Victim', 'White', 'White pro capita', 'Black', 'Black pro capita'])
- : , , , ( - "White", "Black" - "White pro capita", "Black pro capita").
(`df_crimes.head()`):
Offense | Offender/Victim | Black | White | Black pro capita | White pro capita | |
---|---|---|---|---|---|---|
Year | ||||||
1991 | All Offenses | Offender | 490 | 598 | 1.518188e-05 | 2.861673e-06 |
1991 | All Offenses | Offender | 4 | 4 | 1.239337e-07 | 1.914160e-08 |
1991 | All Offenses | Offender | 508 | 122 | 1.573958e-05 | 5.838195e-07 |
1991 | All Offenses | Offender | 155 | 176 | 4.802432e-06 | 8.422314e-07 |
1991 | All Offenses | Offender | 13 | 19 | 4.027846e-07 | 9.092270e-08 |
. :
# ( )
df_crimes1 = df_crimes.loc[df_crimes['Offender/Victim'] == 'Offender']
# (2000-2018)
df_crimes1 = df_crimes1.loc[2000:2018, ['Offense', 'White', 'White pro capita', 'Black', 'Black pro capita']]
(1295 * 5 ):
Offense | White | White pro capita | Black | Black pro capita | |
---|---|---|---|---|---|
Year | |||||
2000 | All Offenses | 679 | 0.000003 | 651 | 0.000018 |
2000 | All Offenses | 11458 | 0.000052 | 30199 | 0.000853 |
2000 | All Offenses | 4439 | 0.000020 | 3188 | 0.000090 |
2000 | All Offenses | 10481 | 0.000048 | 5153 | 0.000146 |
2000 | All Offenses | 746 | 0.000003 | 63 | 0.000002 |
... | ... | ... | ... | ... | ... |
2018 | Larceny Theft Offenses | 1961 | 0.000008 | 1669 | 0.000040 |
2018 | Larceny Theft Offenses | 48616 | 0.000206 | 30048 | 0.000722 |
2018 | Drugs Narcotic Offenses | 555974 | 0.002354 | 223398 | 0.005368 |
2018 | Drugs Narcotic Offenses | 305052 | 0.001292 | 63785 | 0.001533 |
2018 | Weapon Law Violation | 70034 | 0.000297 | 58353 | 0.001402 |
1 1 ( ). :
df_crimes1['White_promln'] = df_crimes1['White pro capita'] * 1e6
df_crimes1['Black_promln'] = df_crimes1['Black pro capita'] * 1e6
, ( ), :
df_crimes_agg = df_crimes1.groupby(['Offense']).sum().loc[:, ['White', 'Black']]
White | Black | |
---|---|---|
Offense | ||
All Offenses | 44594795 | 22323144 |
Assault Offenses | 12475830 | 7462272 |
Drugs Narcotic Offenses | 9624596 | 3453140 |
Larceny Theft Offenses | 9563917 | 4202235 |
Murder And Nonnegligent Manslaughter | 28913 | 39617 |
Sex Offenses | 833088 | 319366 |
Weapon Law Violation | 829485 | 678861 |
:
plt = df_crimes_agg.plot.barh(color=['g', 'olive'])
plt.set_ylabel(' ')
plt.set_xlabel('- ( 2000 - 2018 )')

, , :
, , " " , ,
, ( 2 " ")
, "" . , :
df_crimes_agg1 = df_crimes1.groupby(['Offense']).sum().loc[:, ['White_promln', 'Black_promln']]
White_promln | Black_promln | |
---|---|---|
Offense | ||
All Offenses | 194522.307758 | 574905.952459 |
Assault Offenses | 54513.398833 | 192454.602875 |
Drugs Narcotic Offenses | 41845.758869 | 88575.523095 |
Larceny Theft Offenses | 41697.303725 | 108189.184125 |
Murder And Nonnegligent Manslaughter | 125.943007 | 1016.403706 |
Sex Offenses | 3633.777035 | 8225.144985 |
Weapon Law Violation | 3612.671402 | 17389.163849 |
:
plt = df_crimes_agg1.plot.barh(color=['g', 'olive'])
plt.set_ylabel(' ')
plt.set_xlabel('- 1 ( 2000 - 2018 )')

