Data Scientists find out what people are interested in and what they spend their money on
In the course of researching various audiences, Data Scientists observe both natural and surprising facts that vividly characterize the society around us. In this article I will talk about those curiosities and unusual cases that I noticed when performing tasks related to audit analysis, researching the interests of Internet users and purchasing behavior of various social groups.
What sociological features have been identified through the use of machine learning models? What do we know about customers?

Customer profile on his check? Easy!
I work as a data analyst at CleverDATA and usually face the following tasks: raw data classification, audit analysis and look alike model building (LaL), when the customer has his own audience and he would like to find a similar one. It is very much in demand for various online advertising campaigns.
We have 1DMC DATA Exchange , where members can enrich and monetize their data. It contains depersonalized data of two kinds, aggregated into the attributes of our taxonomy - online purchases and clickstream, that is, the sequence of page visits that we were able to track. The data format meets the European GDPR standard for personal data protection.
The attributes of our taxonomy are the facts of ownership of a thing or the presence of a certain interest in a person. This is binary information - either there or not.
Here are some examples of our taxonomy attributes:
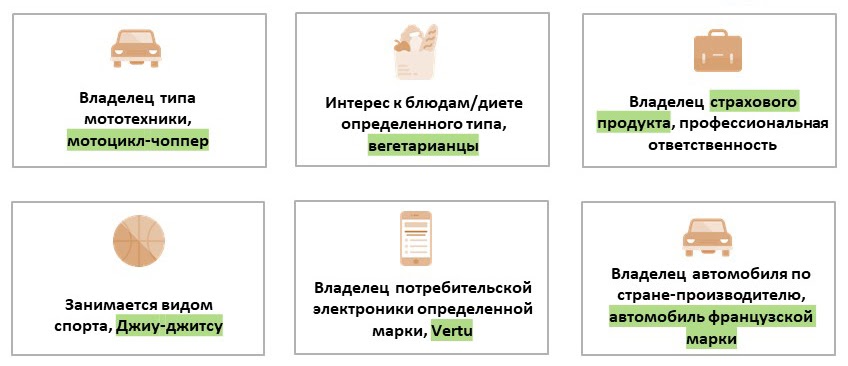
One of the most significant tasks is the aggregation of raw supplier data into taxonomy attributes, that is, the classification task.
I need to draw conclusions on people's purchases about their lifestyle and whether they have certain things (conditionally, a check for a branded street rod model probably indicates that the buyer is the owner of a Harley-Davidson motorcycle) or identify potential interest in purchases from through the Internet pages they visit. This information will then be used for targeted advertising.
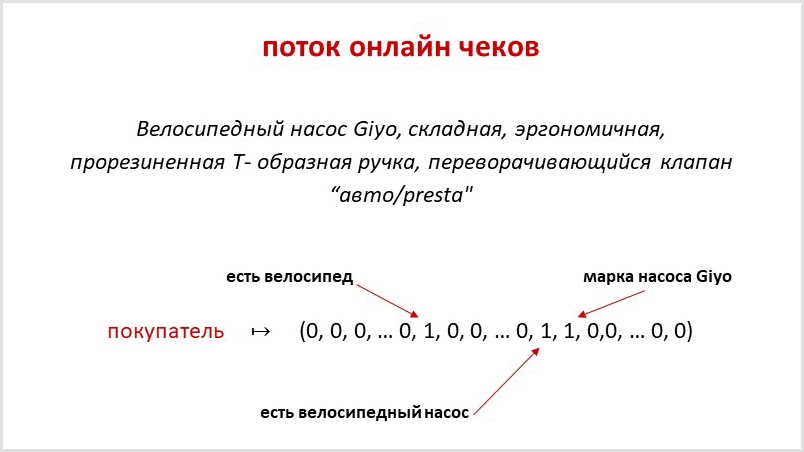
In the course of my work, the following chains appear:
- check - my AI models - buyer profile;
- click stream - my AI models - site visitor profile.
The tool we use in CleverDATA will automatically build a binary classifier for any attribute in our taxonomy. From the very name of the taxonomy attribute (the owner of the chopper motorcycle attribute), we end up with an already automatically evaluated binary classifier (whether the model is good or the analytics needs to be improved), which is able to determine the presence or absence of such an item in a person by check. You can read more about this in our article on Habré .

