
One of the world's first technologies for storing and exchanging data.
In the 19th century, doctors could prescribe mercury for mood swings and arsenic for asthma. It may not have occurred to them to wash their hands before surgery. Of course, they didn't try to kill anyone - they just didn't know there were more suitable methods.
These early physicians had valuable data scrawled on their notebooks, but each saw only one piece of a large puzzle. Without modern tools for the exchange and analysis of information (as well as science for making sense of this data), nothing could prevent superstition from influencing what can be seen through the "keyhole" of observed facts.
Humans have come a long way with technology since then, but today's boom in machine learning and artificial intelligence is not out of touch with the past. All this is a continuation of the basic human instinct - understanding the world around us. This instinct is needed so that we can make smarter decisions. And we now have significantly better technology than ever before.
One way to describe this pattern that has been going on through the ages is to think of it as a revolution in datasets, not units of data. The difference is not trivial. Massives of data have helped shape the modern world. Consider the Sumerian scribes (modern-day Iraq) who pressed their styluses to clay plates over 5,000 years ago. When they did, they not only invented the first writing system, but the first technology for storing and exchanging data.
If you're inspired by the promise that AI can surpass human capabilities, consider stationery to give us superhuman memories. While it is easy to take the recording of information for granted today, the ability to securely store datasets represents a groundbreaking first step towards higher intelligence.
Unfortunately, extracting information from clay slabs and their pre-electronic counterparts is a pain. You cannot click your finger on a book to count the number of words in it. Instead, you have to load each word into your brain to process it. Issues like these made early data analysis laborious, so early attempts got stuck very early on. While the kingdom could analyze tax revenues, only a fearless soul could try to reason as effectively in a field like medicine, where a thousand-year tradition encouraged improvisation.

Fortunately, the human race has produced incredible pioneers. For example, John Snow's map of deaths, compiled during the cholera outbreak in London in 1858, inspired doctors to reconsider the superstition that the disease was caused by miasma (toxic air), and to pay attention to drinking water.
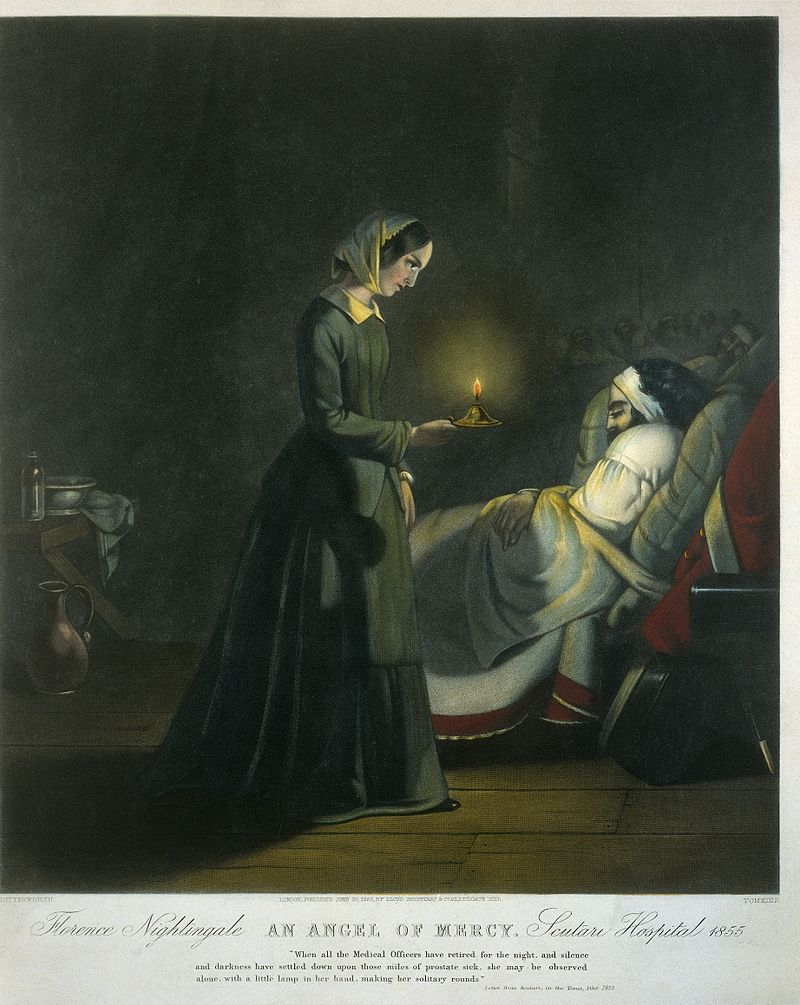
If you know The Lady with the Lamp, Florence Nightingale, for her heroic compassion as a nurse, you might be surprised to learn that she was also a pioneer in analytics. Her inventive infographic during the Crimean War saved many lives because it identified hygiene problems as the leading cause of hospital deaths, and it was this infographic that inspired the government to pay attention to sanitation.

