
In the field of education, specialists are currently actively investigating and widely discussing the issue of how to optimally support students during the learning process. The versatility of the problem of effective personalized learning, together with the depth of questions about the work of cognition, determines the current position of educational institutions and the position of educational systems (very deplorable).
How can you provide effective student support?
The first thing that comes to mind and seems more or less obvious to those participating in the discussion (as well as observers) is the learning outcome is most significant if the training program and content are aimed at the specific needs of the learner. Simple, but the problem of teaching "the wrong" is relevant at all times. It is interesting, by the way, in how many places specifically specifically you, who are reading this post now, have already had to forget what they taught in school / previous place, and retrain.
Second, for the most optimal support of the learner's attempts to learn, the appropriate complexity of the content is extremely important .: not too easy, but not too difficult either. So that there is a balance between engagement and fatigue that keeps you motivated to learn. More scientifically, for successful learning, it is important to maintain the cognitive load of the learner within the optimal interval for a particular learner.
How do you achieve this kind of personalization?
The traditional way to optimize cognitive load is to adapt the complexity of the learning content to the individual competencies of the learner.
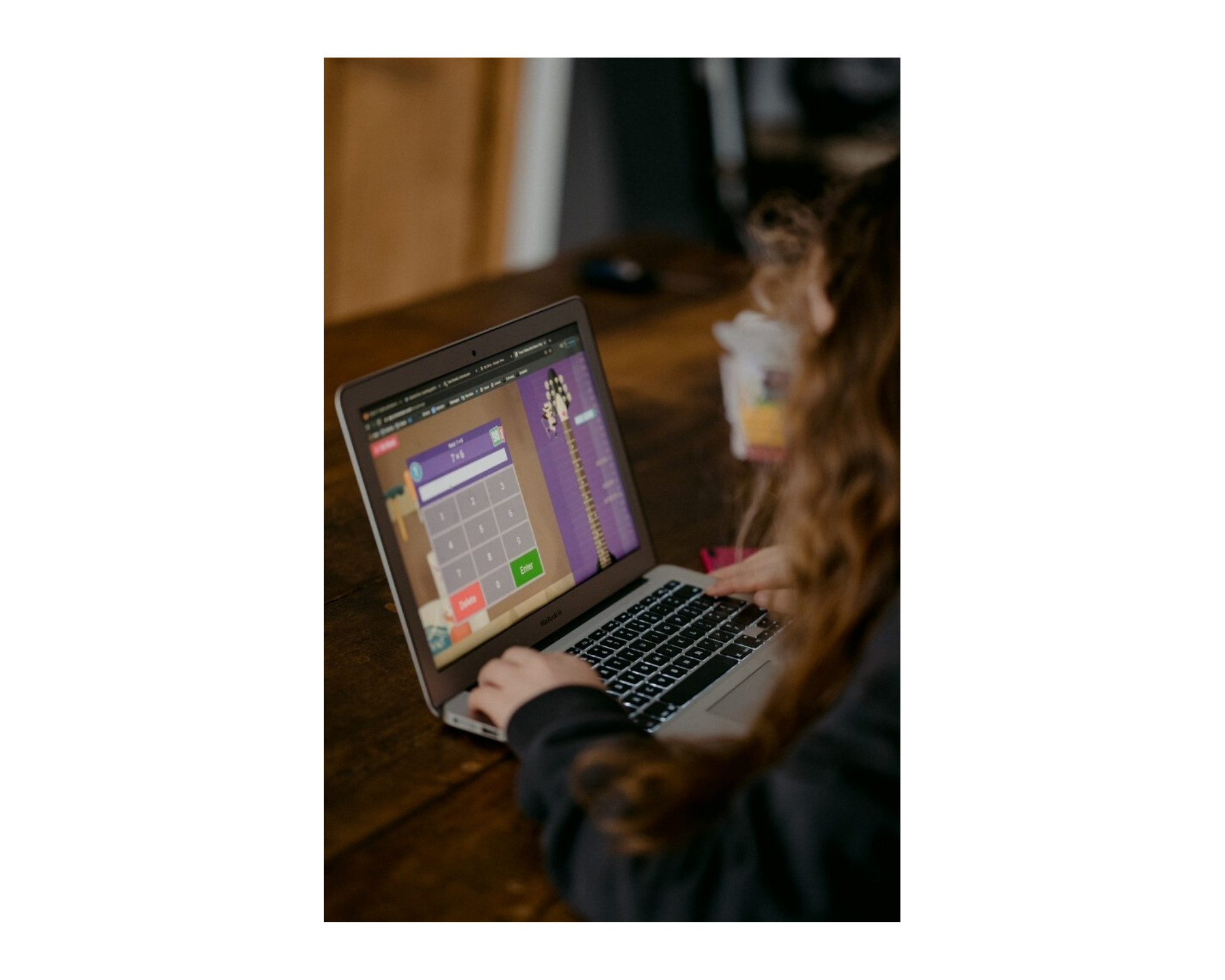
Figure: 1 . A computer assistant program that gamifies learning and adapts educational content for the student.