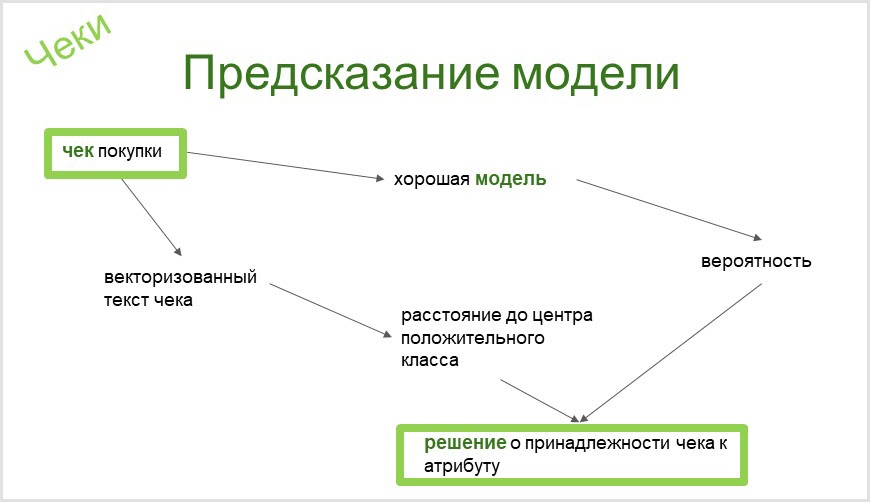
When classifying checks, you need a tool that will allow you to distinguish checks that are similar in words from similar in meaning. So, I somehow built a model to capture interest in professional retraining courses. And she identified the check for the purchase of Paolo Cossi's children's book "A Course in Magic Lessons for an Ordinary Cat" as an interest in the topic. This is, of course, a funny mistake. By the way, I learned about the existence of the book from this check.

To avoid such curiosities, we used language models to evaluate the resulting binary classifiers and cut off those examples that are similar in words, but not in meaning.
From time to time I have to look through receipts with my eyes in order to find some false matches and subsequently automate the search for such mistakenly built connections. It can be helpful to get to the bottom of it because perhaps a single incomprehensible case will allow me to improve the whole process.
Over the course of my entire practice, I have accumulated a whole set of riddle checks that I could not only classify, but even decipher what exactly the buyer purchased. I regularly share these amusing cases with colleagues and even started the "AI jokes" column.
The most common clue is the indication in the check of the title of the book without the name of the product. This is exactly what we see in the case of "magic for an ordinary cat". And what purchases are recorded in the check "Fence Novosibirsk 1029 rubles." and "Contract-box 5000 rubles." I still do not understand. I accept your versions in the comments to this article.
Next, let's move on to the click stream classification.
Client profile by his movements on the website
The clickstream classification system was introduced by us in 2019, which was rich in breakthroughs in the field of NLP (Natural Language Processing). One of the most high-profile and successful inventions in this area is the BERT ( Bidirectional Encoder Representations from Transformers ) network . So there will be a bit of Bertology ahead.

From the name of the attribute, using a probabilistic language model, we get an augmented (extended with synonyms) list of queries that we crawl (send to a search engine and collect search results), hence our training sample is obtained. Let's vectorize it using the pretrained BERT language model. Using the obtained embeddings (vectors), we train the classifier (with the triplet loss function).
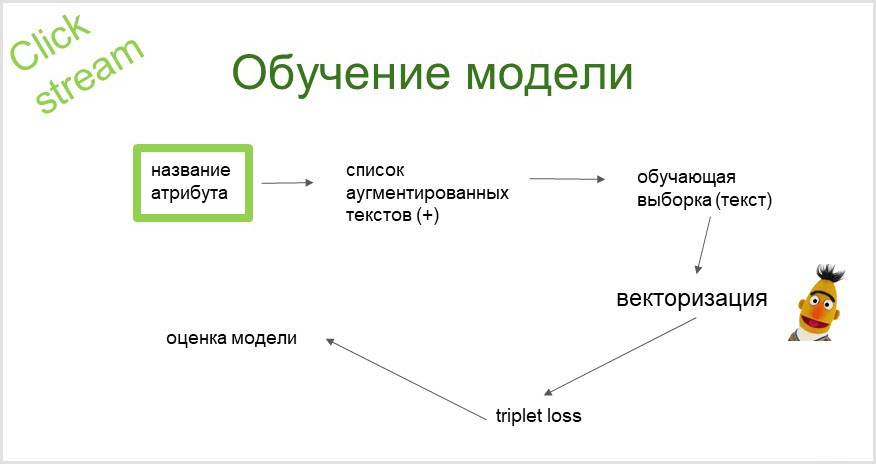
How does the prediction work?