The era of uniform datasets emerged as the value of information began to assert itself in more and more areas, leading to the advent of computers. And this is not about the electronic buddy you are used to today. The "computer" (calculator) originated as a human profession, when special employees performed calculations and processed data manually in order to assess their significance.

These people were all computers! Photo taken in the 1950s by the staff of the Supersonic Pressure Tunnel .
The beauty of data is that it allows you to shape judgment out of something more meaningful than thin air. By looking at the data, you're inspired to ask new questions, following in the footsteps of Florence Nightingale and Jon Snow. This is the discipline of analytics: to inspire models and hypotheses through research.
From datasets to data partitioning
In the early twentieth century, the desire to make better decisions in the face of uncertainty led to the birth of a parallel profession: statistics. Statisticians help to check whether it is reasonable to behave in accordance with the phenomenon that the analyst discovered in the current dataset (and beyond).
A famous example is Ronald A. Fisher, who developed the world's first textbook on statistics. Fisher describes running a hypothesis test in response to his friend's claim that he could determine whether milk was added to tea before or after water. Hoping to prove that this was not true, based on the data, he had to conclude that his friend really could have done it.
Analytics and statistics have a big Achilles' heel: If you use the same piece of data to generate a hypothesis and test it, then you are cheating. The rigor of statistics requires you to declare your intentions before taking the appropriate action. Analytics is more of an extended retrospective game. Analytics and statistics were frustratingly incompatible until the next major revolution (data sharing) changed everything.
Sharing data is a simple idea, but it is one of the most important ideas for scientists like me. If you only have one dataset, you have to choose between analytics (unsubstantiated inspiration) and statistics (strong inferences). Want a trick? Divide your dataset in two and you have both the wolves fed and the sheep safe!
The era of two datasets removes the tension between analytics and statistics and introduces coordinated work between two different types of data scientists. Analysts use one set of data to help you formulate questions, and statisticians use a different set of data to provide strong answers.
This luxury places strict demands on the amount of data. It is easier to talk about separation than to actually implement it. You know what this is about if you've tried to collect enough information for at least one decent dataset. The era of double datasets is a new development that goes hand in hand with better data processing equipment, lower storage costs, and the ability to share collected information over the Internet.
In fact, the technological innovations that led to the era of double datasets quickly ushered in the next phase - the era of automatic three-datasets.
There is a more familiar term for this: machine learning.
Using a dataset destroys its purity as a source of statistical rigor. You only have one chance, so how do you know which analytical insight is most worth testing? If you had a third dataset, you could use it to take a test drive of your idea. This process is called validation, and it is at the heart of what makes machine learning work.
Once you are free to test everything and see solid ideas, you can trust anyone to find a solution: experienced analysts, interns, tea leaves for fortune telling, and even algorithms that work out of context about your business problem. The solution that performs best in the validation process will become a candidate for the appropriate statistical test. You've just empowered yourself with the ability to automate inspiration!
Automated inspiration
This is why machine learning is revolutionizing datasets, not just data. It's all about the luxury of having enough data for a three-way partition.
How does AI fit into this picture? Machine learning with multilayer neural networks is technically called deep learning, but it has received another nickname that has stuck in speech: AI. While AI once had a different meaning, today it is most likely used synonymously with deep learning.
Deep neural networks have created a buzz by beating traditional machine learning algorithms on a multitude of complex problems. However, they require a lot more data to train them, and the requirements for data processing capabilities are beyond the capabilities of a conventional laptop. That is why the emergence of modern AI is associated with cloud technologies. Cloud technology allows you to rent someone else's data center instead of assembling hardware yourself, so you can try out modern AI technologies before you start investing in them.
With this piece of the puzzle, we have a complete set of professions: machine learning and AI experts, analysts and statisticians. The general term that describes each of them is an expert in Data Science, the science that makes data useful.
Data Science is the product of our era of triple data sets. Many industries in today's industry regularly generate more than enough data. So is a four dataset approach possible?
What's the next step if the model you just trained shows low validation values? If you behave like most people, then you will immediately demand to find out the reason! Unfortunately, there is no dataset that can answer your question. You might be tempted to dig into your validation dataset, but alas, debugging will break its ability to validate your models effectively.
By analyzing your validation dataset, you are essentially turning three datasets back into two. Instead of doing something useful, you involuntarily returned to the past!
The solution lies outside the three datasets you already use. To arrive at smarter learning iterations and hyperparametric tuning, you'll want to move closer to best practices: the era of four datasets.
Assuming that three datasets provide you with inspiration, learning iterations, and rigorous testing, the fourth will accelerate your AI development cycle with advanced analytics that provide insight into which approaches can be tried at each iteration. By using four-way data sharing, you can take advantage of the abundance of data! Welcome to the future.

Find out the details of how to get a high-profile profession from scratch or Level Up in skills and salary by taking SkillFactory's paid online courses:
- Machine Learning (12 )
- Data Science (12 )
- (9 )
- «Python -» (9 )
- Data Sciene 2020
- Data Science . Business Science
- Data Scientist
- Data Scientist -
- 450
- Data Science : «data»
- Data Sciene : Decision Intelligence