At the same time, for the implementation of adaptability and the specialized implementation of such training support, the use of digital assistants is best suited: computers, tablets, smartphones. Computer information environments can be rather easily extended by algorithms that change the complexity of the presented material depending on the student's behavioral reactions and responses. This “adaptability” is able to offer an uncomplicated personalization of the learning environment to the user's needs, which is the key to more effective learning.
How does the adaptability of educational services work now?
Currently, computer-assisted learning environments are tailored to a specific user based on behavioral responses and past performance - for example, based on the number of correct answers, the ratio between correct and incorrect answers, progress track, time spent on a task, or other similar metrics. ...
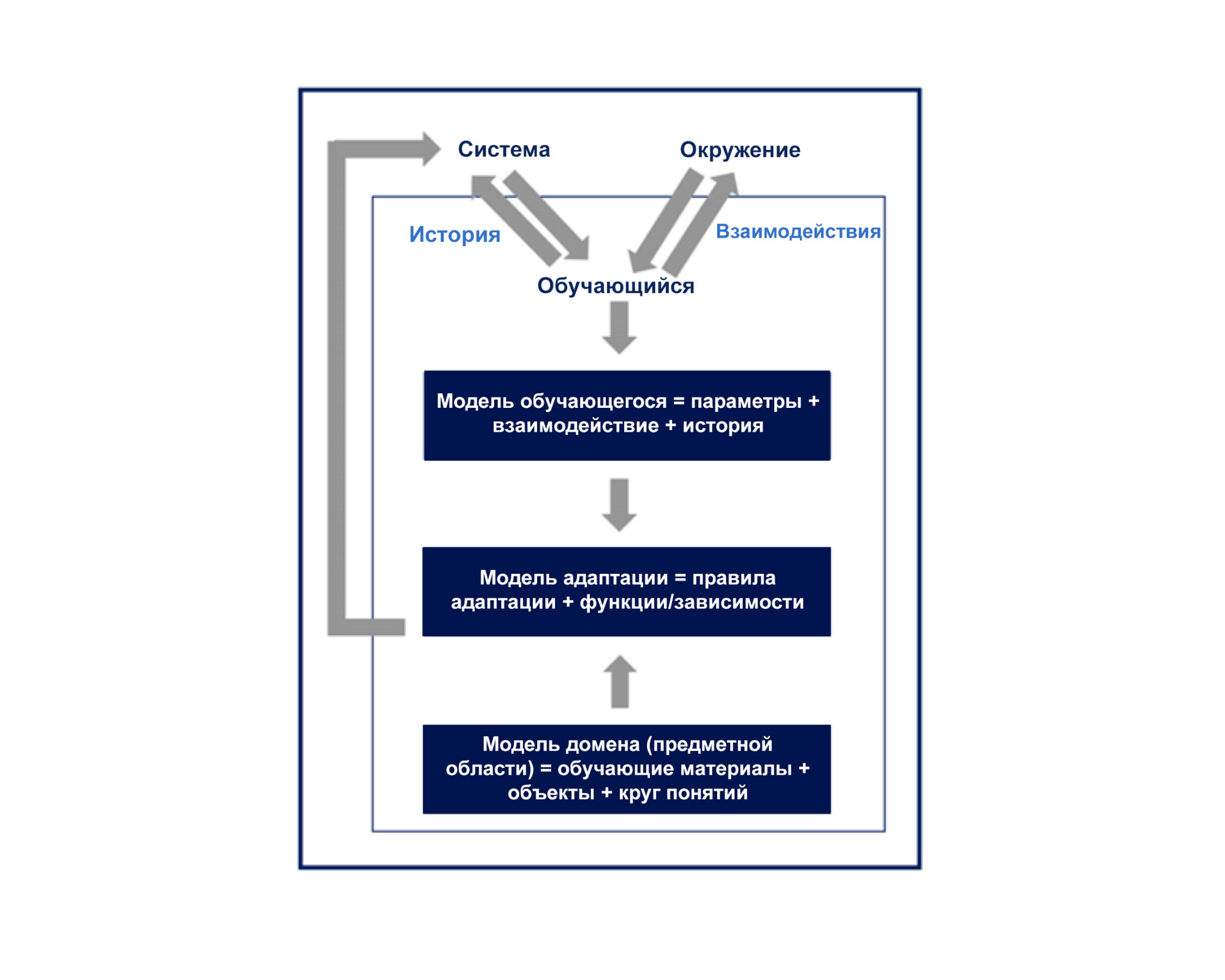
Figure: 2 . The fundamental structure of adaptive learning systems based on modeling student behavior, ideas about the field of knowledge and an adaptive model that connects them. Read more about this in the source .