We take the url of the page, collect text information (title and description of the page). With the help of BERT, we get a vector representation of these texts. Then these vectors are fed into the model and at the output we get an attribute to which we can refer the page.
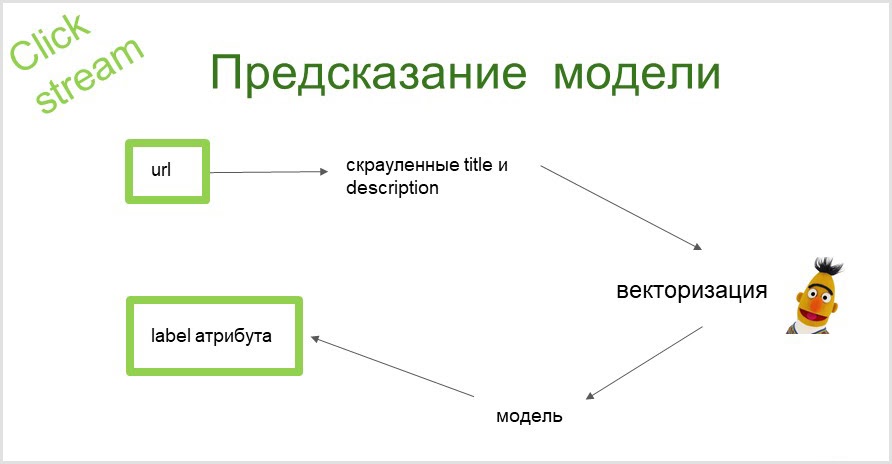
In general, this system is very successful, all the funny cases that I came across are rather exceptions than the rule. But I try to pay great attention to them, because a small error can lead to large unpleasant consequences, since a huge amount of data passes through the system.
Online data that I researched showed that people read more on the Internet. It turned out that this is one of the very popular topics - astrology, fortune-telling, etc.

These specific pages (urls, not domains) were visited by over 5000 thousand people (unique identifiers) per day. I was especially struck by the site dedicated to cat astrology and revealing the connection between the animal's character and its zodiac sign.

Everyone knows about stop words and usually they connect dictionaries or filter by frequencies without going deep into the specifics of texts. At first, I also connected my dictionaries. The result was not pleasing: the site of recipes without using baked goods was classified as an attribute of interest in baking (home baking). And this is due to the fact that all negative particles were present in my stop word dictionary.

Using my example, I urge my colleagues to carefully read the dictionaries with which you filter your data.
Another common problem is that people often use sarcastic language, which, during the crawling stage, leads to funny phrases in the title and description of pages related to certain queries on the Internet. For example, the model can link pasties and interest in a vegetarian diet. It seems to me that this can be explained by the abundance of comments on articles on the topic of vegetarianism in the spirit of "How do you live without pasties?"

And now a minute of black humor in our heading "AI jokes": the model connected the discussion of the legalization of euthanasia with an interest in buying a home, and rapper Timati - with a circus. I had to crawl into the data and manually re-mark the class.
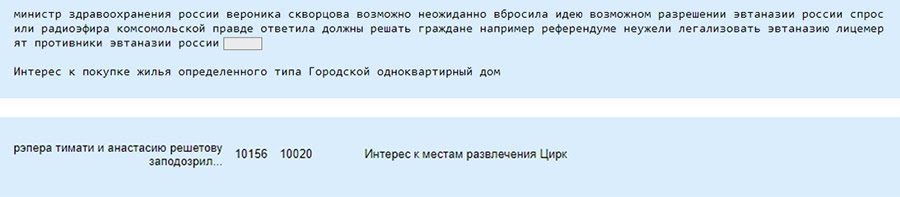
There are setups that we cannot control, they depend on the society in which we live. And then the crime is mixed with comedies and family relationships.
