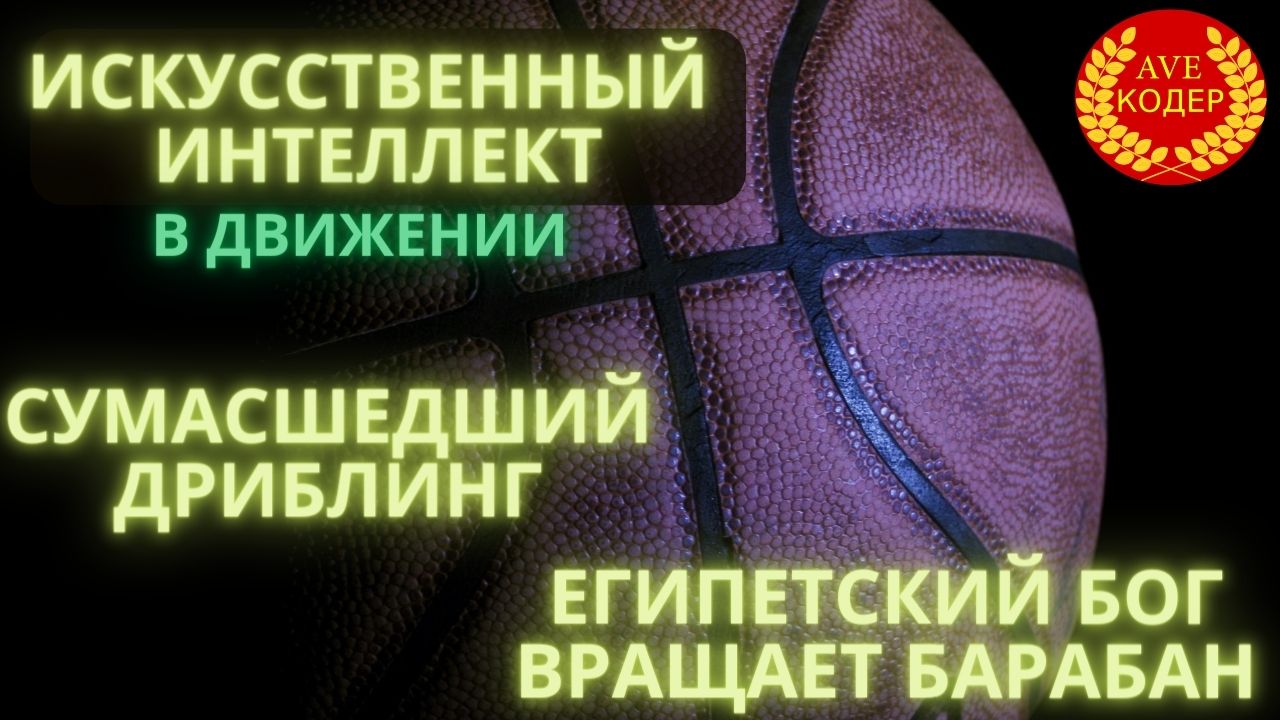
Full video tut: youtu.be/lPfiMHQWP88
Ave, Coder!
Physics in the world of modern computer games is becoming more accurate and juicy from year to year, especially if we are not talking about hypercasuals and classics like Arkanoid, but about hits with an open world and realistic models, in which each joint moves as naturally as possible in order to imitate models from the real world.
And, therefore, when our eye sees something unnatural in the movement of a computer dog, for example, it will immediately send a signal to the brain - something is wrong. Perhaps the gamer will not understand what exactly is wrong, but the brain has subconsciously compared what it saw with the experience from real life, for example, how the dog moves and noticed inaccuracies.
Therefore, developers usually do not code it by hand, but record tons of motion capture in real time and later adapt it for game models.
Artificial intelligence has long been used for these purposes and game studios have been able to achieve real results thanks to it, but today we will talk about a development that can leave competitors far behind - at least in the area for which it was created. But who said that something like this cannot be scaled further?
Basketball. Dribbling. Crazy dynamics. Racks. Handling the ball. Models move quickly, changing direction frequently. It will take a really grandiose solution so that all this dynamic goodness is processed quickly, powerfully and, meanwhile, realistically.
And an additional challenge lies in the fact that the AI is given only three hours of motion capchure training material, which is a drop in the bucket compared to what other neural networks are trained on for similar tasks.
In addition, the neural network must be able to simulate movements that were not presented in the training, but were available for the player-driven model.
It would seem, given the limitations, AI should have been unable to cope with the task, at least partially. There were assumptions that the movements included in these three hours of training, the neural network will adapt without problems, but with the synthesis of new ones it will be more difficult and therefore the models will behave unnaturally at some points, but the result exceeded all expectations.
While controlling a real player, the electronic basketball player did not lose his plasticity in movements, even if the player pressed the control buttons like a madman.
And, by the way, about the variety of model behavior. That is, do the movements look the same for the same situations? Take dribbling, for example - AI is able to add variety and change to the way a model dribbles, combine them together to create new moves of the same type, and still be responsive to control.
Dribbling example:
This is pretty impressive for a neural network that has been trained with just three hours of material, but there is something else that could go beyond expectations.
The player could also throw the ball into the hoop and rebound and the model behaved naturally, despite the fact that the neural network was provided with less than seven minutes of training material.
And, in addition, the model is able to synthesize movements that were not in the training material, but which it considers adequate for certain situations.
As you can see from the video example, one model is trained to move using a training method based on the Phase-Function Neural Network, and the other is taught by AI4Animation.
Comparison of the two models:
When comparing the movements of the two models, the players could notice a clear lack of stiffness in the AI4Animation variant: the smoothness of movements inherent in living organisms and the way the model controls a third-party object - a ball.
When dribbling, the model trained by the Phase-Function Neural Network forces the ball to be, as it were, glued to the player's hand only to make it easier for her to calculate the model's movements, but in this case it did not bring an obvious advantage.
In AI4Animation, the model remained more responsive to player control and therefore was not only more pleasant to look at, but also to control it.
Now let's imagine what this technology will be capable of, not even in five or ten years, but already, say, in a year.
How much will it improve? What other sports games will it find application in? Just ... sports? Only in ... games?
In this case, the creators tested the neural network in a very narrow specialization, namely, the ability to synthesize the natural movements of human models playing basketball, based only on a limited amount of data provided for training, while the models had to remain controllable and adequately respond to control. And of course the quality should not have suffered from this.
Now let's see how this same technology can be applied to other problems.
For example, this drawn “good boy” moves just like dogs move in life, moreover, movements and gait masterfully adapt to commands and conditions.
Example with a "good boy":
And here Anubis decides to lay his mythological ass on various furniture and, as Malysheva would say, does it naturally.
Anubis example:
Or trying to work as a delivery man for black boxes in “What? Where? When?". It remains only to teach him how to spin the drum ...
In any case, we can be sure for the Egyptian god of death - he will have a gorgeous future on television.
You can check it out here: github.com/sebastianstarke/AI4Animation
It was V. Check out the channel "Ave, Coder!"
Ave!