. ( ) , . " " 3 .
" " (All Offenses) , ( ) ( - , ).
# 'All Offenses' =
df_crimes1 = df_crimes1.loc[df_crimes1['Offense'] == 'All Offenses']
# , , , :
#df_crimes1 = df_crimes1.loc[df_crimes1['Offense'].str.contains('Assault|Murder')]
#
df_crimes1 = df_crimes1.groupby(level=0).sum().loc[:, ['White_promln', 'Black_promln']]
:
White_promln | Black_promln | |
---|---|---|
Year | ||
2000 | 6115.058976 | 17697.409882 |
2001 | 6829.701429 | 20431.707645 |
2002 | 7282.333249 | 20972.838329 |
2003 | 7857.691182 | 22218.966500 |
2004 | 8826.576863 | 26308.815799 |
2005 | 9713.826255 | 30616.569637 |
2006 | 10252.894313 | 33189.382429 |
2007 | 10566.527362 | 34100.495064 |
2008 | 10580.520024 | 34052.276749 |
2009 | 10889.263592 | 33954.651792 |
2010 | 10977.017218 | 33884.236826 |
2011 | 11035.346176 | 32946.454471 |
2012 | 11562.836825 | 33150.706035 |
2013 | 11211.113491 | 32207.571607 |
2014 | 11227.354594 | 31517.346141 |
2015 | 11564.786088 | 31764.865490 |
2016 | 12193.026562 | 33186.064958 |
2017 | 12656.261666 | 34900.390499 |
2018 | 13180.171893 | 37805.202605 |
:
plt = df_crimes1.plot(xticks=df_crimes1.index, color=['g', 'olive'])
plt.set_xticklabels(df_fenc_agg.index, rotation='vertical')
plt.set_xlabel('')
plt.set_ylabel('- \n 1 ')
plt

:
1. 2 , , , 3 ( ).
2. ( 2 18 ). , : 2001 2006 . , 2007 2016 , 2017 . 2 ( ).
3. 2007-2016 ., , .
, :
- ?
- 3 .
: , " , ?"
- - .
, :
#
df_uof_crimes = df_fenc_agg.join(df_crimes1, lsuffix='_uof', rsuffix='_cr')
# (. )
df_uof_crimes = df_uof_crimes.loc[:, 'White_pop':'Black_promln_cr']
?
White_pop | Black_pop | White_promln_uof | Black_promln_uof | White_promln_cr | Black_promln_cr | |
---|---|---|---|---|---|---|
Year | ||||||
2000 | 218756353 | 35410436 | 1.330247 | 4.179559 | 6115.058976 | 17697.409882 |
2001 | 219843871 | 35758783 | 1.605685 | 4.418495 | 6829.701429 | 20431.707645 |
2002 | 220931389 | 36107130 | 1.643044 | 4.458953 | 7282.333249 | 20972.838329 |
2003 | 222018906 | 36455476 | 1.747599 | 4.910099 | 7857.691182 | 22218.966500 |
2004 | 223106424 | 36803823 | 1.949742 | 4.265861 | 8826.576863 | 26308.815799 |
2005 | 224193942 | 37152170 | 2.016112 | 4.871855 | 9713.826255 | 30616.569637 |
2006 | 225281460 | 37500517 | 2.041890 | 5.653255 | 10252.894313 | 33189.382429 |
2007 | 226368978 | 37848864 | 1.983487 | 5.786171 | 10566.527362 | 34100.495064 |
2008 | 227456495 | 38197211 | 1.943229 | 5.576323 | 10580.520024 | 34052.276749 |
2009 | 228544013 | 38545558 | 2.091501 | 6.459888 | 10889.263592 | 33954.651792 |
2010 | 229397472 | 38874625 | 2.205778 | 5.633495 | 10977.017218 | 33884.236826 |
2011 | 230838975 | 39189528 | 2.499578 | 7.399936 | 11035.346176 | 32946.454471 |
2012 | 231992377 | 39623138 | 2.724227 | 7.621809 | 11562.836825 | 33150.706035 |
2013 | 232969901 | 39919371 | 2.974633 | 7.765653 | 11211.113491 | 32207.571607 |
2014 | 233963128 | 40379066 | 3.009021 | 6.538041 | 11227.354594 | 31517.346141 |
2015 | 234940100 | 40695277 | 3.102919 | 6.683822 | 11564.786088 | 31764.865490 |
2016 | 234644039 | 40893369 | 3.081263 | 6.578084 | 12193.026562 | 33186.064958 |
2017 | 235507457 | 41393491 | 3.154889 | 6.401973 | 12656.261666 | 34900.390499 |
2018 | 236173020 | 41617764 | 3.281493 | 6.367473 | 13180.171893 | 37805.202605 |
, :
White_pop -
Black_pop -
White promln_uof - ( 1 )
Black promln_uof - ( 1 )
White promln_cr - , ( 1 )
Black promln_cr - , ( 1 )
, ... , :)
, . - :)
plt = df_uof_crimes['White_promln_cr'].plot(xticks=df_uof_crimes.index, legend=True)
plt.set_ylabel('- 1 .')
plt2 = df_uof_crimes['White_promln_uof'].plot(xticks=df_uof_crimes.index, legend=True, secondary_y=True, style='g')
plt2.set_ylabel('- 1 .', rotation=90)
plt2.set_xlabel('')
plt.set_xlabel('')
plt.set_xticklabels(df_uof_crimes.index, rotation='vertical')
plt