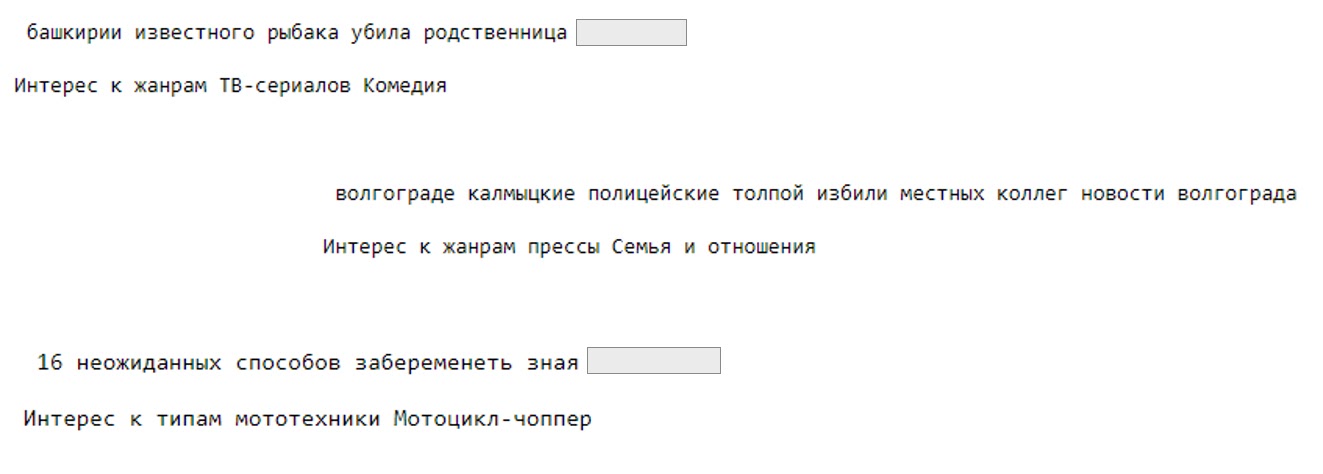
And there are also controversial cases when you do not even know whether it is worth scolding the model and redesigning something, struggling with errors, or leaving everything as it is.

It is possible that the receipt of parcels does carry a business risk.
Anything can be found on the bulletin board.

Search for a similar audience
The next block of tasks that I, as an analyst, have to solve is Audiene Research / Look-alike modeling. The customer, as a rule, wants some new knowledge about the audience, which should help him to establish communication with her. But even if his request is not clearly formulated, we always try to help him, and in most cases we succeed.
Here you have a choice whether to focus on Audience Research, that is, on internal insights (intelligence analysis of the audience), or on a look-alike model, which will then allow you to speed up the audience of our exchange and find potential customers based on the customer's internal data about the target audience. An audience is understood as a set of encoded identifiers (phone numbers, email addresses or online id). I remind you that we do not work with data in an open form, we comply with all the rules of the law.
So, we can cross many encoded identifiers with the exchange and see the buying behavior or their click stream. We do clustering for any target audience and any task. After the model grouped people according to their buying behavior, I somehow saw a cluster of only people who bet on sports and no longer buy anything online. Although, it is possible that they have some kind of separate accounts for bookmaking purposes.
Here is a screenshot of this cluster.