If you think about it, it becomes clear that such “behavioral” parameters at the heart of personalization are mostly “indirect” measurements, therefore the accuracy provided by this approach is often extremely low.
So, for example, a large number of mistakes in passing the test, made by a student in a row, can easily be caused not by the test itself, its complexity, but by nonspecific processes such as loss of concentration, involvement, psychophysiological state or emotional reactions while completing tasks.
Can new technology come to the rescue?
Spoiler
. , , .
A number of neurocognitive processes are involved in learning, such as, for example, memory, perception, attention, etc., which underlie cognition and, in fact, are responsible for the result of educational activity. Therefore, direct access to information about these processes (for example, their monitoring), as well as the ability to manage them, can provide new tools in personalized learning and bring educational practices to a fundamentally new level.
Information about cognitive processes is hidden, of course, in the activity of the brain. Quite deeply and covertly, however, it can still be found and extracted from the recorded neurophysiological indicators.
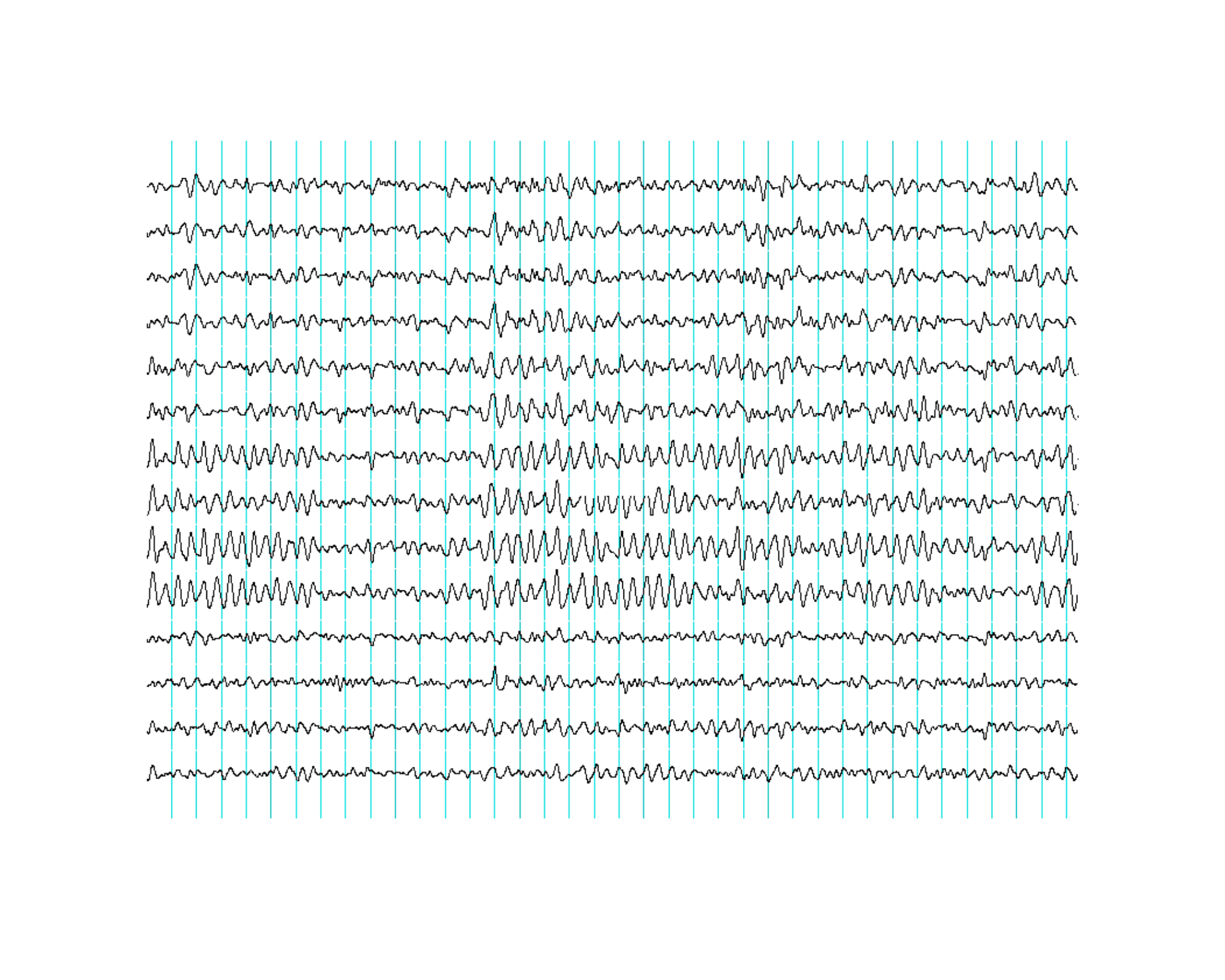
Figure: 3. , . - .
?