:

, . , :
plt = df_uof_crimes['Black_promln_cr'].plot(xticks=df_uof_crimes.index, legend=True)
plt.set_ylabel('- 1 .')
plt2 = df_uof_crimes['Black_promln_uof'].plot(xticks=df_uof_crimes.index, legend=True, secondary_y=True, style='g')
plt2.set_ylabel('- 1 .', rotation=90)
plt2.set_xlabel('')
plt.set_xlabel('')
plt.set_xticklabels(df_uof_crimes.index, rotation='vertical')
plt

:

: "", : , .
df_corr = df_uof_crimes.loc[:, ['White_promln_cr', 'White_promln_uof', 'Black_promln_cr', 'Black_promln_uof']].corr(method='pearson')
df_corr.style.background_gradient(cmap='PuBu')
:
White_promln_cr | White_promln_uof | Black_promln_cr | Black_promln_uof | |
---|---|---|---|---|
White_promln_cr | 1.000000 | 0.885470 | 0.949909 | 0.802529 |
White_promln_uof | 0.885470 | 1.000000 | 0.710052 | 0.795486 |
Black_promln_cr | 0.949909 | 0.710052 | 1.000000 | 0.722170 |
Black_promln_uof | 0.802529 | 0.795486 | 0.722170 | 1.000000 |
: = 0.885, = 0.722. , , , ( ), . , , , .
, . ( , , ). : ( 100, %):
# ( )
df_uof_crimes_agg = df_uof_crimes.loc[:, ['White_promln_cr', 'White_promln_uof', 'Black_promln_cr', 'Black_promln_uof']].agg(['mean', 'sum', 'min', 'max'])
# ""
df_uof_crimes_agg['White_uof_cr'] = df_uof_crimes_agg['White_promln_uof'] * 100. / df_uof_crimes_agg['White_promln_cr']
df_uof_crimes_agg['Black_uof_cr'] = df_uof_crimes_agg['Black_promln_uof'] * 100. / df_uof_crimes_agg['Black_promln_cr']
:
White_promln_cr | White_promln_uof | Black_promln_cr | Black_promln_uof | White_uof_cr | Black_uof_cr | |
---|---|---|---|---|---|---|
mean | 10238.016198 | 2.336123 | 30258.208024 | 5.872145 | 0.022818 | 0.019407 |
sum | 194522.307758 | 44.386338 | 574905.952459 | 111.570747 | 0.022818 | 0.019407 |
min | 6115.058976 | 1.330247 | 17697.409882 | 4.179559 | 0.021754 | 0.023617 |
max | 13180.171893 | 3.281493 | 37805.202605 | 7.765653 | 0.024897 | 0.020541 |
:
plt = df_uof_crimes_agg.loc['mean', ['White_uof_cr', 'Black_uof_cr']].plot.bar(color=['g', 'olive'])
plt.set_ylabel(' - - ')
plt.set_xticklabels(['', ''], rotation=0)

, , . , , - .
:
1. ( ). : , .
2. , " " , ( ). , "" ( -> -> -> ).
3. , . .
, :
- , ?
- Yes, such a correlation is observed, although it is heterogeneous across races: for whites it is almost perfect, for blacks it is almost imperfect.
In the next part of the article, we will look at the geographic distribution of the analyzed data across the US states.
Link to the English version of the article (at the request of workers).