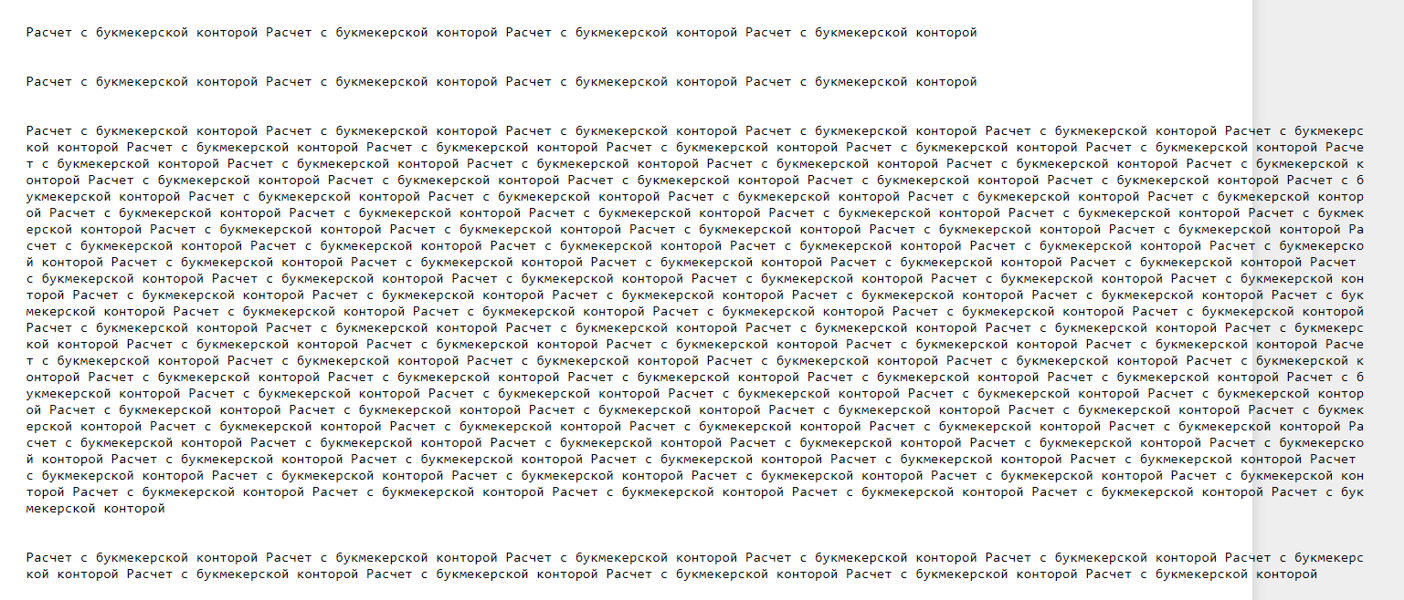
Case "Happy motherhood"
For an advertising campaign for a well-known brand of diapers, it was necessary to conduct an audience research and find women in the third trimester of pregnancy - the customer suggested that it was from the third trimester that the product had to be advertised so that most of the audience would buy it.
At the beginning of the analysis, the description of the circumstances of the pregnant women's life resembled an idyllic picture: a young family with pets, on the eve of the birth of a child, equips housing.
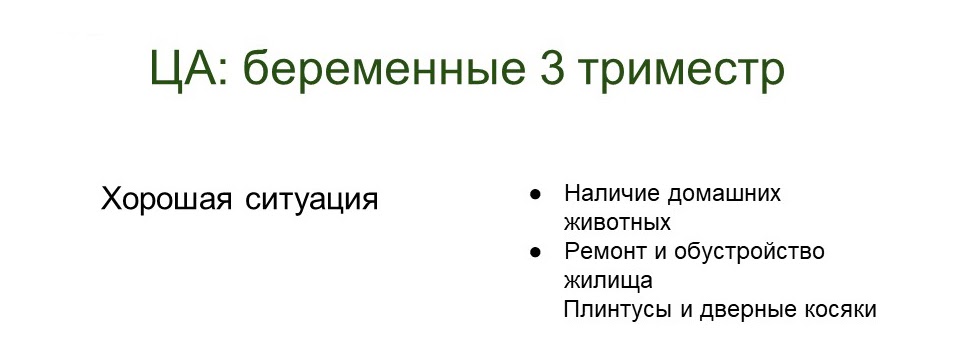
Women from different clusters own gadgets of different brands, prefer different brands of hygiene products, and in general everything is fine. See for yourself.
25.5% of IDs
Buyers of Huggies Elite Soft are three times less likely to buy Pampers and 7 times less likely to buy Lovular products. They use Peligrin brand products. With a high probability (0.6) are the parents of girls. They tend to pay for utilities via the Internet.
25.5% of identifiers are
inclined to pay for communication and insurance services via the Internet. With a high probability (0.6) are dog owners. Buy Helen Harper products. Among consumer electronics, the Xiaomi brand is expressed.
17.5% of identifiers
Ozon Premium users. They buy Philips Avent baby care equipment and are interested in ironing equipment and installations.
Attention, advice for the future: watch out for promotions / brands that create noise in the total volume of data.
Ozon Premium status in many of our clusters turned out to be one of the defining attributes. But targeting the audience of potential diaper buyers only for Ozon Premium is beyond common sense. So I had to strip out the status from all data. Yes, I thus lowered the metrics, but at the same time increased the adequacy of the model. The first place was taken by goods for newborns, and not by the promoted, popular status. It was an experience that taught me to cut off goods that were too significant for the model.
For look-alike modeling, the idea of building several simple classifiers of the target audience (class 1) and generalized (class 0) lies on the surface in order to highlight the target audience.