With the emergence and development of brain-computer interfaces - devices that directly connect the human brain and a computer (and therefore the brain and any device of the Internet of Things), allowing the transmission of brain signals into control signals of physical or information devices - as well as the development of methods analysis of complex data, a new technology is emerging, which makes it possible to extract and use information about the ongoing individual neurocognitive processes in learning and building learning environments.
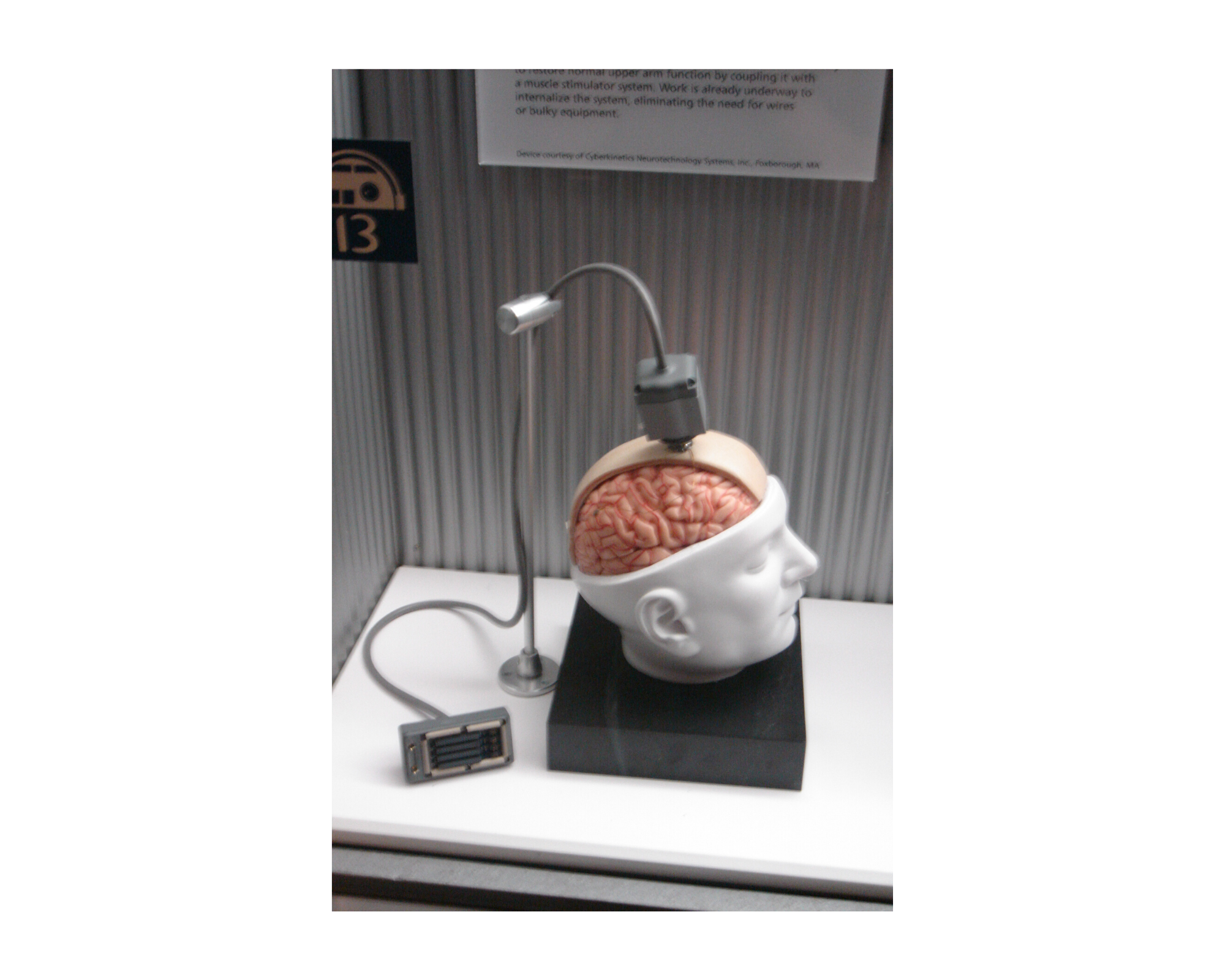
Figure: 4 . A model of the "brain - computer" interface, which few people would agree to use (due to its invasiveness: the electrode is inserted directly into the brain tissue). Read more in the source .
Brain activity can be accessed in a variety of ways. Among others, electroencephalography (EEG) is the most widely used method for measuring brain activity. The signals are read using electrodes located in separate different parts on the surface of the head (non-invasively, not as in the picture above), amplified and transmitted to a computer. The figure below shows an example of a system that records weak electrical signals coming from the brain from the surface of the head.
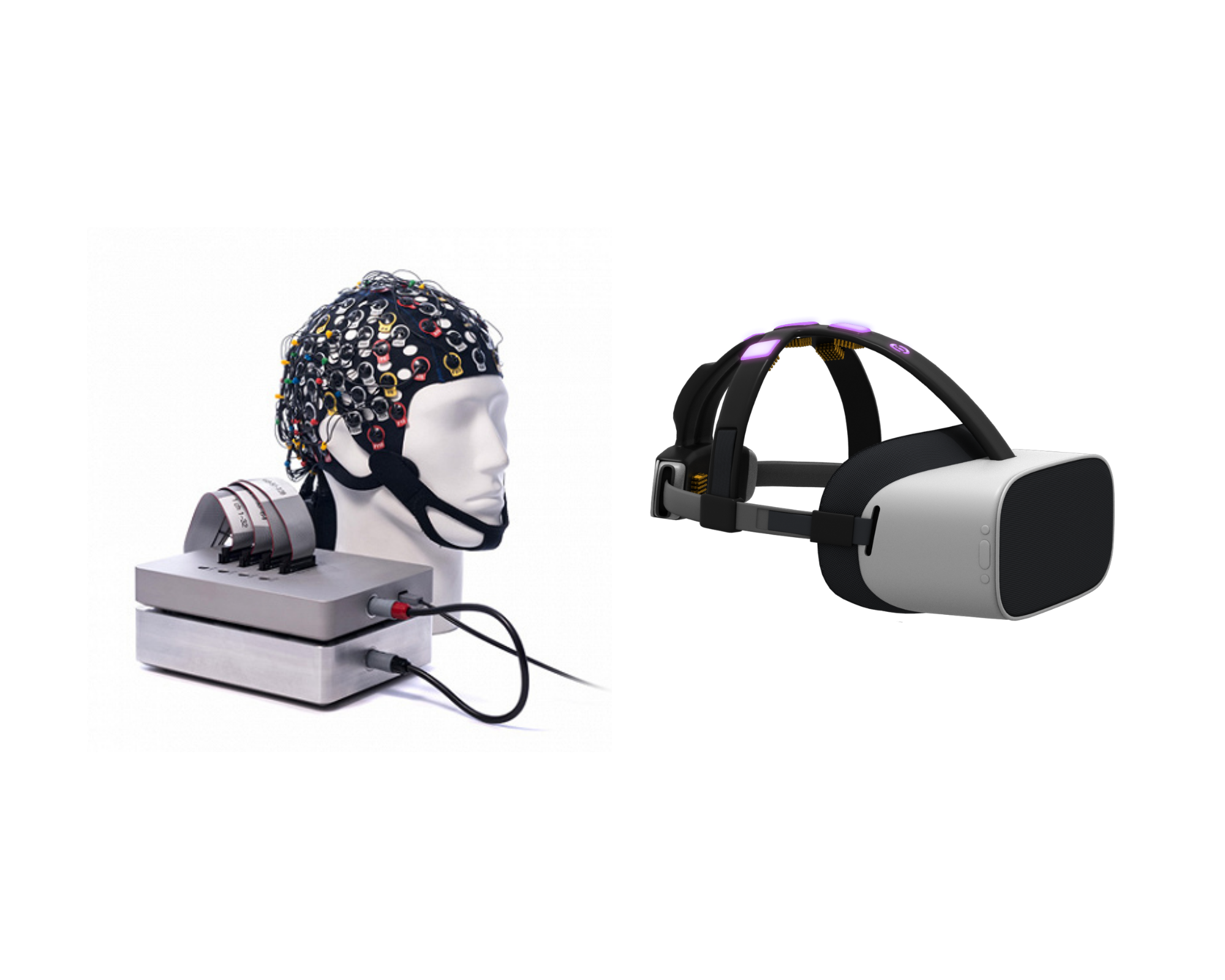
Figure: 5 . Laboratory device for reading bioelectrical signals of the brain (left) and its non-laboratory "analogue", developed by a young Russian companycombined with a VR helmet (right). The electrodes are highlighted in pink in the picture.