For example, let's take purchases of the target audience and ten times the volume of random profiles. We bring this information in sequence, purchases. Then we work with the resulting texts (preprocessing): we remove all high-frequency, uninformative words, and bring the rest to the initial form. Next, we build simple classifiers of several different families - linear (Linear SVC, Logistic Regression), "wooden" (RandomForest), etc. - and measure feature importance, that is, the importance of any words according to models. I found threshold values, above which the importance of these signs is inadequate, that is, the sign is too noisy. Before building something automatic, you have to apply common sense and the method of careful glance many times to collect internal statistics and understand which methods work and which don't.
We examined clusters with an idyllic picture on the eve of the birth of a child, but other life stories were also traced. For example, in one of the clusters, potential buyers of diapers for newborns are highly likely (0.65) to have an account on a dating site. This is not an unfounded statement, they pay for services on such sites.
In order for insights to "work", you always have to interpret new knowledge, but this time I do not want to look for the inside story at all - everyone knows about social ill-being and everyday disorder in our country.
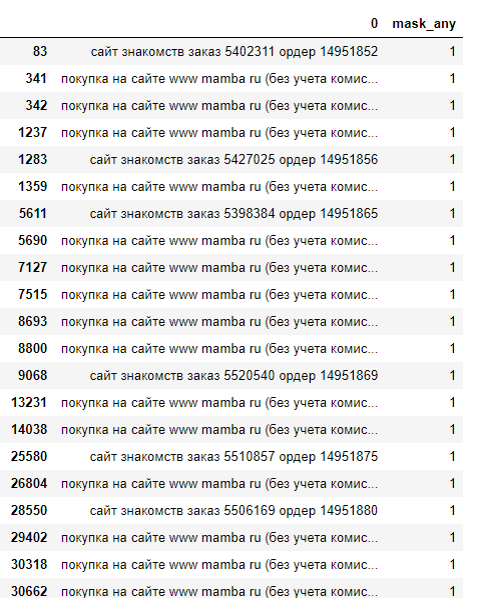
Let me remind you that as part of this case, we researched the entire audience that was interested in buying diapers for newborns. And it turned out not only women in the third trimester of pregnancy.
I called a separate cluster "Sunday Dads" - its representatives are football fans, avid car enthusiasts, purchase components for Sparco cars and from time to time buy Chupa Chups goods.