Many of you have probably seen these extravagant-looking hats with tons of wires coming from different places and connected to the box with the encephalograph. However, not everyone knows that at present, the direction associated with the transition from such devices to user-friendly consumer averiday-interfaces is actively developing, and many foreign and Russian companies are developing devices convenient for use by a wide audience for measuring brain activity. ...
Let's go back to brain signals. How strong are they? What and how can you do with them?
Raw sensor signals are noisy, complex, non-stationary, and large. Therefore, they go through the process of primary processing - preprocessing, including filtering, after which they become suitable for the extraction of individual components, which can be further used as control signals. Various machine learning methods are used to process sensor readings. Such methods usually require a certain amount of data to train the algorithms: they have been preprocessed, subjected to manual markup to understand where what is written, and the possibility of assigning them to a particular class by specialized labels.
Based on this data and the corresponding labels, the algorithms learn to find patterns in the new information being read and classify them.as well as build predictive models to predict which class new data points will belong to.
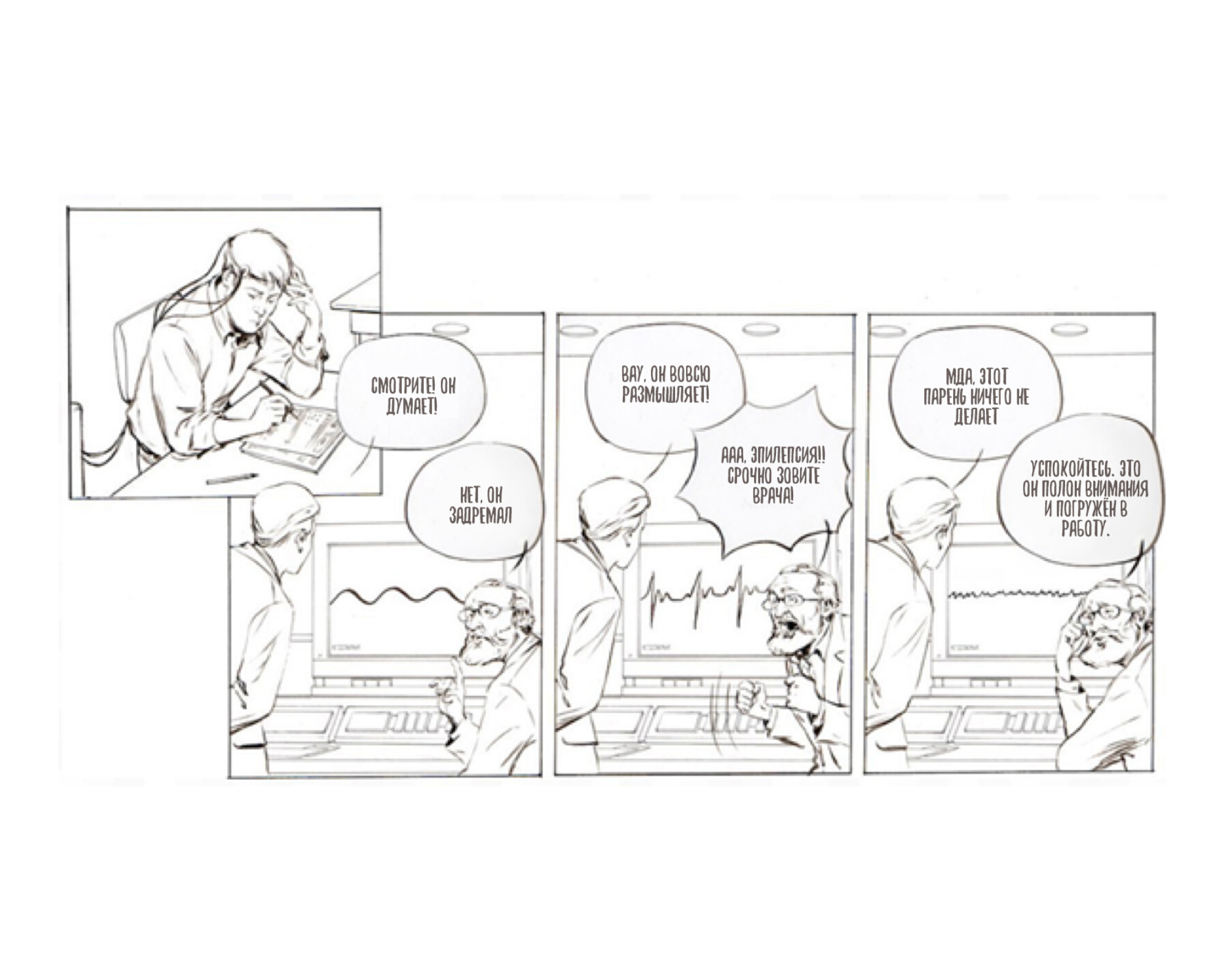
Figure: 6 . Signal classification without the use of modern computer methods.
Controlling and highlighting patterns is clear. How to use it in education?