And now attention, the question: is it worth deleting "Sunday dads" if they do not belong to the originally designated target audience? I often ask this question to my project managers, and the task is rethought. Perhaps we do not really need a specific target audience, but everyone who can become buyers of the product. In our case, these are dads, and grandparents, brothers-sisters, and girlfriends of a woman in labor, ready to take care of the baby. The answer to which audience should be considered the target is for business representatives.
Case "Individual Entrepreneurs"
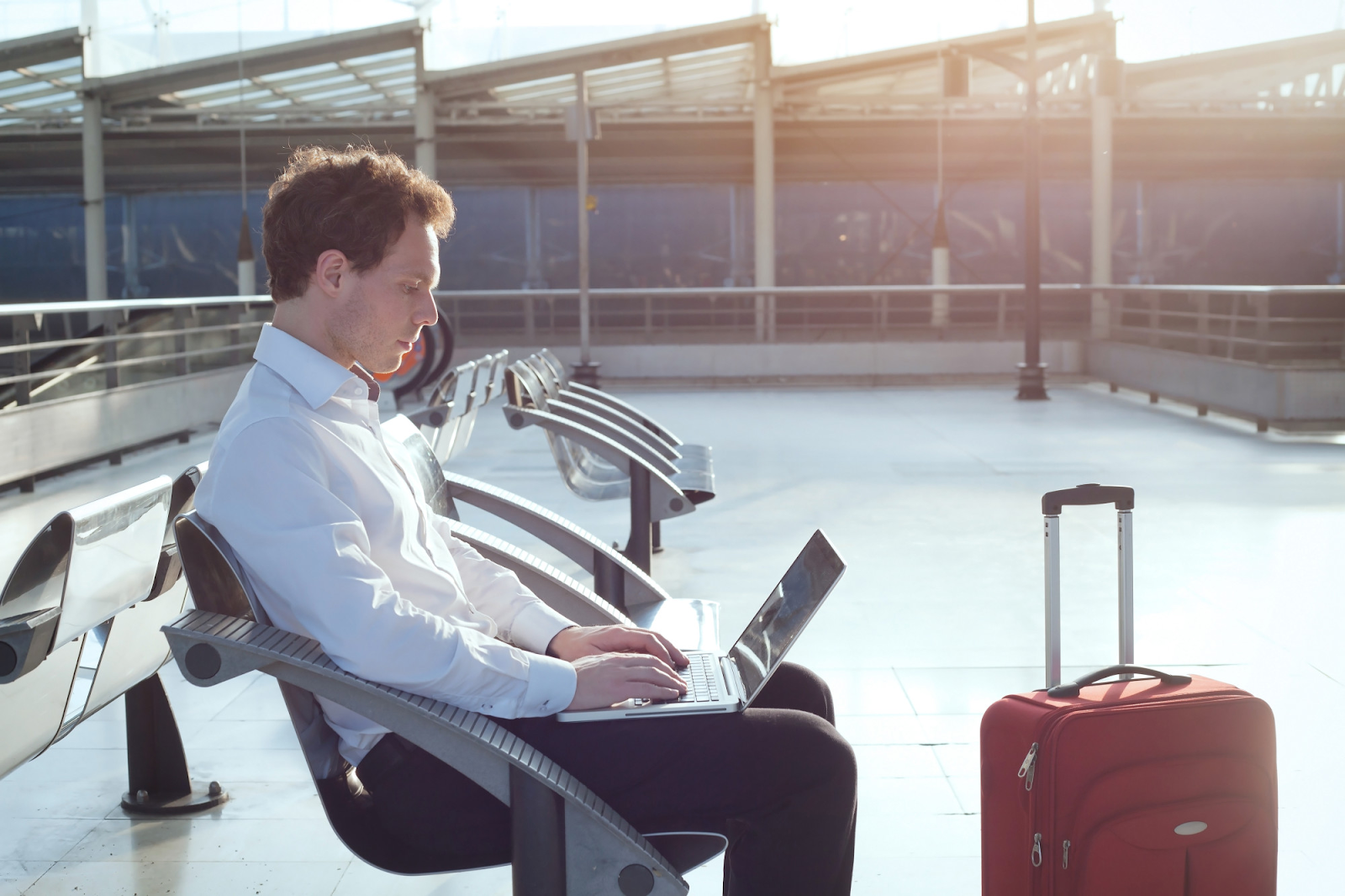
The next case, which I will tell you about, is Audience Research for the target audience “Individual entrepreneurs” who have opened a current account in a well-known bank.
The main differences between these people and the audience of the exchange can be clearly seen in their purchases. The most obvious is the payment of royalties (10-15% of profiles), security services and utility bills for non-residential premises. Among the indirect signs pointing to entrepreneurs is the purchase of an additional piece of baggage during flights (in 15-20% of cases). In the entire volume of checks, a significant part is made up of books on psychology, self-knowledge and self-development, workshops for communicating with subordinates and coaching literature.
With the help of LaL feature importance, we got indirect signs of target audience: air transportation, purchase of a robot vacuum cleaner, coffee machine, Honor smartphone, flower delivery, payment of insurance premiums. This case is one of those wonderful cases where machines give us an easily interpretable result.
Busy people buy home robots. No office can do without coffee machines. Flower delivery and frequent flights can also be linked =).
Case "Car owners"
A well-known auto brand in the “above average” price segment was absolutely convinced that its customers were completely exceptional people and wanted to know their habits and preferences.
This target audience significantly overlaps with the previous case ("Individual Entrepreneurs"). But not all individual entrepreneurs buy this brand of car.