Traditional BCI (Brain-computer interfaces) make it possible to communicate with a computer or control it by using the brain activity, divided by algorithms into several classes. Later modifications of neurointerfaces allow just as effectively to extract some information about the user himself (of course, with his consent, and - attention! - this is not mind reading), assess his / her mental states (e.g., cognitive load, emotional state, level of attention / vigilance).
Thus, with the help of the BCI and the computer, it becomes possible to carry out monitoring and feedback with the presentation of information about the functioning of the brain that is useful to the user. In addition, since neurointerfaces allow assessing cognitive processes, including in real time, the use of BCIs is able to implement more cunning and accurate (in comparison with the indirect methods that underlie traditional adaptive learning systems) implicit tracking of the student's state and thereby contribute to better adaptation educational content to improve the success of the educational process.
Many studies aimed at measuring cognitive load, done by different groups of scientists, have repeatedly demonstrated how the amount of cognitive load can be measured using EEG.

Figure: 7. Experiment of the BrainCo company in the Chinese school. A device that tracks student concentration at school. China is actively interested in learning effectiveness.
This topic deserves a separate review. Here I will only say that the main parameters for EEG feedback are usually changes in amplitudes associated with potential events or specific oscillations (rhythms), recorded - for example, as a change in signal power in certain frequency ranges when solving arithmetic problems - with increased attention (concentration) or on the contrary, relaxation.
Similar workload effects, extracted and classified in the EEG, can be detected by the BCI and used to adapt the learning environment.
So this is technology? Let's use?
- Well, yes, yes. Let's try ...
Current advances in artificial intelligence, reinforcement learning using neural signals, coupled with brain-computer interface technologies, are able to provide a better understanding of the functioning of the learner's brain, as well as use individual metrics in constructing adaptive immersion computer environments for effective personalized learning.
The use of neuro-computer interfaces, which are actively developing now, has the potential to take personalized learning to a new level, increasing the quality, efficiency of the educational process and the enjoyment of it, significantly improving traditional approaches of adaptive learning.
Of course, not every neural interface actually allows you to do what is written above, however, devices that really work are already actively appearing on the market.
Tell us in the comments if you would like to try such devices on yourself.