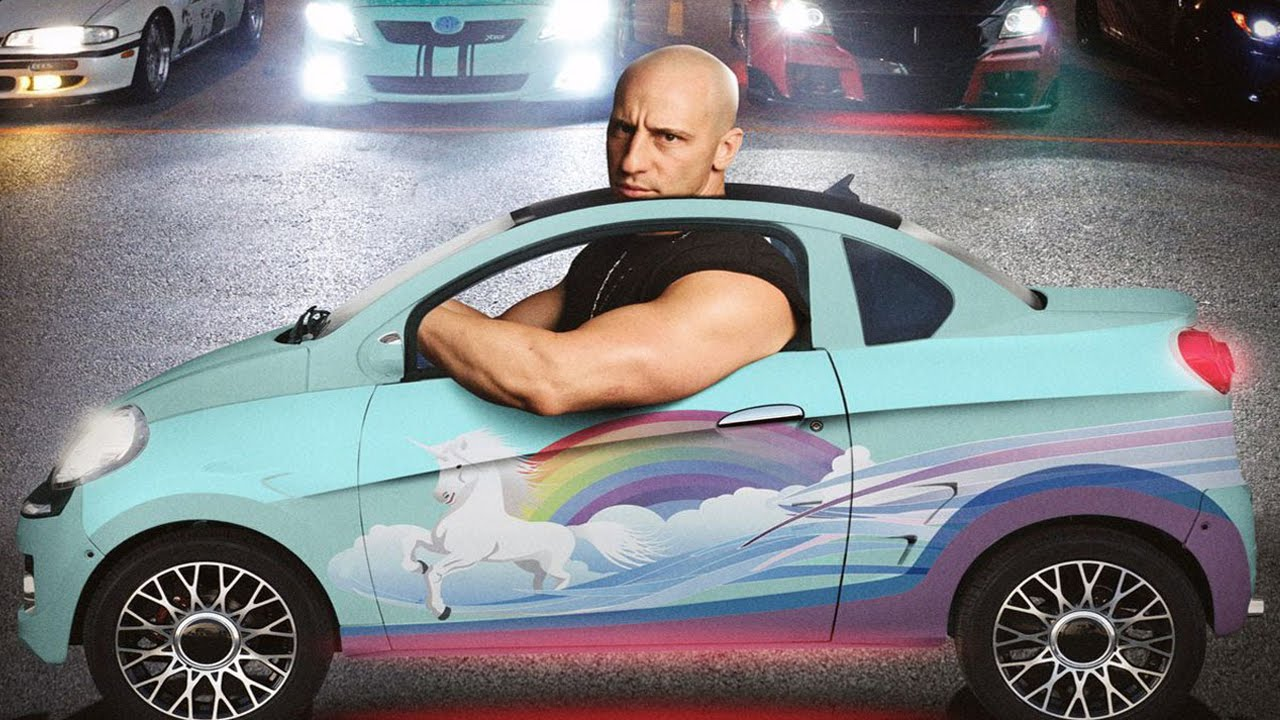
It turned out that the customer's idea of the uniqueness of customers is greatly exaggerated. Yes, the audience does not coincide with the average one, but only in some details, for example, motorists prefer to buy elite tea (300 rubles more expensive) and generally spend more on beautiful and aesthetic than on functional and practical.
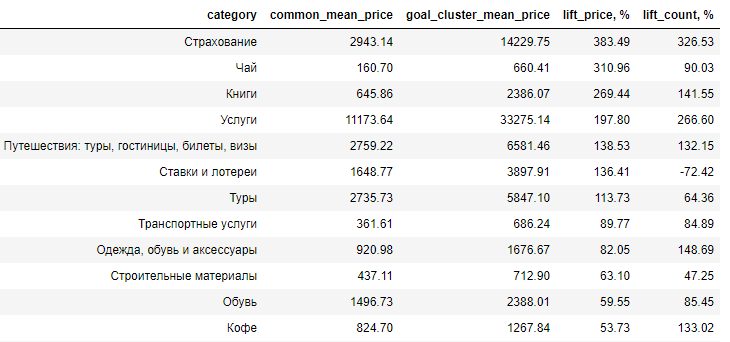
Here the difference between the target audience and the average audience of buyers in terms of lift is presented, that is, by what percentage the average price of a product in the studied audience exceeds the same value in the average audience (lift_price). As you can see, the main spending is on pleasure.
We always test hypotheses fairly and impartially. It is quite expected that sometimes the customer's hypothesis about the exclusivity of his audience is not supported by the data obtained. There is nothing to worry about, it just needs a new hypothesis and new research.

In conclusion, I would like to say that in my work I am guided by the principle “Routine calms”. And I advise you.
With such a variety of data, it is imperative to be very careful and attentive to the little things, since any exception at first glance may later turn out to be the rule and we can get many erroneous results.
So, if I hadn’t seen what my “no baking” model refers to as “baking”, the “leaky” system would have gone into production. So do not neglect the routine: if you spend half an hour checking with your eyes, you can sleep well - the model will not make mistakes.
